Probability Live
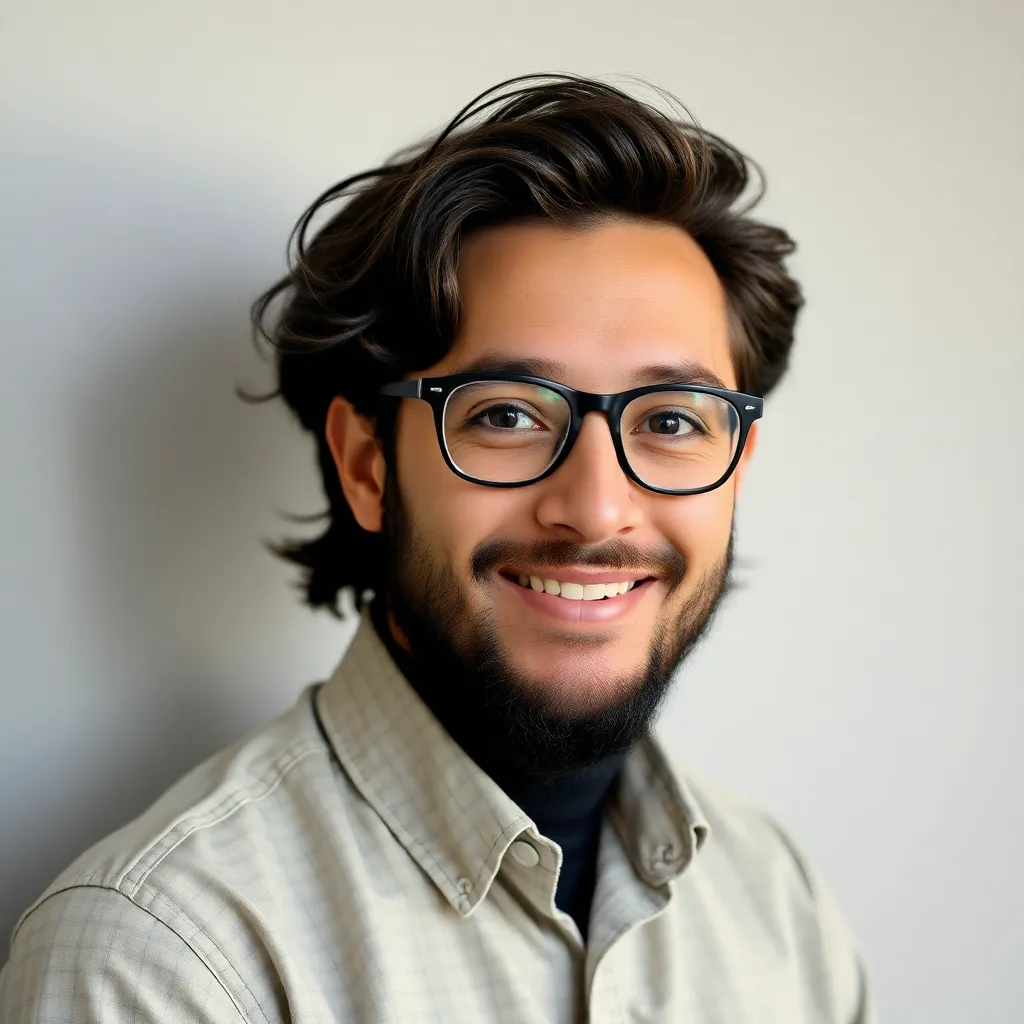
adminse
Apr 02, 2025 · 7 min read
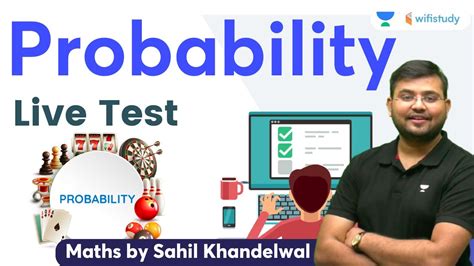
Table of Contents
Decoding the Dice: A Deep Dive into Probability Live
What if the thrill of the casino floor could be understood, not just experienced? Probability live, a vibrant field, empowers us to predict, analyze, and even influence the seemingly random outcomes of chance events.
Editor’s Note: This article on probability live, published today, explores the fascinating intersection of mathematics and real-time events. We’ll unravel the complexities of probability in dynamic situations, offering insights applicable to various fields, from gaming to finance.
Why Probability Live Matters:
Probability live concerns the application of probabilistic principles to events unfolding in real time. It's not about static calculations based on historical data; it's about analyzing probabilities as they evolve, adapting predictions based on new information, and understanding the inherent uncertainties of dynamic systems. Its relevance spans numerous domains:
-
Gambling and Gaming: In casino games, understanding probability live allows for strategic decision-making, improving odds, and managing risk. Live betting on sporting events relies heavily on real-time probability assessments, adjusting odds as the game progresses.
-
Finance and Trading: Stock prices, currency exchange rates, and other financial instruments fluctuate constantly. Probability live models help predict market trends, assess risk, and inform investment strategies.
-
Weather Forecasting: Meteorological models utilize probability live to forecast weather patterns, providing probabilities of various outcomes (rain, snow, temperature) as new data from sensors and satellites becomes available.
-
Healthcare and Epidemiology: Real-time epidemiological models use probability live to predict the spread of infectious diseases, inform public health interventions, and allocate resources effectively.
-
Robotics and Autonomous Systems: Robots and autonomous vehicles operating in dynamic environments need to make real-time decisions based on probability estimates of various scenarios (obstacle avoidance, path planning).
Overview: What This Article Covers:
This article will explore the core concepts of probability live, examining its applications across diverse fields. We'll delve into the mathematical foundations, discuss challenges in real-time probability estimation, and explore advanced techniques used to manage uncertainty and make informed decisions in dynamic environments.
The Research and Effort Behind the Insights:
This article synthesizes insights from diverse sources, including academic papers on stochastic processes, statistical modeling, and machine learning, as well as practical applications in various industries. Numerous case studies, industry reports, and expert opinions have been consulted to provide a comprehensive and accurate overview of probability live.
Key Takeaways:
- Understanding Basic Probability: A review of fundamental probabilistic concepts, including conditional probability and Bayes' theorem, essential for understanding live probability applications.
- Real-Time Data Streams: Examination of how real-time data streams are incorporated into probability models and the challenges of handling noisy and incomplete data.
- Dynamic Bayesian Networks: Exploring the use of Dynamic Bayesian Networks (DBNs) and other advanced modeling techniques for capturing temporal dependencies and making probabilistic inferences in live scenarios.
- Applications Across Industries: Case studies illustrating the practical applications of probability live in diverse sectors such as finance, gaming, and healthcare.
- Challenges and Future Directions: Addressing the limitations of current methods and highlighting the ongoing research in improving the accuracy and efficiency of probability live models.
Smooth Transition to the Core Discussion:
Having established the importance and scope of probability live, let's explore its key components and applications in greater detail.
Exploring the Key Aspects of Probability Live:
1. Fundamental Concepts:
At its core, probability live builds upon classical probability theory. Key concepts include:
- Probability Distribution: Describes the likelihood of different outcomes for a random variable. In live scenarios, these distributions are often dynamic, changing over time.
- Conditional Probability: The probability of an event occurring given that another event has already occurred. This is crucial in live settings where new information constantly updates probabilities.
- Bayes' Theorem: Provides a way to update probabilities based on new evidence. This is fundamental to Bayesian methods, widely used in probability live applications.
- Stochastic Processes: Mathematical models describing the evolution of random variables over time. These form the basis for many probability live models.
2. Real-Time Data Handling:
The core challenge of probability live lies in processing and incorporating continuous streams of data. This necessitates efficient algorithms and data structures to:
- Handle High-Volume Data: Real-time data often arrives at a high rate, requiring robust systems to manage and process the information effectively.
- Address Noisy Data: Real-world data is often noisy and contains errors. Robust estimation techniques are needed to filter out noise and extract reliable information.
- Manage Incomplete Data: In many situations, complete data may not be available. Probability live models need to handle missing data and still provide meaningful probabilistic inferences.
3. Advanced Modeling Techniques:
Various advanced modeling techniques are employed in probability live applications, including:
- Dynamic Bayesian Networks (DBNs): Represent probabilistic relationships between variables that change over time. DBNs are particularly useful for modeling complex systems with many interacting variables.
- Hidden Markov Models (HMMs): Useful for modeling systems where the underlying state is not directly observable but can be inferred from observations.
- Kalman Filters: Efficient algorithms for estimating the state of a dynamic system from noisy measurements.
- Particle Filters: A more general class of algorithms that can handle non-linear and non-Gaussian systems.
4. Applications Across Industries:
The applications of probability live are vast and diverse. Consider these examples:
- Live Sports Betting: Bookmakers use sophisticated models to adjust odds based on real-time game events, player performance, and other relevant factors.
- Algorithmic Trading: High-frequency trading algorithms use probability live models to make rapid decisions based on market data, aiming to capitalize on short-term price fluctuations.
- Weather Forecasting: Probabilistic weather forecasts are updated constantly as new data arrives from weather stations and satellites, improving accuracy over time.
- Healthcare Monitoring: Real-time monitoring systems in hospitals use probability live to assess patient risk, predict adverse events, and optimize treatment strategies.
Closing Insights: Summarizing the Core Discussion:
Probability live is not just a theoretical concept; it's a powerful tool with significant practical applications. By effectively managing real-time data streams and leveraging advanced modeling techniques, we can gain valuable insights into dynamic systems and make informed decisions under uncertainty.
Exploring the Connection Between Real-Time Data Analysis and Probability Live:
Real-time data analysis is inextricably linked to probability live. The ability to analyze data as it arrives is crucial for updating probabilistic models and making accurate predictions.
Key Factors to Consider:
- Data Acquisition and Processing: Efficient and reliable methods for collecting, cleaning, and processing real-time data are essential.
- Model Selection and Parameter Estimation: Choosing appropriate probabilistic models and estimating their parameters based on real-time data is a critical step.
- Model Validation and Calibration: Regularly validating and calibrating models ensures their accuracy and reliability.
- Computational Resources: Handling large volumes of real-time data requires substantial computational power.
Conclusion: Reinforcing the Connection:
The seamless integration of real-time data analysis and advanced probabilistic modeling is fundamental to successful probability live applications. Continuous improvement in data acquisition, modeling techniques, and computational resources will further enhance the accuracy and impact of probability live across numerous domains.
Further Analysis: Examining Bayesian Methods in Greater Detail:
Bayesian methods are particularly well-suited for probability live applications because they provide a principled way to update probabilities as new data becomes available. Bayes' theorem, the cornerstone of Bayesian inference, allows us to combine prior knowledge with new evidence to obtain updated posterior probabilities.
FAQ Section: Answering Common Questions About Probability Live:
-
What is the difference between classical probability and probability live? Classical probability deals with static probabilities based on historical data, while probability live involves dynamic probabilities that change over time.
-
What are some common challenges in implementing probability live systems? Challenges include handling high-volume data streams, dealing with noisy and incomplete data, and selecting appropriate probabilistic models.
-
What are the ethical considerations of using probability live in decision-making? Ethical considerations include ensuring fairness, transparency, and accountability in the use of probabilistic models, especially in high-stakes applications like algorithmic trading or healthcare.
Practical Tips: Maximizing the Benefits of Probability Live:
- Start with a clear definition of the problem: Identify the specific question you are trying to answer with a probability live model.
- Choose an appropriate probabilistic model: Select a model that is well-suited to the nature of the data and the problem at hand.
- Validate your model rigorously: Test your model on historical data to ensure its accuracy and reliability before deploying it in a live setting.
- Monitor performance continuously: Track the performance of your model in real time and make adjustments as needed.
Final Conclusion: Wrapping Up with Lasting Insights:
Probability live is a rapidly evolving field with the potential to transform numerous industries. By embracing advanced probabilistic modeling techniques and leveraging real-time data analysis, we can make more informed decisions, manage risks more effectively, and unlock new opportunities in a world of uncertainty. The continued advancement of computational power and sophisticated algorithms promises even greater accuracy and broader applications for probability live in the years to come.
Latest Posts
Latest Posts
-
Money Management Book
Apr 06, 2025
-
Best Money Books To Read
Apr 06, 2025
-
Best Money Books
Apr 06, 2025
-
Good Books On Money Management
Apr 06, 2025
-
Top 5 Money Management Books
Apr 06, 2025
Related Post
Thank you for visiting our website which covers about Probability Live . We hope the information provided has been useful to you. Feel free to contact us if you have any questions or need further assistance. See you next time and don't miss to bookmark.