Matching Concept Example
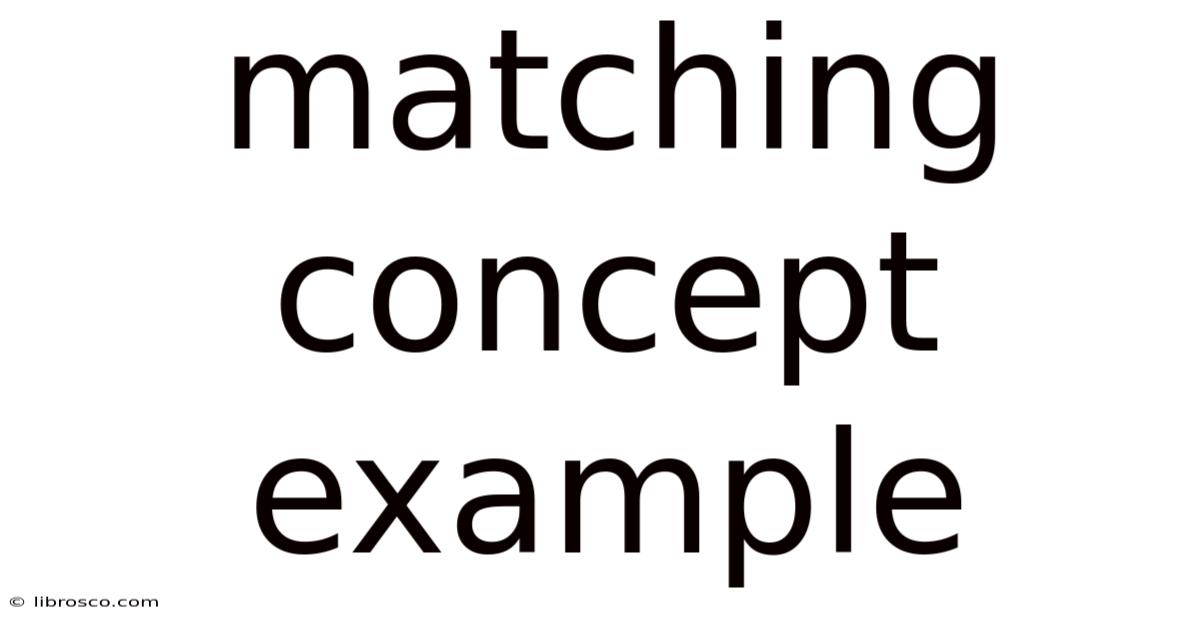
Discover more detailed and exciting information on our website. Click the link below to start your adventure: Visit Best Website meltwatermedia.ca. Don't miss out!
Table of Contents
Unlocking Potential: A Deep Dive into Matching Concept Examples
What if the seemingly simple act of matching could unlock unprecedented levels of efficiency and innovation? This fundamental concept, often overlooked, underpins countless processes across diverse fields, offering powerful solutions to complex challenges.
Editor’s Note: This article on matching concepts provides a comprehensive exploration of the topic, drawing upon real-world examples and expert insights. Updated [Date], this resource offers practical applications and valuable insights for anyone seeking to understand and leverage the power of matching.
Why Matching Matters: Relevance, Practical Applications, and Industry Significance
Matching, at its core, involves finding correspondences or similarities between elements within a dataset or across different datasets. This deceptively simple process has far-reaching implications, impacting everything from personalized recommendations in e-commerce to advanced algorithms in medical diagnostics. Its relevance stems from its ability to optimize resource allocation, improve decision-making, and drive innovation across industries. From optimizing supply chains to enhancing customer experiences, the practical applications of matching are vast and transformative.
Overview: What This Article Covers
This article delves into the multifaceted nature of matching concepts, examining various types of matching, their applications in different industries, and the underlying algorithms and techniques that make them possible. Readers will gain a comprehensive understanding of the power and limitations of matching, along with actionable insights and real-world examples.
The Research and Effort Behind the Insights
This article is the result of extensive research, incorporating insights from academic publications, industry reports, and real-world case studies. The analysis integrates diverse perspectives, ensuring a balanced and comprehensive understanding of matching concepts and their applications.
Key Takeaways:
- Definition and Core Concepts: A precise definition of matching and its fundamental principles.
- Types of Matching: Exploration of different matching techniques, including exact matching, fuzzy matching, and probabilistic matching.
- Applications Across Industries: Examples of matching's use in e-commerce, healthcare, finance, and other sectors.
- Challenges and Solutions: Identification of common challenges associated with matching and strategies to overcome them.
- Future Implications: Discussion of the evolving role of matching in the context of big data and artificial intelligence.
Smooth Transition to the Core Discussion
Having established the significance of matching, let's now explore its diverse facets and applications in greater detail. We'll examine various matching techniques, dissect real-world applications, and discuss the challenges and future prospects of this transformative concept.
Exploring the Key Aspects of Matching Concepts
1. Definition and Core Concepts:
Matching, in its broadest sense, is the process of identifying pairs or groups of items that share specific characteristics or attributes. This process can be simple, such as matching socks based on color and size, or highly complex, involving sophisticated algorithms to identify patterns in large datasets. The core principle remains consistent: finding correspondences between data points.
2. Types of Matching:
Several types of matching exist, each suited to different data and objectives:
-
Exact Matching: This involves finding perfect matches between data points based on identical values in specified attributes. For example, matching customer IDs in two databases to ensure data consistency.
-
Fuzzy Matching: This deals with imperfect matches, where data points may have slight variations. Algorithms like Levenshtein distance are used to quantify the similarity between strings, enabling matching even with typos or minor discrepancies. For example, matching customer names with slight spelling variations.
-
Probabilistic Matching: This approach assigns probabilities to potential matches based on the likelihood of similarity. It's particularly useful when dealing with incomplete or uncertain data. For example, matching medical records based on partial patient information.
-
Record Linkage: This focuses on linking records from different sources that represent the same entity, often requiring sophisticated techniques to handle inconsistencies and missing data. This is crucial in epidemiological studies or customer relationship management.
3. Applications Across Industries:
The applications of matching are diverse and impactful:
-
E-commerce: Personalized recommendations, fraud detection, and targeted advertising all rely heavily on matching algorithms. Matching customer preferences with product attributes drives sales and enhances the customer experience.
-
Healthcare: Matching patient records across different hospitals or systems is essential for accurate diagnosis and treatment. Matching genetic data helps identify disease predispositions and personalize therapies.
-
Finance: Matching transactions to prevent fraud, identifying duplicate accounts, and risk assessment all benefit from advanced matching techniques.
-
Marketing: Matching customer demographics with marketing campaigns increases campaign effectiveness and return on investment.
-
Supply Chain Management: Matching inventory levels with demand forecasts optimizes logistics and reduces waste.
-
Data Integration: Matching records across disparate databases is crucial for creating a unified and consistent view of information.
4. Challenges and Solutions:
Implementing matching techniques presents several challenges:
-
Data Quality: Inconsistent, incomplete, or inaccurate data can significantly hinder matching accuracy. Data cleansing and preprocessing are essential steps.
-
Scalability: Matching large datasets requires efficient algorithms and computational resources. Distributed computing and parallel processing techniques are often necessary.
-
Computational Complexity: Advanced matching algorithms can be computationally intensive, particularly for fuzzy or probabilistic matching. Optimization strategies are needed to manage computational costs.
-
Privacy Concerns: Matching techniques can raise privacy concerns, particularly when dealing with sensitive personal information. Data anonymization and security protocols are critical.
5. Impact on Innovation:
Matching is not merely a technical process; it's a driver of innovation. By facilitating the identification of patterns and relationships within data, matching enables new insights, improved decision-making, and the development of novel products and services. The continuous refinement of matching algorithms and their integration with other technologies, such as artificial intelligence and machine learning, will continue to fuel innovation across various fields.
Closing Insights: Summarizing the Core Discussion
Matching is a fundamental concept with far-reaching implications. Its ability to identify correspondences between data points underpins numerous applications across diverse industries. By understanding different matching techniques and addressing associated challenges, organizations can leverage this powerful tool to optimize processes, improve decision-making, and drive innovation.
Exploring the Connection Between Data Quality and Matching Accuracy
Data quality plays a pivotal role in the accuracy and effectiveness of matching processes. Poor data quality can lead to inaccurate matches, missed opportunities, and ultimately, compromised decision-making. The relationship between data quality and matching accuracy is inextricably linked.
Key Factors to Consider:
Roles and Real-World Examples:
-
Incomplete Data: Missing values in key attributes can prevent accurate matching. For instance, missing addresses in customer records can impede accurate geolocation matching for targeted advertising.
-
Inconsistent Data: Variations in data formats or naming conventions (e.g., different spellings of a customer’s name) can lead to false negatives (missed matches).
-
Erroneous Data: Incorrect or outdated information can result in false positives (incorrect matches). For example, an incorrect date of birth can lead to mismatched medical records.
Risks and Mitigations:
-
Risk of Inaccurate Insights: Poor data quality can lead to flawed analyses and incorrect conclusions based on inaccurate matches.
-
Risk of Misallocation of Resources: Inaccurate matching can lead to inefficient resource allocation, wasted time, and financial losses.
-
Risk of Reputational Damage: Inaccurate matching, especially in sensitive areas like healthcare or finance, can severely damage an organization's reputation.
Mitigations:
-
Data Cleansing: Implement rigorous data cleansing processes to identify and correct errors, inconsistencies, and missing values.
-
Data Standardization: Standardize data formats and naming conventions to improve data consistency.
-
Data Validation: Implement data validation rules to ensure data accuracy and integrity.
-
Data Quality Monitoring: Regularly monitor data quality metrics to identify and address potential issues proactively.
Impact and Implications:
The impact of data quality on matching accuracy extends far beyond the immediate matching process. It affects the overall reliability of downstream analyses, decision-making, and business outcomes. High-quality data is essential for building robust and reliable matching systems that can deliver accurate and actionable insights.
Conclusion: Reinforcing the Connection
The relationship between data quality and matching accuracy is paramount. Investing in data quality management is a critical step in ensuring the accuracy and reliability of matching processes, maximizing the benefits of this powerful tool. Organizations must prioritize data quality to unlock the full potential of matching and avoid the risks associated with poor data.
Further Analysis: Examining Data Preprocessing in Greater Detail
Data preprocessing is a crucial step in preparing data for matching. It involves a series of techniques to transform raw data into a format suitable for matching algorithms. This includes data cleaning, transformation, and reduction.
FAQ Section: Answering Common Questions About Matching Concepts
Q: What is the difference between exact and fuzzy matching?
A: Exact matching requires perfect matches between data points, while fuzzy matching allows for some variations or inconsistencies.
Q: What are some common challenges in implementing matching techniques?
A: Common challenges include data quality issues, scalability, computational complexity, and privacy concerns.
Q: How can organizations ensure the accuracy of matching processes?
A: Organizations can ensure accuracy through rigorous data quality management, the selection of appropriate matching algorithms, and thorough validation of matching results.
Practical Tips: Maximizing the Benefits of Matching
-
Assess Data Quality: Before starting any matching project, thoroughly assess the quality of your data.
-
Choose the Right Algorithm: Select the matching algorithm that best suits your data and objectives.
-
Implement Data Preprocessing: Clean, transform, and reduce your data to improve matching accuracy.
-
Validate Results: Validate the results of your matching process to ensure accuracy and reliability.
-
Monitor Performance: Regularly monitor the performance of your matching system to identify and address potential issues.
Final Conclusion: Wrapping Up with Lasting Insights
Matching concepts are fundamental to many aspects of modern data analysis and decision-making. Understanding the different types of matching, associated challenges, and the crucial role of data quality empowers organizations to leverage this powerful tool for improved efficiency, innovation, and enhanced business outcomes. By embracing best practices and proactively addressing potential challenges, organizations can unlock the full potential of matching and gain a competitive edge in today's data-driven world.
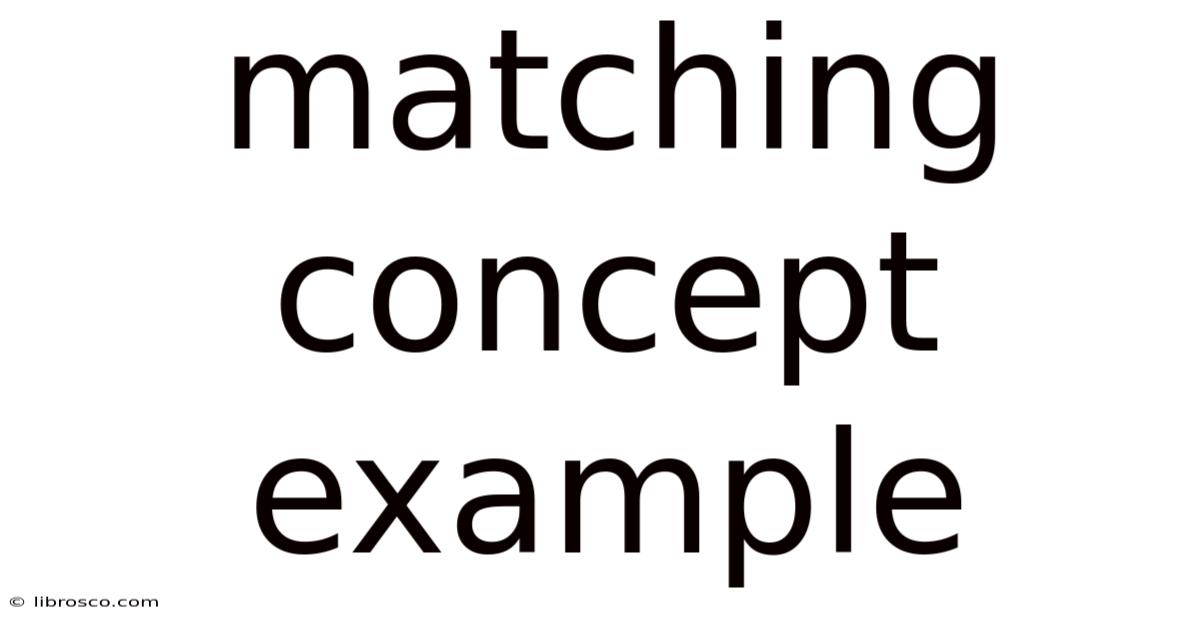
Thank you for visiting our website wich cover about Matching Concept Example. We hope the information provided has been useful to you. Feel free to contact us if you have any questions or need further assistance. See you next time and dont miss to bookmark.
Also read the following articles
Article Title | Date |
---|---|
Cash Management Services Companies In India | Apr 23, 2025 |
Matching Concept Is A Accrual Concept | Apr 23, 2025 |
Factors Influencing Business Risk | Apr 23, 2025 |
Can You Have More Than One Payday Loan | Apr 23, 2025 |
Million Barrel Of Oil Equivalent | Apr 23, 2025 |