Definition Z Test
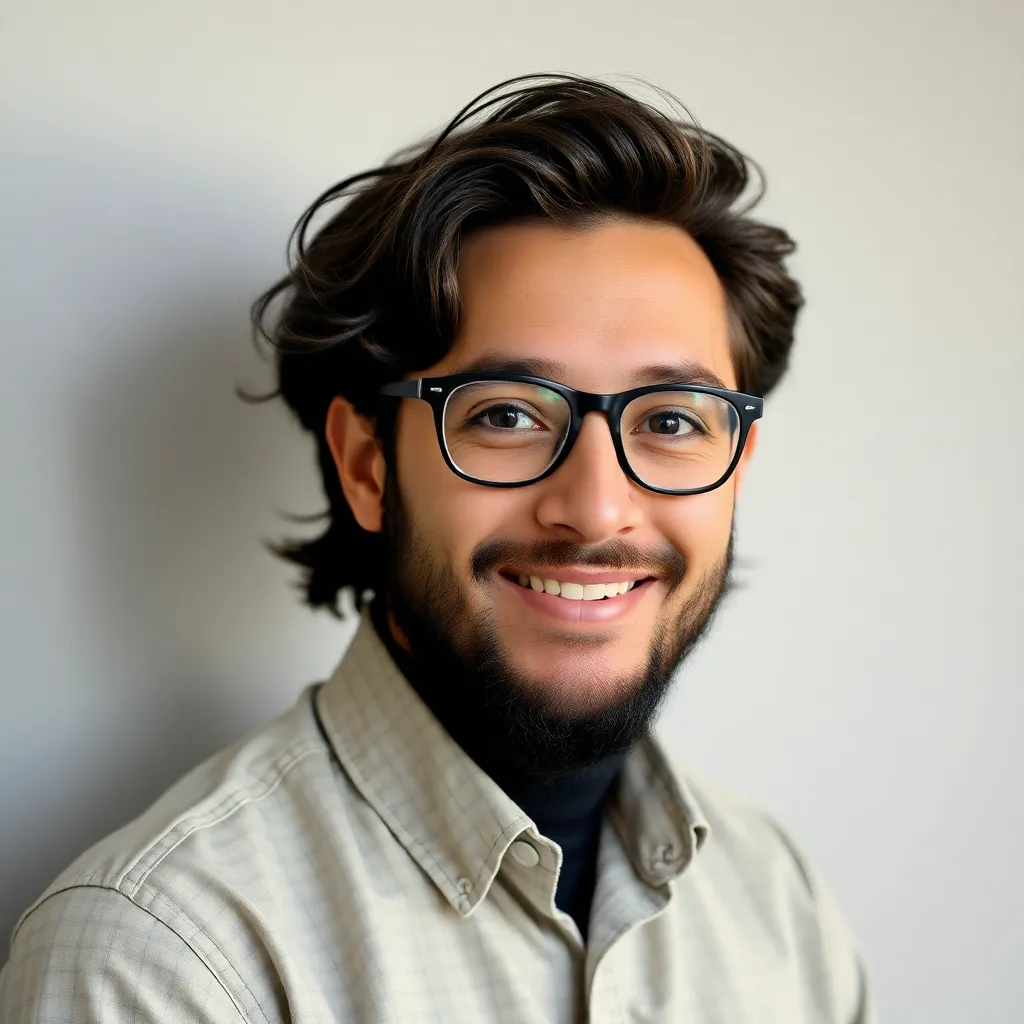
adminse
Apr 02, 2025 · 10 min read

Table of Contents
Decoding the Z-Test: A Comprehensive Guide
What if the power of statistical inference rested on your ability to understand the z-test? This fundamental statistical tool unlocks the ability to make informed decisions based on sample data, offering crucial insights across numerous fields.
Editor’s Note: This article on the z-test provides a comprehensive overview, suitable for students, researchers, and anyone seeking a deeper understanding of this essential statistical concept. The information presented is current and grounded in established statistical principles.
Why the Z-Test Matters:
The z-test is a cornerstone of inferential statistics, allowing researchers to draw conclusions about a population based on a sample. Its applications span numerous fields, from healthcare and finance to engineering and social sciences. Understanding the z-test enables informed decision-making in situations where complete population data is unavailable or impractical to collect. This test's widespread use stems from its relative simplicity and the availability of readily accessible statistical tools for its calculation. Furthermore, the z-test forms the basis for understanding more complex statistical tests.
Overview: What This Article Covers:
This article provides a detailed exploration of the z-test, encompassing its underlying principles, different types of z-tests, assumptions, step-by-step calculation procedures, interpretation of results, and potential limitations. Readers will gain a comprehensive understanding of how to apply the z-test correctly and interpret its findings effectively. We will also delve into the practical applications and explore related concepts.
The Research and Effort Behind the Insights:
This article synthesizes information from leading statistical textbooks, peer-reviewed research papers, and reputable online resources. Each aspect of the z-test, from its theoretical underpinnings to its practical application, is supported by established statistical methods and principles. The explanations are designed for clarity and accessibility, avoiding unnecessary technical jargon.
Key Takeaways:
- Definition and Core Concepts: A clear explanation of the z-test, its purpose, and underlying statistical principles.
- Types of Z-Tests: Differentiation between one-sample, two-sample, and proportion z-tests with detailed examples.
- Assumptions and Conditions: A critical examination of the assumptions necessary for valid z-test application.
- Step-by-Step Calculation: A practical guide to performing z-tests manually and using statistical software.
- Interpreting Results: Guidance on understanding p-values, critical values, and making informed conclusions.
- Applications and Limitations: Real-world examples and a discussion of the z-test's limitations.
Smooth Transition to the Core Discussion:
Having established the importance and scope of this article, let's now delve into the specifics of the z-test, beginning with its fundamental definition and core concepts.
Exploring the Key Aspects of the Z-Test:
1. Definition and Core Concepts:
The z-test is a statistical test used to determine whether two population means are different when the population variances are known and the sample size is large (generally n ≥ 30). It assesses the significance of a difference between a sample mean and a population mean (one-sample z-test) or between two sample means (two-sample z-test). The test statistic, denoted as 'z', measures the distance between the sample mean and the population mean in terms of standard errors. This distance helps determine the probability of observing the sample data if there were no real difference between the means.
2. Types of Z-Tests:
-
One-Sample Z-Test: This test compares the mean of a single sample to a known population mean. It is used to determine if the sample mean is significantly different from the population mean.
-
Two-Sample Z-Test: This test compares the means of two independent samples. It determines if there's a significant difference between the means of the two populations from which the samples were drawn. There are variations depending on whether the population variances are assumed to be equal or unequal.
-
Z-Test for Proportions: This test compares two proportions or a sample proportion to a known population proportion. It's frequently used in surveys and opinion polls.
3. Assumptions and Conditions:
The validity of z-test results hinges on several crucial assumptions:
- Random Sampling: The samples must be randomly selected from their respective populations to ensure representativeness.
- Independence: Observations within each sample must be independent of each other.
- Normality: While the Central Limit Theorem often mitigates this requirement for larger sample sizes, ideally, the population data should be normally distributed or the sample size should be sufficiently large (n ≥ 30) to approximate normality. For smaller samples, alternative tests (like the t-test) might be more appropriate.
- Known Population Variance (or Large Sample Size): For one-sample and two-sample z-tests, the population variance (σ²) must be known. If it's unknown, the t-test is generally preferred. However, for larger sample sizes, the sample variance can be used as an estimate of the population variance.
4. Step-by-Step Calculation:
The calculation process for each type of z-test varies slightly but generally involves these steps:
- State the Hypotheses: Define the null hypothesis (H₀) and the alternative hypothesis (Hₐ). The null hypothesis typically states that there is no significant difference between the means or proportions being compared.
- Calculate the Test Statistic (z): This involves using the appropriate formula for the chosen z-test. The formulas differ based on the type of z-test (one-sample, two-sample, proportion). They incorporate the sample means, population means (if known), population standard deviations, and sample sizes.
- Determine the p-value: The p-value represents the probability of obtaining the observed results (or more extreme results) if the null hypothesis is true. A smaller p-value indicates stronger evidence against the null hypothesis. Statistical software or z-tables are used to determine the p-value corresponding to the calculated z-statistic.
- Set the Significance Level (α): This is typically set at 0.05 (5%), indicating a 5% chance of rejecting the null hypothesis when it is actually true (Type I error).
- Make a Decision: If the p-value is less than or equal to the significance level (p ≤ α), the null hypothesis is rejected. If the p-value is greater than the significance level (p > α), the null hypothesis is not rejected.
5. Interpreting Results:
The interpretation of the z-test results focuses on the p-value and the decision regarding the null hypothesis. A low p-value (typically below 0.05) suggests strong evidence against the null hypothesis, leading to its rejection. This implies that a statistically significant difference exists between the means or proportions being compared. Conversely, a high p-value (above 0.05) indicates insufficient evidence to reject the null hypothesis, suggesting no statistically significant difference. It's crucial to remember that statistical significance doesn't necessarily imply practical significance.
6. Applications and Limitations:
Z-tests find extensive applications in various fields:
- Quality Control: Monitoring product dimensions or characteristics.
- Clinical Trials: Comparing treatment effects.
- Market Research: Assessing consumer preferences.
- Finance: Analyzing investment returns.
However, the z-test has limitations:
- Assumption of Known Population Variance: This assumption is often unrealistic in practice.
- Sensitivity to Outliers: Outliers can significantly influence the results.
- Requirement for Large Sample Sizes: The accuracy of the test improves with larger sample sizes.
Exploring the Connection Between Sample Size and Z-Test Validity:
The relationship between sample size and z-test validity is crucial. While the z-test assumes a known population variance, the Central Limit Theorem states that the sampling distribution of the mean approximates a normal distribution even if the population is not normally distributed, provided the sample size is sufficiently large (generally n ≥ 30). This means that with a large enough sample, the z-test can provide reasonably accurate results even if the normality assumption is slightly violated. However, smaller samples necessitate a more cautious approach, potentially requiring the use of the t-test, which is less sensitive to deviations from normality.
Key Factors to Consider:
-
Roles and Real-World Examples: Larger sample sizes are essential in studies with small effect sizes to achieve sufficient statistical power and avoid Type II errors (failing to reject the null hypothesis when it's false). For instance, in clinical trials, a larger sample size increases the chance of detecting a small but meaningful difference between treatments.
-
Risks and Mitigations: Relying on smaller sample sizes without considering the potential for increased Type II error rates can lead to inaccurate conclusions. Using power analysis before conducting a study helps determine the appropriate sample size to achieve a desired level of power.
-
Impact and Implications: The choice of sample size directly impacts the reliability and generalizability of the z-test results. Underpowered studies might fail to detect true effects, while overly powered studies might be unnecessarily costly and time-consuming.
Conclusion: Reinforcing the Connection:
The connection between sample size and z-test validity emphasizes the importance of carefully considering sample size selection before conducting a study. Using appropriate statistical methods, such as power analysis, helps ensure that the z-test yields reliable and meaningful results.
Further Analysis: Examining Sample Size Determination in Greater Detail:
Power analysis is a critical step in planning research using the z-test. It involves determining the minimum sample size needed to detect a specified effect size with a desired level of power and significance level. Power is the probability of correctly rejecting the null hypothesis when it is false. Factors that influence sample size determination include the desired power, the significance level, the effect size, and the variability in the data. Software packages and online calculators can assist in performing power analysis.
FAQ Section: Answering Common Questions About the Z-Test:
-
What is a z-test? A z-test is a statistical test used to compare the means of two populations or a sample mean to a population mean when the population variance is known or the sample size is large.
-
When should I use a z-test instead of a t-test? Use a z-test when the population variance is known, or when the sample size is large (generally n ≥ 30) allowing the sample variance to be a good estimate of the population variance. If the population variance is unknown and the sample size is small, use a t-test.
-
What does the p-value tell me? The p-value represents the probability of observing the obtained results (or more extreme results) if the null hypothesis is true. A low p-value suggests strong evidence against the null hypothesis.
-
What is a Type I error? A Type I error occurs when the null hypothesis is rejected when it is actually true. The significance level (α) determines the probability of making a Type I error.
-
What is a Type II error? A Type II error occurs when the null hypothesis is not rejected when it is actually false. The power of a test is the probability of avoiding a Type II error.
Practical Tips: Maximizing the Benefits of Z-Tests:
- Clearly Define Hypotheses: Ensure that the null and alternative hypotheses are precisely stated before beginning the analysis.
- Verify Assumptions: Check that the assumptions of the z-test are met before proceeding.
- Use Appropriate Software: Statistical software packages simplify the calculation and interpretation of z-tests.
- Consider Effect Size: Statistical significance alone doesn't tell the whole story; consider the practical importance of the findings.
- Report Results Clearly: Present the results in a way that is easily understandable to your intended audience.
Final Conclusion: Wrapping Up with Lasting Insights:
The z-test, despite its limitations, remains a powerful tool in statistical inference. Understanding its principles, assumptions, and applications allows for informed decision-making across numerous disciplines. By carefully considering the assumptions and interpreting the results in context, researchers can leverage the z-test to extract valuable insights from data and make evidence-based conclusions. Remember that responsible data analysis requires careful consideration of the limitations of any statistical method.
Latest Posts
Latest Posts
-
How To Beat A Credit Check
Apr 04, 2025
-
How To Pass A Credit Check For An Apartment
Apr 04, 2025
-
How To Pass A Credit Check For A Job
Apr 04, 2025
-
How To Pass A Credit Check For Renting
Apr 04, 2025
-
How To Pass A Credit Check With Bad Credit
Apr 04, 2025
Related Post
Thank you for visiting our website which covers about Definition Z Test . We hope the information provided has been useful to you. Feel free to contact us if you have any questions or need further assistance. See you next time and don't miss to bookmark.