How To Measure Credit Risk
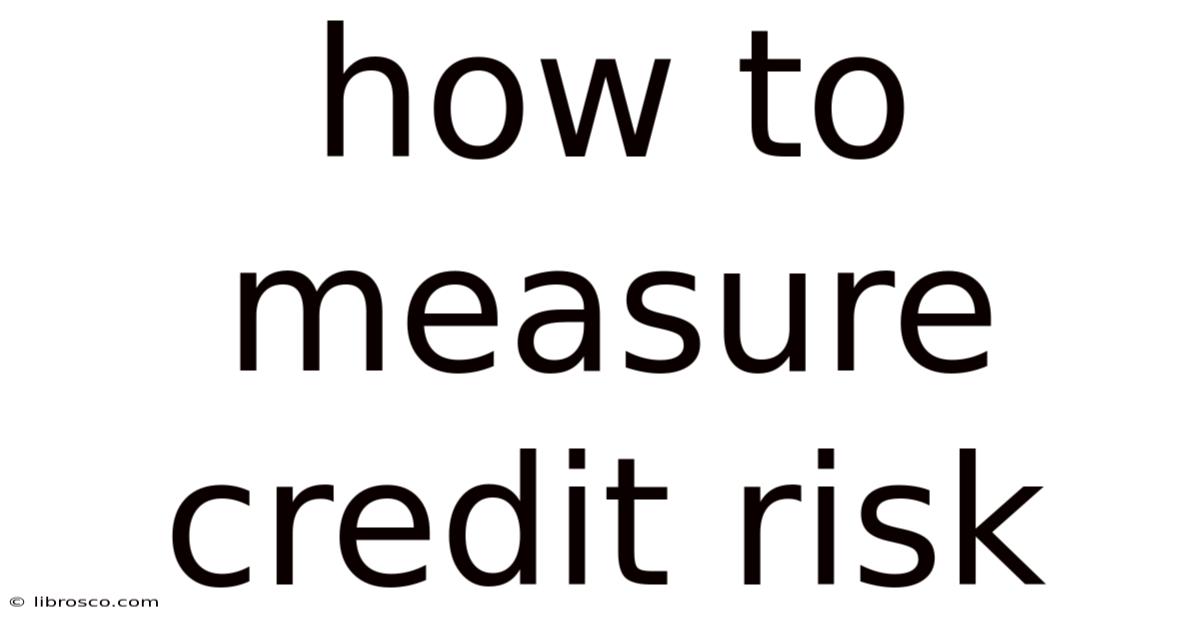
Discover more detailed and exciting information on our website. Click the link below to start your adventure: Visit Best Website meltwatermedia.ca. Don't miss out!
Table of Contents
How to Measure Credit Risk: A Comprehensive Guide
What if the future of lending hinges on accurately measuring credit risk? Mastering credit risk assessment is no longer a luxury; it's a necessity for survival in the modern financial landscape.
Editor's Note: This article on measuring credit risk was published today, providing you with the latest insights and methodologies used by financial institutions and businesses worldwide. It covers various approaches, highlighting both traditional and modern techniques for a comprehensive understanding.
Why Measuring Credit Risk Matters:
Credit risk, the potential for financial loss due to a borrower's failure to repay a debt, is a pervasive concern across numerous sectors. From banks and financial institutions to businesses extending credit to customers, understanding and managing this risk is crucial for maintaining financial health and profitability. The consequences of inadequate credit risk assessment can be severe, ranging from loan defaults and bankruptcies to systemic financial crises. Effective measurement helps organizations make informed lending decisions, optimize pricing strategies, allocate capital effectively, and comply with regulatory requirements. Furthermore, robust credit risk management fosters trust and stability within the financial ecosystem.
Overview: What This Article Covers
This in-depth article explores the multifaceted nature of credit risk measurement. It begins by defining core concepts and delves into traditional methods such as credit scoring and financial ratio analysis. Subsequently, it examines more advanced techniques, including statistical modeling, machine learning, and the integration of alternative data sources. The article also addresses the challenges and limitations of each method, emphasizing the importance of a holistic and adaptable approach. Finally, it provides practical recommendations for implementing effective credit risk measurement strategies.
The Research and Effort Behind the Insights
This article is the result of extensive research, drawing upon academic literature, industry reports, regulatory guidelines, and practical experience in credit risk management. The information presented reflects widely accepted best practices and incorporates insights from leading experts in the field. Every claim is substantiated by evidence to ensure accuracy and reliability.
Key Takeaways:
- Definition and Core Concepts: A clear understanding of credit risk, its different types, and the factors that influence it.
- Traditional Methods: A detailed examination of credit scoring, financial ratio analysis, and other established techniques.
- Advanced Techniques: An exploration of statistical modeling, machine learning, and the use of alternative data in credit risk assessment.
- Challenges and Limitations: A critical analysis of the weaknesses and potential biases inherent in various methods.
- Practical Applications: Actionable insights and best practices for implementing effective credit risk management strategies.
- Regulatory Considerations: An overview of relevant regulations and compliance requirements.
Smooth Transition to the Core Discussion:
With a foundational understanding of the importance of credit risk measurement, let's delve into the specific methods and techniques employed to quantify and manage this inherent risk.
Exploring the Key Aspects of Credit Risk Measurement
1. Definition and Core Concepts:
Credit risk encompasses the possibility that a borrower will fail to meet its obligations, resulting in a financial loss for the lender. This loss can manifest in various forms, including principal loss, interest loss, and administrative costs associated with recovery efforts. Credit risk is inherently probabilistic; it cannot be eliminated entirely but can be mitigated through effective measurement and management. Several factors influence credit risk, including macroeconomic conditions, industry trends, borrower-specific characteristics (financial health, credit history, management quality), and the terms of the loan agreement itself.
2. Traditional Methods:
-
Credit Scoring: This widely used method assigns a numerical score to a borrower based on their credit history and other relevant factors. Scores are typically generated using statistical models that incorporate variables such as payment history, amounts owed, length of credit history, new credit, and credit mix. Higher scores indicate a lower probability of default. Credit bureaus like Experian, Equifax, and TransUnion play a critical role in providing the underlying data for credit scoring.
-
Financial Ratio Analysis: This involves analyzing a borrower's financial statements (balance sheet, income statement, cash flow statement) to assess their financial health and solvency. Key ratios include liquidity ratios (current ratio, quick ratio), profitability ratios (gross profit margin, net profit margin), leverage ratios (debt-to-equity ratio, debt service coverage ratio), and activity ratios (inventory turnover, receivables turnover). Unusual trends or ratios outside of acceptable benchmarks can signal heightened credit risk.
-
Qualitative Assessment: While quantitative methods provide valuable data, qualitative factors are equally crucial. These include management quality, industry outlook, competitive landscape, and the borrower's overall business strategy. Experienced credit analysts often use qualitative assessments to complement quantitative data and gain a holistic perspective.
3. Advanced Techniques:
-
Statistical Modeling: More sophisticated models such as logistic regression, probit models, and survival analysis can predict the probability of default more accurately than simpler credit scoring methods. These models use historical data to estimate the relationship between various factors and default events.
-
Machine Learning: Machine learning algorithms, such as neural networks, support vector machines, and random forests, can analyze vast datasets and identify complex patterns that traditional methods might miss. These techniques are particularly effective in handling non-linear relationships and incorporating alternative data sources.
-
Alternative Data: The rise of big data has expanded the range of information available for credit risk assessment. Alternative data sources, including social media activity, online transaction history, and mobile phone usage patterns, can provide insights into borrower behavior and creditworthiness that traditional methods might overlook.
4. Challenges and Limitations:
-
Data Quality and Availability: Accurate and reliable data is the cornerstone of any credit risk measurement system. Inaccurate or incomplete data can lead to biased results and flawed predictions. Data availability can also be a significant challenge, particularly for smaller businesses or borrowers with limited credit history.
-
Model Risk: Sophisticated statistical models and machine learning algorithms, while powerful, can be susceptible to model risk. This refers to the potential for errors or biases in the model's specification, estimation, or implementation. Regular model validation and monitoring are crucial to mitigate model risk.
-
Economic Cycles: Credit risk models are often developed and calibrated using historical data. However, economic cycles can significantly impact default rates. Models trained on data from a period of economic expansion may not perform well during a recession.
-
Regulatory Changes: The regulatory landscape for credit risk management is constantly evolving. Organizations must adapt their measurement techniques and processes to comply with new regulations and requirements.
Exploring the Connection Between Data Quality and Credit Risk Measurement
The relationship between data quality and credit risk measurement is fundamental. Accurate, complete, and timely data is crucial for developing reliable models and making informed lending decisions. Poor data quality can lead to inaccurate risk assessments, potentially resulting in significant financial losses.
Key Factors to Consider:
-
Roles and Real-World Examples: Consider the case of a bank using a credit scoring model based on flawed data. Incorrect or missing information about a borrower's payment history could result in an inaccurate credit score, leading to either the rejection of a creditworthy applicant or the approval of a high-risk borrower.
-
Risks and Mitigations: The risks associated with poor data quality include inaccurate risk assessments, incorrect lending decisions, and increased loan defaults. Mitigation strategies include implementing robust data validation and cleansing processes, regularly auditing data sources, and investing in data management infrastructure.
-
Impact and Implications: The impact of poor data quality can extend beyond individual lending decisions. It can affect a financial institution's overall profitability, regulatory compliance, and reputation.
Conclusion: Reinforcing the Connection
The interplay between data quality and credit risk measurement underscores the critical need for robust data management practices. By addressing challenges and leveraging opportunities related to data quality, financial institutions can improve the accuracy of their risk assessments, enhance their lending decisions, and ultimately enhance their financial performance.
Further Analysis: Examining Data Governance in Greater Detail
Effective data governance is essential for maintaining data quality. This involves establishing clear policies and procedures for data collection, storage, processing, and access. Implementing data governance frameworks helps ensure the accuracy, completeness, and reliability of data used in credit risk measurement. Key aspects of data governance include data quality management, data security, data privacy, and data lineage.
FAQ Section: Answering Common Questions About Credit Risk Measurement
-
What is credit risk? Credit risk is the potential for financial loss resulting from a borrower's failure to repay a debt.
-
How is credit risk measured? Credit risk is measured using a variety of techniques, ranging from simple credit scoring to sophisticated statistical models and machine learning algorithms.
-
What are the key factors influencing credit risk? Factors influencing credit risk include macroeconomic conditions, industry trends, borrower-specific characteristics, and loan terms.
-
What are the challenges in measuring credit risk? Challenges include data quality issues, model risk, economic cycles, and regulatory changes.
Practical Tips: Maximizing the Benefits of Effective Credit Risk Measurement
-
Invest in Data Quality: Prioritize data accuracy, completeness, and timeliness. Implement robust data validation and cleansing processes.
-
Employ Diverse Measurement Techniques: Use a combination of traditional and advanced methods to gain a holistic view of credit risk.
-
Regularly Validate and Monitor Models: Ensure that your credit risk models remain accurate and effective over time.
-
Stay Informed About Regulatory Changes: Keep abreast of new regulations and adapt your processes accordingly.
-
Integrate Qualitative and Quantitative Assessments: Combine quantitative data with qualitative insights to enhance the accuracy of your risk assessments.
Final Conclusion: Wrapping Up with Lasting Insights
Effective credit risk measurement is not merely a compliance requirement; it's a strategic imperative for organizations that extend credit. By implementing robust measurement techniques and prioritizing data quality, businesses and financial institutions can mitigate potential losses, optimize lending strategies, and enhance their overall financial performance. The dynamic nature of the financial landscape necessitates ongoing adaptation and refinement of credit risk management strategies, ensuring resilience and success in the face of evolving challenges. Continuous improvement, incorporating new technologies and data sources, and a commitment to data integrity are key to maintaining a strong and sustainable credit risk management framework.
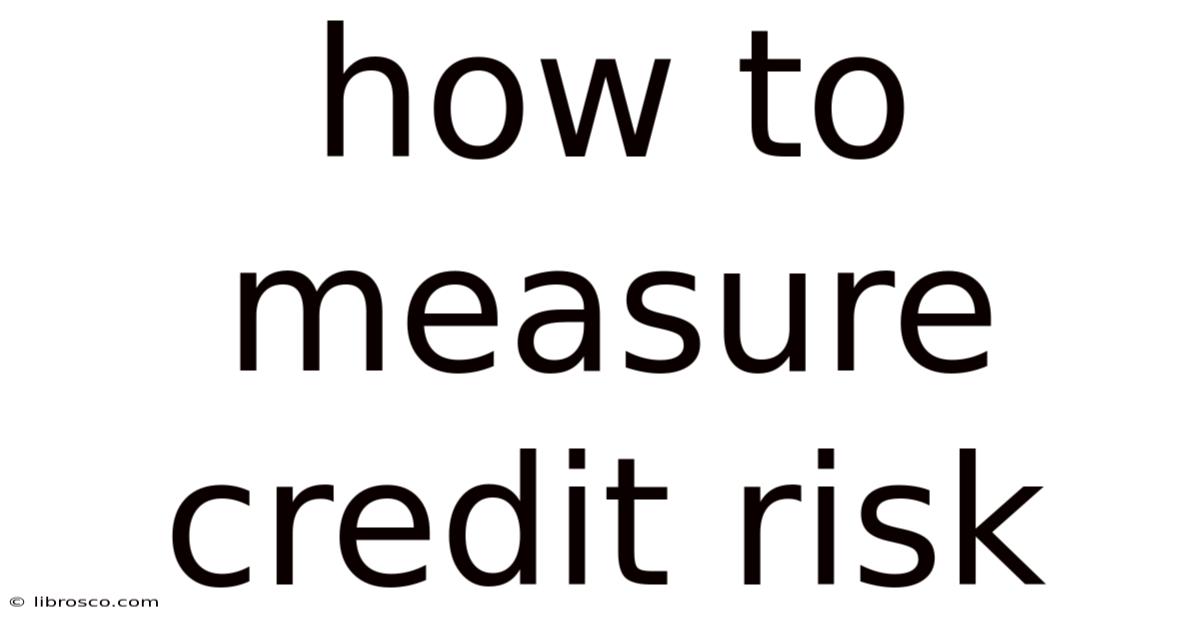
Thank you for visiting our website wich cover about How To Measure Credit Risk. We hope the information provided has been useful to you. Feel free to contact us if you have any questions or need further assistance. See you next time and dont miss to bookmark.
Also read the following articles
Article Title | Date |
---|---|
Origination Fee Definition Average Cost And Ways To Save | Mar 09, 2025 |
What Credit Bureau Does Andrews Federal Credit Union Use | Mar 09, 2025 |
How Do You Get An Eviction Off Your Credit | Mar 09, 2025 |
How Long Till Credit Inquiries Fall Off | Mar 09, 2025 |
When Does Epd Pay Dividends | Mar 09, 2025 |