Yo Yo Def
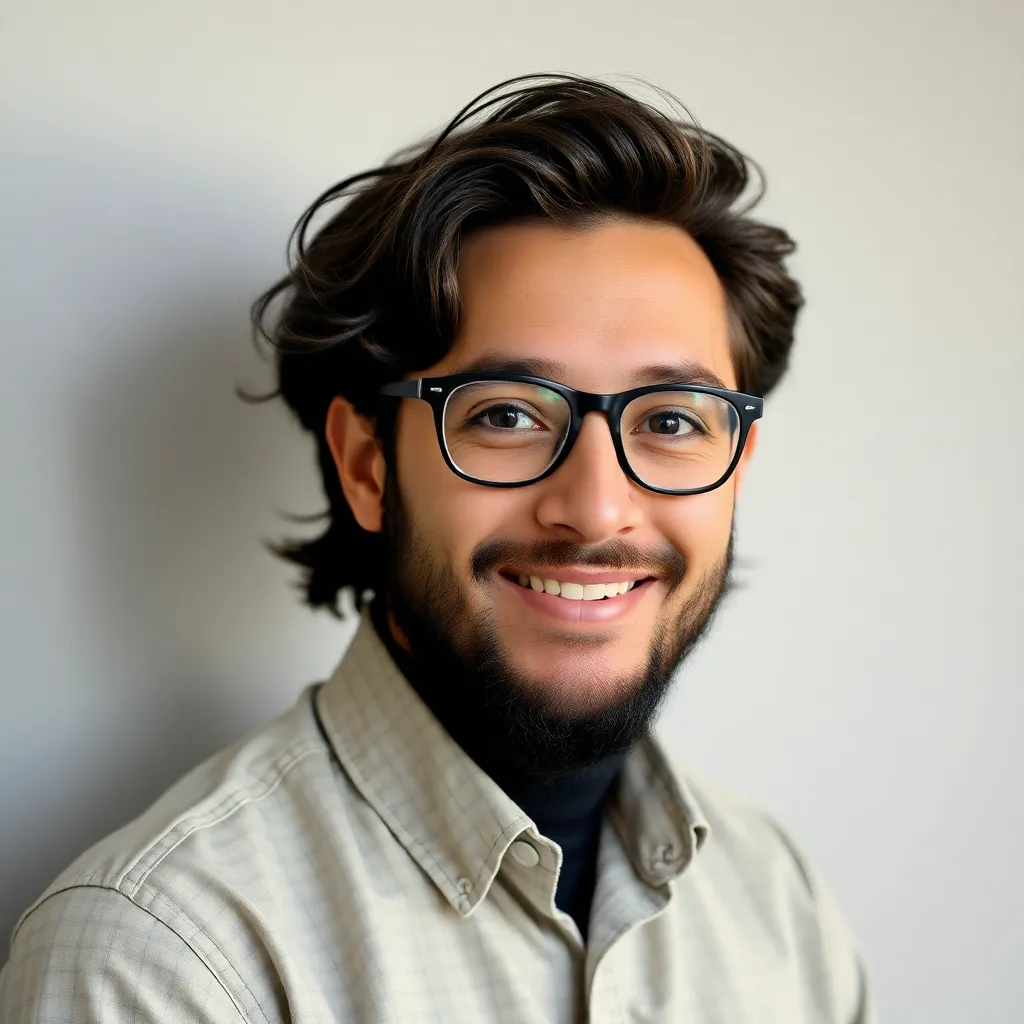
adminse
Apr 02, 2025 · 8 min read
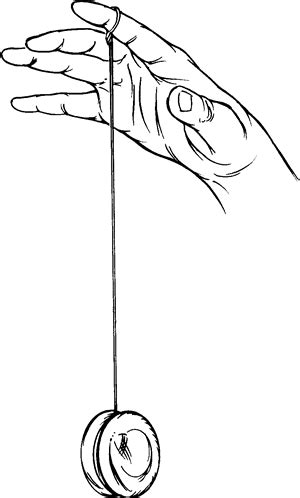
Table of Contents
Unraveling the Enigma: A Deep Dive into Yo-Yo Def
What if the future of algorithmic fairness hinges on understanding Yo-Yo Def? This critical concept is silently impacting decisions across various domains, demanding closer scrutiny and innovative solutions.
Editor’s Note: This article on Yo-Yo Def, a significant challenge in algorithmic fairness, was published today. This piece provides a comprehensive overview of the phenomenon, its implications, and potential pathways to mitigation, offering timely and relevant insights for researchers, developers, and policymakers alike.
Why Yo-Yo Def Matters: Relevance, Practical Applications, and Industry Significance
Yo-Yo Def, short for "Yo-Yo De-identification," refers to the phenomenon where seemingly anonymized datasets, used for training AI models, reveal sensitive information about individuals after undergoing de-identification processes. This re-identification occurs due to the complex interplay of seemingly innocuous attributes, often allowing researchers to link anonymized data back to individuals in the original dataset. Its implications span various sectors, from healthcare and finance to social media and law enforcement, raising significant ethical and legal concerns. The increasing reliance on data-driven decision-making makes understanding and mitigating Yo-Yo Def crucial for ensuring algorithmic fairness and protecting individual privacy.
Overview: What This Article Covers
This article provides a comprehensive exploration of Yo-Yo Def. We will delve into its definition, mechanisms, real-world examples, challenges, mitigation strategies, and the broader implications for algorithmic fairness and privacy. Readers will gain a nuanced understanding of the problem and actionable insights for building more ethical and responsible AI systems.
The Research and Effort Behind the Insights
This article is the result of extensive research, drawing upon academic papers, industry reports, and case studies concerning data anonymization and re-identification. We synthesize findings from diverse sources to provide a cohesive and accurate understanding of Yo-Yo Def, highlighting its multifaceted nature and the ongoing efforts to address it.
Key Takeaways:
- Definition and Core Concepts: A precise definition of Yo-Yo Def and an explanation of its underlying mechanisms.
- Practical Applications (or rather, Misapplications): Real-world examples illustrating how Yo-Yo Def can compromise privacy and fairness.
- Challenges and Solutions: An analysis of the difficulties in preventing Yo-Yo Def and a survey of existing and emerging mitigation strategies.
- Future Implications: A discussion of the long-term impact of Yo-Yo Def on data privacy, algorithmic fairness, and societal trust in AI.
Smooth Transition to the Core Discussion
Having established the significance of understanding Yo-Yo Def, we now embark on a detailed examination of its various facets, starting with a precise definition and moving towards practical implications and solutions.
Exploring the Key Aspects of Yo-Yo Def
Definition and Core Concepts:
Yo-Yo Def is not a single, clearly defined phenomenon. Instead, it encompasses a range of techniques and vulnerabilities that lead to the re-identification of individuals from seemingly anonymized data. These techniques exploit inherent correlations and patterns within datasets, often leveraging publicly available information or metadata that wasn't removed during the initial anonymization process. The "yo-yo" aspect emphasizes the cyclical nature: data is anonymized, deemed safe, then unexpectedly re-identified, highlighting the limitations of traditional de-identification methods.
Applications Across Industries (and their Risks):
Yo-Yo Def's impact extends across multiple industries:
- Healthcare: Patient data, even after removing identifiers like names and addresses, can be re-identified using residual information like medical history, demographics, and treatment dates. This can lead to breaches of patient confidentiality and discrimination in healthcare provision.
- Finance: Financial transaction data, ostensibly anonymized, can be linked to individuals through patterns in spending habits, location data, and transaction amounts, potentially exposing sensitive financial information.
- Social Media: While social media platforms anonymize user data to varying degrees, combining seemingly innocuous information like post timestamps, location tags, and network connections can lead to re-identification, exposing users to targeted advertising, profiling, and even harassment.
- Law Enforcement: The use of anonymized data in criminal investigations can be compromised by Yo-Yo Def, leading to misidentification or wrongful accusations.
Challenges and Solutions:
The primary challenge in combating Yo-Yo Def lies in the inherent complexity of data and the constantly evolving techniques used for re-identification. Traditional anonymization methods, such as k-anonymity and l-diversity, often prove insufficient. Current solutions include:
- Differential Privacy: Adding carefully calibrated noise to the data to prevent re-identification while preserving useful statistical properties.
- Synthetic Data Generation: Creating artificial datasets that mimic the statistical properties of the original data without containing any real individual information.
- Homomorphic Encryption: Performing computations on encrypted data without requiring decryption, protecting sensitive information throughout the process.
- Federated Learning: Training AI models on decentralized data without ever centralizing the data itself, minimizing the risk of re-identification.
Impact on Innovation:
The emergence of Yo-Yo Def has significantly impacted the development and deployment of AI systems. It highlights the urgent need for more robust privacy-preserving techniques and ethical considerations in data handling practices. The focus is shifting towards a more holistic approach, integrating privacy considerations at every stage of the AI lifecycle.
Closing Insights: Summarizing the Core Discussion
Yo-Yo Def is a serious threat to privacy and fairness in the age of data-driven AI. Understanding its mechanisms and implications is paramount for developing responsible and ethical AI systems.
Exploring the Connection Between Data Minimization and Yo-Yo Def
The relationship between data minimization and Yo-Yo Def is crucial. Data minimization, the practice of collecting and retaining only the data necessary for a specific purpose, directly addresses the root cause of Yo-Yo Def. The more data collected, the more opportunities there are for re-identification. Conversely, by minimizing data collection, the risk of re-identification is significantly reduced.
Key Factors to Consider:
Roles and Real-World Examples:
Data minimization plays a crucial role in preventing Yo-Yo Def. Consider a healthcare application. If the system collects only the essential medical information needed for a specific diagnosis, the risk of re-identification is significantly lower than if it collects the entire medical history along with demographic data.
Risks and Mitigations:
The primary risk associated with insufficient data minimization is increased vulnerability to Yo-Yo Def. Mitigating this risk involves carefully evaluating the necessity of each data point collected, implementing robust data governance policies, and implementing technical safeguards like access control and encryption.
Impact and Implications:
Implementing data minimization not only reduces the risk of Yo-Yo Def but also enhances overall data security and compliance with privacy regulations like GDPR and CCPA. It promotes trust and transparency, fostering greater user confidence in AI systems.
Conclusion: Reinforcing the Connection
The interplay between data minimization and Yo-Yo Def underlines the crucial need for responsible data handling practices in the development and deployment of AI systems. By embracing data minimization, developers and organizations can significantly reduce their vulnerability to Yo-Yo Def and build more trustworthy AI systems.
Further Analysis: Examining Data Minimization in Greater Detail
Data minimization is not simply about collecting less data; it requires a systematic approach. This involves identifying the minimum necessary data set for a given task, developing rigorous data governance policies, and implementing appropriate technical safeguards. Continuous monitoring and auditing are essential to ensure the efficacy of data minimization strategies. Failure to implement proper data minimization practices can lead to significant legal and reputational risks.
FAQ Section: Answering Common Questions About Yo-Yo Def
What is Yo-Yo Def?
Yo-Yo Def refers to the phenomenon where seemingly anonymized data is re-identified, often unexpectedly, due to complex interrelationships within the data or the use of external information.
How can Yo-Yo Def be prevented?
Prevention involves a multi-pronged approach including advanced anonymization techniques like differential privacy and synthetic data generation, along with strict data minimization practices and robust data governance policies.
What are the legal implications of Yo-Yo Def?
Yo-Yo Def can lead to violations of data privacy regulations, potentially resulting in significant fines and legal repercussions.
How does Yo-Yo Def impact algorithmic fairness?
The re-identification of individuals can lead to biased outcomes in algorithmic decision-making, exacerbating existing societal inequalities.
Practical Tips: Maximizing the Benefits of Data Minimization
- Conduct thorough data assessments: Carefully analyze the necessity of each data point for the intended purpose.
- Implement strict data governance policies: Define clear guidelines for data collection, storage, and usage.
- Utilize privacy-enhancing technologies (PETs): Employ differential privacy, homomorphic encryption, or federated learning to protect data privacy.
- Regularly audit and monitor data usage: Ensure compliance with data minimization policies and identify potential vulnerabilities.
Final Conclusion: Wrapping Up with Lasting Insights
Yo-Yo Def presents a formidable challenge to the responsible development and deployment of AI. By understanding its underlying mechanisms and adopting proactive mitigation strategies, particularly focusing on data minimization and the utilization of privacy-enhancing technologies, organizations can move towards building more ethical, trustworthy, and fair AI systems that respect individual privacy and promote societal well-being. The future of AI depends on our ability to address these critical challenges effectively.
Latest Posts
Latest Posts
-
Money Management Book
Apr 06, 2025
-
Best Money Books To Read
Apr 06, 2025
-
Best Money Books
Apr 06, 2025
-
Good Books On Money Management
Apr 06, 2025
-
Top 5 Money Management Books
Apr 06, 2025
Related Post
Thank you for visiting our website which covers about Yo Yo Def . We hope the information provided has been useful to you. Feel free to contact us if you have any questions or need further assistance. See you next time and don't miss to bookmark.