What Is The Pearson Coefficient Definition Benefits And History
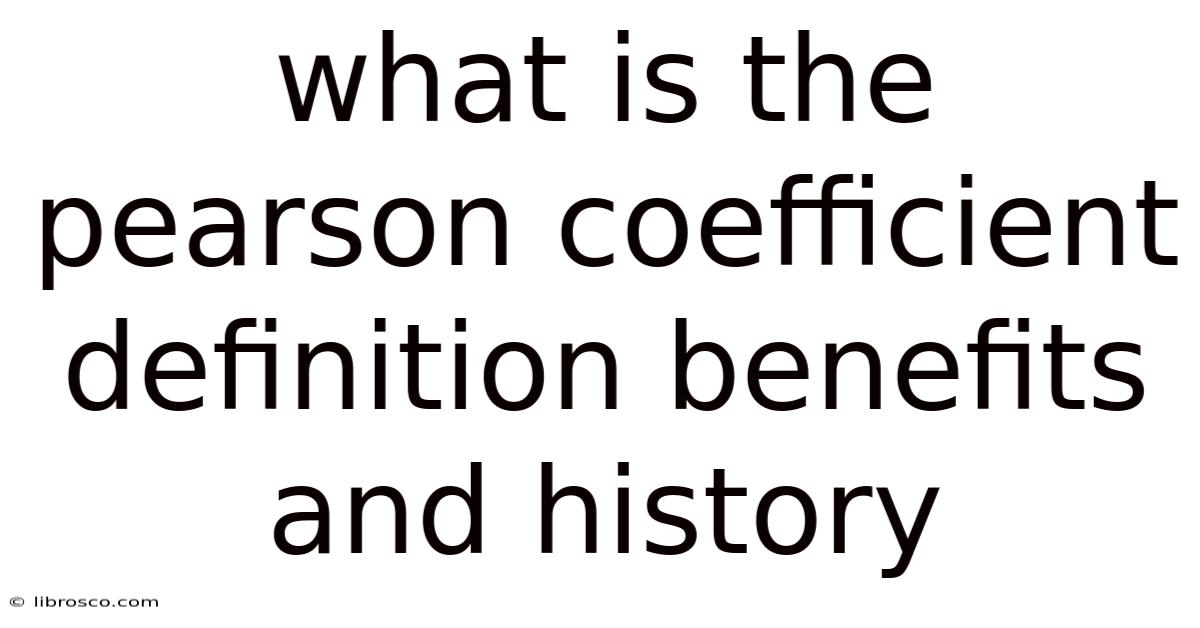
Discover more detailed and exciting information on our website. Click the link below to start your adventure: Visit Best Website meltwatermedia.ca. Don't miss out!
Table of Contents
Unlocking the Secrets of Correlation: A Deep Dive into Pearson's Coefficient
What if understanding the strength and direction of relationships between variables could unlock a world of predictive power? Pearson's correlation coefficient, a cornerstone of statistical analysis, provides precisely that, offering invaluable insights across diverse fields.
Editor’s Note: This article on Pearson's correlation coefficient provides a comprehensive overview of its definition, benefits, history, and applications. Updated with the latest understanding, it serves as a valuable resource for students, researchers, and anyone interested in understanding the power of correlation analysis.
Why Pearson's Correlation Coefficient Matters:
Pearson's correlation coefficient, often denoted as r, is a fundamental statistical measure quantifying the linear association between two continuous variables. Understanding this coefficient is crucial because it allows researchers to move beyond simply observing relationships to measuring their strength and direction. This, in turn, enables more accurate predictions, informed decision-making, and a deeper understanding of complex phenomena across numerous disciplines, from finance and healthcare to social sciences and environmental studies. Its widespread applicability stems from its ability to quantify the degree to which changes in one variable are associated with changes in another. This is valuable for identifying potential causal links (though correlation does not equal causation), predicting outcomes, and developing more effective models.
Overview: What This Article Covers:
This article embarks on a journey through the world of Pearson's correlation coefficient, starting with its precise definition and delving into its historical context. We will explore its practical applications across various fields, examine its strengths and limitations, and address common misconceptions surrounding its interpretation. Finally, we will provide a detailed explanation of how to calculate and interpret the coefficient, making this complex statistical concept accessible to a broader audience.
The Research and Effort Behind the Insights:
The information presented in this article is grounded in extensive research, drawing upon seminal statistical texts, peer-reviewed journal articles, and reputable online resources. The historical context is meticulously researched, ensuring accurate representation of the evolution of this crucial statistical tool. The explanations are designed to be clear, concise, and accessible, employing various illustrative examples to solidify understanding.
Key Takeaways:
- Definition and Core Concepts: A precise definition of Pearson's r and the concepts of linear correlation, positive and negative correlation, and the range of the coefficient (-1 to +1).
- Practical Applications: Exploring the diverse applications of Pearson's r across various fields, including examples and case studies.
- Calculation and Interpretation: A step-by-step guide to calculating r, including formula explanations and interpretations of different values.
- Strengths and Limitations: A balanced assessment of the benefits and drawbacks of using Pearson's r, including potential pitfalls and alternative correlation measures.
- Historical Context: Tracing the development and evolution of Pearson's r, highlighting its contributions to statistical analysis.
Smooth Transition to the Core Discussion:
Having established the importance and scope of this article, let's now delve into the heart of the matter: a comprehensive exploration of Pearson's correlation coefficient.
Exploring the Key Aspects of Pearson's Correlation Coefficient:
1. Definition and Core Concepts:
Pearson's correlation coefficient measures the linear relationship between two variables. A linear relationship implies that the change in one variable is directly proportional to the change in the other. The coefficient ranges from -1 to +1:
- +1: Indicates a perfect positive correlation. As one variable increases, the other increases proportionally.
- 0: Indicates no linear correlation. There's no consistent relationship between the changes in the two variables.
- -1: Indicates a perfect negative correlation. As one variable increases, the other decreases proportionally.
Values between -1 and +1 represent varying degrees of correlation strength. For instance, r = 0.8 signifies a strong positive correlation, while r = -0.5 indicates a moderate negative correlation.
2. Applications Across Industries:
The applications of Pearson's r are incredibly diverse:
- Finance: Assessing the relationship between stock prices, interest rates, and economic indicators.
- Healthcare: Investigating correlations between lifestyle factors (diet, exercise) and health outcomes.
- Education: Examining the relationship between study time and academic performance.
- Social Sciences: Analyzing correlations between social factors (income, education) and various social outcomes.
- Environmental Science: Studying the correlation between pollution levels and environmental health indicators.
3. Challenges and Solutions:
While powerful, Pearson's r has limitations:
- Non-linear relationships: It only measures linear relationships. A strong non-linear relationship might yield a low r value.
- Outliers: Extreme data points can significantly influence the correlation coefficient.
- Causation vs. Correlation: A high correlation doesn't imply causation. Other factors might be involved.
Solutions include:
- Data transformation: Transforming non-linear data to make it more linear.
- Outlier analysis: Identifying and addressing outliers before calculating r.
- Controlling for confounding variables: Using statistical methods to account for the influence of other factors.
4. Impact on Innovation:
Pearson's r has significantly impacted various fields by enabling:
- Predictive modeling: Developing models to predict future outcomes based on observed correlations.
- Risk assessment: Identifying potential risks by analyzing correlations between different factors.
- Improved decision-making: Making better decisions based on data-driven insights.
Closing Insights: Summarizing the Core Discussion:
Pearson's correlation coefficient is a fundamental tool for understanding the linear relationship between variables. Its ability to quantify the strength and direction of these relationships has profoundly impacted scientific inquiry and decision-making across a broad range of fields. However, it's crucial to understand its limitations and interpret the results cautiously, recognizing that correlation does not necessarily imply causation.
Exploring the Connection Between Sample Size and Pearson's Correlation Coefficient:
The sample size significantly influences the reliability and statistical significance of a Pearson's correlation coefficient. A larger sample size generally leads to a more accurate and stable estimate of the population correlation. With smaller sample sizes, the calculated r can be heavily influenced by random variation, leading to potentially misleading conclusions. Statistical tests, such as the t-test, are used to assess the significance of the correlation, taking into account the sample size. A larger sample size increases the power of these tests, making it more likely to detect a true correlation if one exists.
Key Factors to Consider:
- Roles and Real-World Examples: In a clinical trial investigating the relationship between a new drug and blood pressure, a larger sample size would provide a more reliable estimate of the correlation. A smaller sample size might yield a significant correlation due to chance alone.
- Risks and Mitigations: Small sample sizes increase the risk of Type I error (false positive) – concluding a significant correlation when none exists in the population. Mitigating this risk involves using larger sample sizes and adjusting significance levels accordingly.
- Impact and Implications: The impact of sample size on the interpretation of Pearson's r is crucial for drawing valid conclusions. Ignoring sample size can lead to inaccurate inferences and flawed decision-making.
Conclusion: Reinforcing the Connection:
The relationship between sample size and Pearson's r is inseparable. Larger sample sizes lead to more reliable and statistically significant results, reducing the risk of erroneous conclusions. Researchers must carefully consider sample size when designing studies and interpreting correlation coefficients.
Further Analysis: Examining the Statistical Significance of Pearson's r
The statistical significance of Pearson's r indicates the probability that the observed correlation is not due to random chance. This is usually determined using a t-test, which calculates a t-statistic based on the calculated r, sample size, and degrees of freedom. The p-value associated with the t-statistic indicates the probability of observing such a correlation (or a stronger one) if there were no true correlation in the population. A small p-value (typically below 0.05) indicates that the correlation is statistically significant, meaning it's unlikely to be due to chance.
FAQ Section: Answering Common Questions About Pearson's Correlation Coefficient:
- What is Pearson's correlation coefficient? Pearson's r is a statistical measure quantifying the linear association between two continuous variables, ranging from -1 to +1.
- How is Pearson's r calculated? The calculation involves a formula using the covariance of the two variables and their standard deviations. Software packages readily perform this calculation.
- What does a Pearson's r of 0.7 mean? This indicates a strong positive correlation; as one variable increases, the other tends to increase.
- Can Pearson's r be used for non-linear relationships? No, Pearson's r is specifically designed for linear relationships. Other correlation measures are more suitable for non-linear data.
- What is the difference between correlation and causation? Correlation indicates an association, but it doesn't necessarily imply causation. Other factors could be involved.
Practical Tips: Maximizing the Benefits of Pearson's Correlation Coefficient:
- Understand the assumptions: Ensure the data meets the assumptions of linearity and normality before calculating r.
- Check for outliers: Identify and address potential outliers that might distort the results.
- Consider sample size: Use a sufficiently large sample size to ensure the reliability of the results.
- Interpret cautiously: Remember that correlation doesn't imply causation. Explore potential confounding variables.
- Use appropriate software: Statistical software packages simplify the calculation and interpretation of Pearson's r.
Final Conclusion: Wrapping Up with Lasting Insights:
Pearson's correlation coefficient remains a cornerstone of statistical analysis, providing a powerful tool for understanding the relationships between variables. By carefully considering its assumptions, limitations, and appropriate interpretations, researchers and analysts can leverage its immense power to draw meaningful insights from data and make informed decisions. Its enduring relevance stems from its simplicity, widespread applicability, and ability to quantify a fundamental aspect of data relationships – the linear correlation. Understanding Pearson's r is crucial for navigating the world of data analysis and extracting meaningful information for a wide variety of applications.
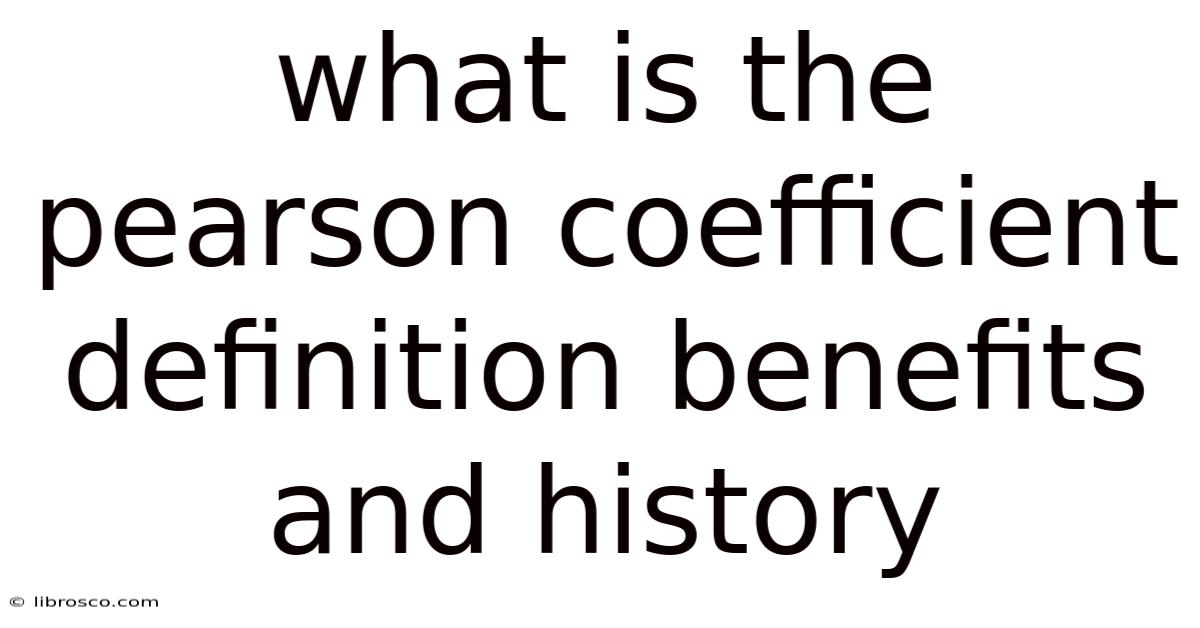
Thank you for visiting our website wich cover about What Is The Pearson Coefficient Definition Benefits And History. We hope the information provided has been useful to you. Feel free to contact us if you have any questions or need further assistance. See you next time and dont miss to bookmark.
Also read the following articles
Article Title | Date |
---|---|
Overnight Position Definition Risks And Benefits In Trading | Mar 09, 2025 |
Par Yield Curve Definition Calculation Vs Spot Curve | Mar 09, 2025 |
Owners Equivalent Rent Oer Definition And Relationship To Cpi | Mar 09, 2025 |
Overnight Limit Definition | Mar 09, 2025 |
Operation Twist Definition How It Works And Economic Impact | Mar 09, 2025 |