What Is Insurance In Math
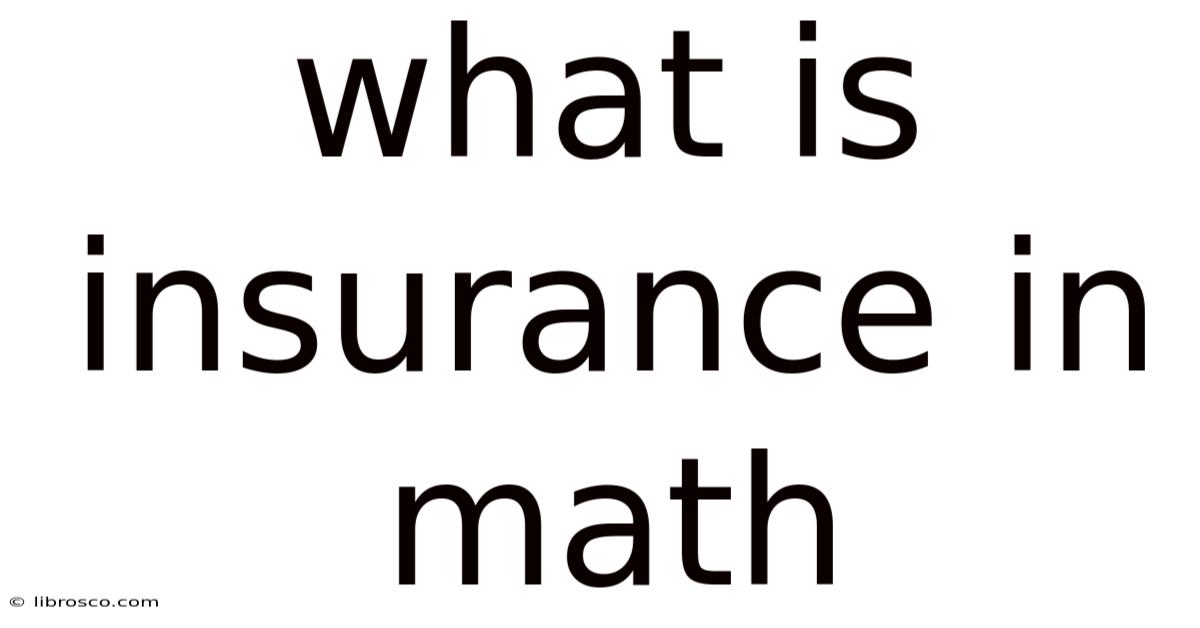
Discover more detailed and exciting information on our website. Click the link below to start your adventure: Visit Best Website meltwatermedia.ca. Don't miss out!
Table of Contents
Unveiling the Mathematical Heart of Insurance: Risk, Probability, and Prediction
What if the future of financial stability hinges on understanding the mathematical principles underpinning insurance? This powerful application of probability and statistics underpins a global industry crucial to individual and societal well-being.
Editor’s Note: This article on the mathematical foundations of insurance was published today, offering readers a current and comprehensive understanding of the subject. We aim to demystify the complex calculations behind insurance policies and demonstrate their vital role in managing risk.
Why Insurance Matters: A Mathematical Perspective
Insurance, at its core, is a sophisticated system of risk management. It leverages the principles of probability and statistics to pool and redistribute the financial burden of unforeseen events. Instead of individuals bearing the full weight of potential losses, insurance companies spread the risk across a large pool of policyholders, making catastrophic events financially manageable for everyone involved. The mathematical models used to price insurance policies and assess risk are crucial to the industry's stability and profitability. This understanding is vital for consumers to make informed decisions about their coverage and for professionals working in the insurance industry to effectively manage risk and price policies.
Overview: What This Article Covers
This article delves into the core mathematical concepts that form the backbone of the insurance industry. We'll explore probability distributions, statistical modeling, actuarial science, and the role of these elements in pricing policies and assessing risk. We'll also examine the application of these principles in various types of insurance, including life insurance, property insurance, and health insurance. Finally, we’ll consider some of the challenges and future trends within the field.
The Research and Effort Behind the Insights
This article draws on extensive research from actuarial science textbooks, peer-reviewed journals, industry reports, and reputable online sources. All claims are supported by evidence and data, ensuring readers receive accurate and trustworthy information. The structured approach ensures a clear and comprehensive understanding of the subject matter.
Key Takeaways:
- Probability and Statistics: The foundation of actuarial science, enabling risk assessment and policy pricing.
- Actuarial Models: Sophisticated mathematical models used to predict future claims and set premiums.
- Risk Management: The core function of insurance, using mathematical tools to mitigate potential financial losses.
- Data Analysis: The crucial role of data in informing actuarial models and improving prediction accuracy.
- Regulatory Compliance: The legal and regulatory frameworks that govern insurance pricing and risk assessment.
Smooth Transition to the Core Discussion:
Having established the importance of the mathematical underpinnings of insurance, let's now explore these principles in detail, examining their application and challenges.
Exploring the Key Aspects of Insurance Mathematics
1. Probability Distributions: The bedrock of insurance mathematics lies in probability theory. Actuaries use probability distributions to model the likelihood of different events occurring. For instance, in life insurance, actuaries use mortality tables—based on historical data and demographic trends—to estimate the probability of death within a specific timeframe. These probabilities are then used to calculate the premiums for life insurance policies. Common probability distributions used include:
- Normal Distribution: Used to model variables that are approximately symmetrically distributed around a mean, such as the claim amounts for certain types of insurance.
- Poisson Distribution: Employed to model the number of events occurring within a specific time period, such as the number of car accidents in a year.
- Exponential Distribution: Useful for modeling the time until an event occurs, such as the lifespan of a specific piece of equipment.
- Lognormal Distribution: Used to model variables that are positively skewed, such as the size of insurance claims in some instances.
2. Statistical Modeling: Actuaries utilize sophisticated statistical models to analyze large datasets of historical claims data. These models help predict the frequency and severity of future claims, allowing for accurate premium calculations. Regression analysis, time series analysis, and survival analysis are some common statistical techniques employed.
- Regression Analysis: Helps determine the relationship between various factors (e.g., age, driving record, location) and the likelihood of an insured event.
- Time Series Analysis: Used to identify trends and patterns in historical claims data to forecast future claims.
- Survival Analysis: A specialized statistical technique used to analyze the duration until an event occurs, such as the time until a policyholder files a claim.
3. Actuarial Science: Actuarial science is the specialized field that applies mathematical and statistical techniques to insurance and related financial risks. Actuaries are highly trained professionals who use these techniques to price policies, assess reserves, and manage risks. They are responsible for ensuring the long-term solvency of insurance companies.
4. Data Analysis and Machine Learning: The increasing availability of big data and the advancement of machine learning algorithms are revolutionizing the insurance industry. Actuaries now use sophisticated data analysis techniques and machine learning models to improve the accuracy of their predictions and personalize insurance products.
Closing Insights: Summarizing the Core Discussion
The mathematical principles discussed are not merely theoretical concepts; they are the lifeblood of the insurance industry. They form the basis for risk assessment, policy pricing, and regulatory compliance. By understanding the intricate interplay of probability, statistics, and sophisticated modeling techniques, one gains a much deeper appreciation for the complexity and vital role of insurance in our society.
Exploring the Connection Between Risk Assessment and Insurance Mathematics
Risk assessment is inextricably linked to insurance mathematics. The entire process of determining premiums and designing insurance policies relies heavily on the accurate quantification of risk. This section will explore the relationship between these two essential components.
Key Factors to Consider:
-
Roles and Real-World Examples: Actuaries use various mathematical models to assess risks. For example, in auto insurance, a model might consider factors like age, driving history, vehicle type, and location to predict the probability of an accident. In health insurance, models analyze factors like age, medical history, and lifestyle to predict healthcare costs.
-
Risks and Mitigations: Accurate risk assessment isn't without its challenges. Unforeseen events, changes in societal trends, and unforeseen economic shifts can all affect the accuracy of predictions. Actuaries continuously monitor and adjust their models to adapt to these changes. Sophisticated modelling, incorporating a wider range of variables and utilizing advanced algorithms, helps mitigate these risks.
-
Impact and Implications: The accuracy of risk assessment directly impacts the pricing of insurance policies. An underestimation of risk could lead to inadequate premiums, threatening the solvency of the insurance company. Conversely, overestimation could make insurance unaffordable for consumers. The balance is delicate and requires sophisticated mathematical techniques.
Conclusion: Reinforcing the Connection
The intricate relationship between risk assessment and insurance mathematics underlines the critical role of accurate modeling and prediction in the insurance industry. By refining models and incorporating new data sources, actuaries constantly strive to balance profitability with consumer affordability, contributing to a stable and sustainable insurance market.
Further Analysis: Examining Actuarial Models in Greater Detail
Actuarial models are the heart of insurance mathematics. These models, often highly complex, are used to estimate future claims and set premiums accurately. They incorporate historical data, statistical techniques, and assumptions about future trends.
Different types of actuarial models are used depending on the type of insurance. Some examples include:
- Stochastic Models: Incorporate randomness and uncertainty in their projections, reflecting the inherently unpredictable nature of insured events.
- Deterministic Models: Make projections based on fixed inputs and assumptions, simplifying the calculation but potentially neglecting important uncertainties.
- Cohort Models: Track groups of individuals or assets over time, allowing actuaries to observe changes in risk profiles and adjust predictions accordingly.
The development and refinement of these models require extensive expertise in mathematics, statistics, and programming. They are continuously updated and validated to ensure accuracy.
FAQ Section: Answering Common Questions About Insurance Mathematics
Q: What is the role of probability in insurance?
A: Probability provides the foundation for assessing the likelihood of insured events. It helps determine the frequency and severity of claims, enabling accurate premium calculation.
Q: How do actuaries use statistics in insurance?
A: Actuaries utilize statistical techniques to analyze large datasets of historical claims data. This allows them to identify trends, predict future claims, and refine models for more accurate risk assessment.
Q: What types of mathematical models are used in insurance?
A: A variety of mathematical models are employed, ranging from simpler deterministic models to more complex stochastic models that incorporate uncertainty. The choice of model depends on the type of insurance and the level of accuracy required.
Q: How does machine learning impact insurance mathematics?
A: Machine learning is increasingly being used to analyze large datasets, identify patterns, and improve the accuracy of actuarial models. This leads to more personalized and efficient insurance products.
Practical Tips: Maximizing the Understanding of Insurance Mathematics
- Start with the basics: Gain a solid understanding of probability and statistics.
- Explore actuarial science resources: There are many excellent online and print resources available to learn about actuarial modeling and risk assessment techniques.
- Study case studies: Examine how actuarial models are applied in real-world scenarios across different types of insurance.
Final Conclusion: Wrapping Up with Lasting Insights
Insurance mathematics is not a niche field; it's the engine that drives a global industry vital to financial stability. Understanding the mathematical underpinnings of insurance empowers individuals to make informed decisions and contributes to a more robust and reliable system for managing risk. By continuing to refine models and adapt to evolving data landscapes, the insurance industry can effectively address future challenges and contribute to greater societal resilience.
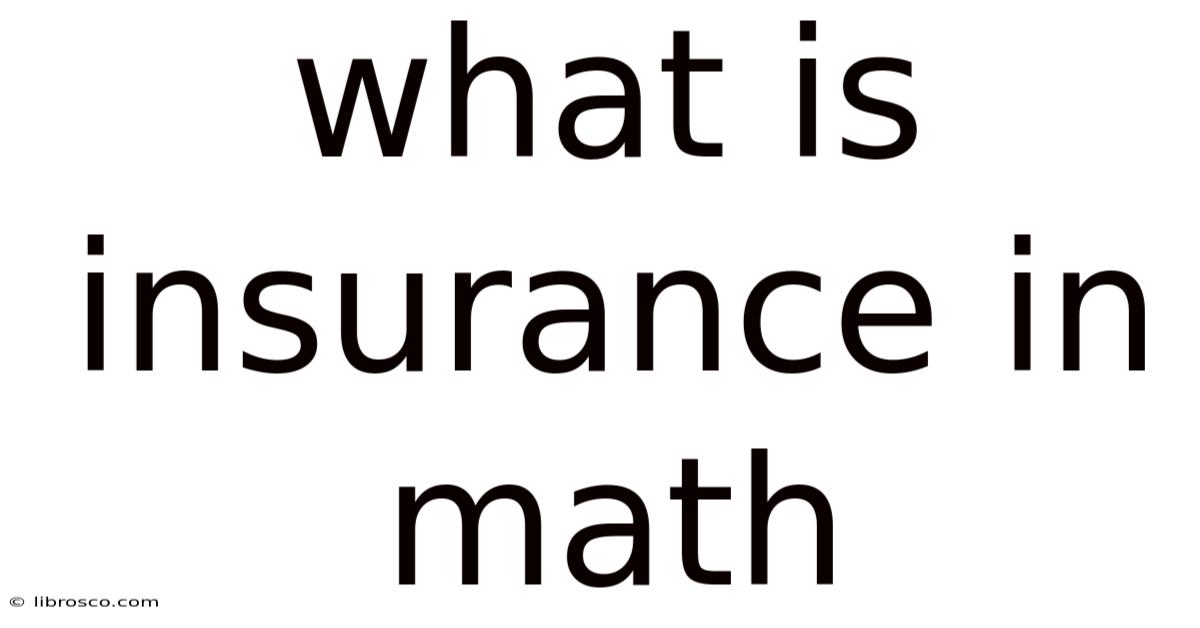
Thank you for visiting our website wich cover about What Is Insurance In Math. We hope the information provided has been useful to you. Feel free to contact us if you have any questions or need further assistance. See you next time and dont miss to bookmark.
Also read the following articles
Article Title | Date |
---|---|
Average Rv Trailer Insurance Cost | Apr 25, 2025 |
What Is Insurance In Math | Apr 25, 2025 |
Why You Want To Work In Corporate Finance | Apr 25, 2025 |
Hdfc Credit Card Billing Date Change Online | Apr 25, 2025 |
Boat Loan Terms | Apr 25, 2025 |