Expected Value Definition Formula And Examples
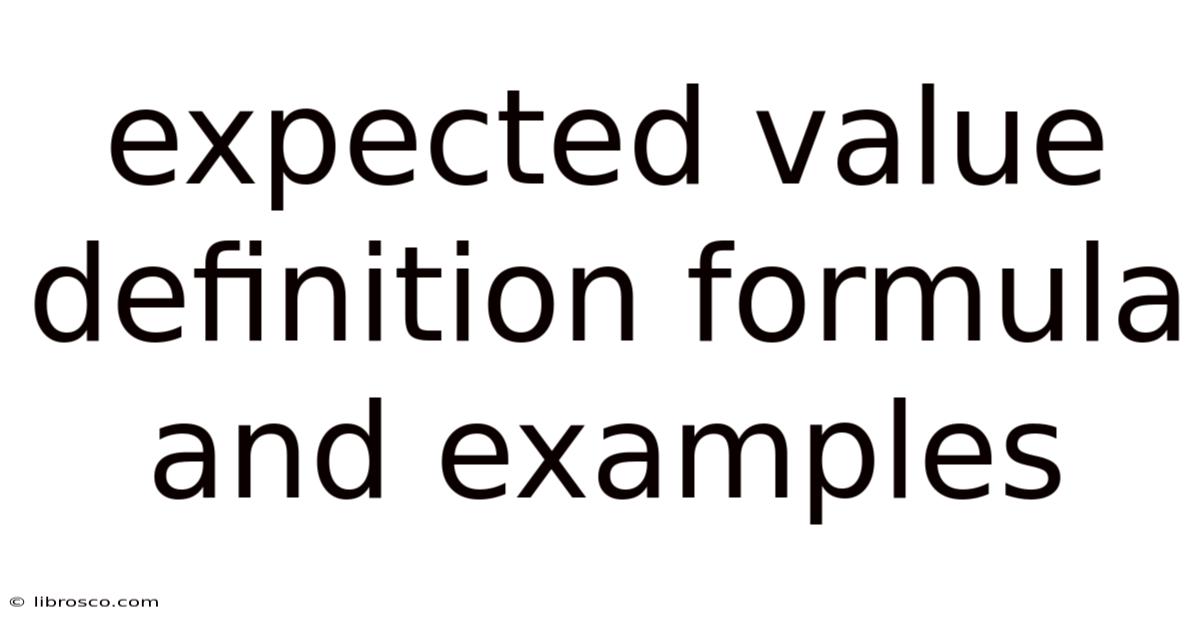
Discover more detailed and exciting information on our website. Click the link below to start your adventure: Visit Best Website meltwatermedia.ca. Don't miss out!
Table of Contents
Unlocking the Power of Expected Value: Definition, Formula, and Real-World Applications
What if making optimal decisions consistently relied on a single, powerful concept? Expected value, a cornerstone of decision-making under uncertainty, provides exactly that.
Editor’s Note: This article on expected value provides a comprehensive overview of the concept, including its definition, formula, various applications, and nuanced considerations. It aims to equip readers with a practical understanding of this crucial tool for informed decision-making.
Why Expected Value Matters:
Expected value (EV) is a fundamental concept in probability and statistics with far-reaching applications across diverse fields. From finance and gambling to healthcare and artificial intelligence, understanding expected value allows for the quantification of risk and the optimization of decision-making processes in situations with uncertain outcomes. Its practical implications are vast, influencing everything from investment strategies to medical treatments and even everyday choices. The ability to calculate and interpret expected value empowers individuals and organizations to make more informed, rational decisions in the face of uncertainty.
Overview: What This Article Covers:
This article will provide a detailed explanation of expected value, beginning with its core definition and formula. We will explore diverse real-world examples illustrating its application in different scenarios, including simple games of chance, complex investment decisions, and strategic planning. We’ll also examine limitations and considerations to ensure a comprehensive understanding of this powerful tool. The article will delve into the calculation of expected value in scenarios with both discrete and continuous probability distributions. Finally, we will explore advanced applications and related concepts.
The Research and Effort Behind the Insights:
This article draws upon established principles of probability theory and statistics, referencing standard textbooks and academic papers. Numerous examples are provided to illustrate the concepts clearly and practically. The explanations aim for clarity and accessibility, avoiding overly technical jargon wherever possible.
Key Takeaways:
- Definition and Core Concepts: A precise definition of expected value and its underlying principles.
- Formula and Calculation: A step-by-step guide to calculating expected value in various scenarios.
- Discrete vs. Continuous Distributions: Understanding the calculation differences for different types of probability distributions.
- Real-World Applications: Diverse examples illustrating the use of expected value in various fields.
- Limitations and Considerations: Acknowledging the situations where the expected value approach may not be sufficient.
- Advanced Applications: Exploring more complex applications and related concepts.
Smooth Transition to the Core Discussion:
With a foundational understanding of the importance of expected value, let’s now delve into its precise definition and the mechanics of its calculation.
Exploring the Key Aspects of Expected Value:
1. Definition and Core Concepts:
Expected value represents the average outcome one can expect over many repetitions of a random process. It's a weighted average, where each possible outcome is weighted by its probability of occurrence. In simpler terms, it's the long-run average value of a random variable.
2. Formula and Calculation:
The formula for expected value depends on whether the random variable is discrete or continuous.
-
Discrete Random Variable: For a discrete random variable X with possible outcomes x₁, x₂, ..., xₙ and corresponding probabilities p₁, p₂, ..., pₙ, the expected value is calculated as:
E(X) = x₁p₁ + x₂p₂ + ... + xₙpₙ = Σ [xᵢ * pᵢ] (where Σ denotes summation)
-
Continuous Random Variable: For a continuous random variable X with probability density function f(x), the expected value is calculated as:
E(X) = ∫ xf(x)dx (where ∫ denotes integration over the entire range of X)
3. Examples Illustrating Expected Value Calculations:
Example 1: A Simple Dice Game
Consider a game where you roll a fair six-sided die. If you roll a 6, you win $10; otherwise, you win nothing. What is the expected value of your winnings?
- Possible outcomes (xᵢ): $10, $0
- Probabilities (pᵢ): 1/6, 5/6
E(X) = ($10)(1/6) + ($0)(5/6) = $1.67
The expected value of your winnings is $1.67. This doesn't mean you'll win exactly $1.67 each time you play, but over many games, your average winnings should approach this value.
Example 2: Investment Decision
An investor is considering two investment options:
- Option A: A high-risk investment with a 60% chance of a $100,000 profit and a 40% chance of a $50,000 loss.
- Option B: A low-risk investment with a 90% chance of a $20,000 profit and a 10% chance of a $5,000 loss.
Let's calculate the expected value for each option:
Option A: E(A) = ($100,000)(0.6) + (-$50,000)(0.4) = $40,000
Option B: E(B) = ($20,000)(0.9) + (-$5,000)(0.1) = $17,500
Based on expected value, Option A is preferable, despite its higher risk.
4. Discrete vs. Continuous Distributions:
The key difference lies in the calculation method. Discrete distributions involve summing weighted outcomes, while continuous distributions require integration. The choice depends on the nature of the random variable. For example, the number of heads in a coin toss is a discrete variable, while the height of a randomly selected person is a continuous variable.
5. Real-World Applications:
Expected value finds applications in numerous fields:
- Finance: Evaluating investment opportunities, pricing options, and managing risk.
- Insurance: Calculating premiums based on expected payouts.
- Gambling: Assessing the fairness of games and developing optimal betting strategies.
- Healthcare: Evaluating the effectiveness of treatments and making decisions under uncertainty.
- Artificial Intelligence: Developing decision-making algorithms for autonomous systems.
- Supply Chain Management: Predicting demand, optimizing inventory levels, and mitigating risks.
6. Limitations and Considerations:
While expected value is a powerful tool, it has limitations:
- Risk Aversion: Expected value doesn't account for individual risk preferences. Some individuals might prefer a less risky option with a lower expected value.
- Non-linear Payoffs: Expected value calculations are most accurate for linear relationships between outcomes and payoffs. In situations with non-linear payoffs, additional considerations are necessary.
- Limited Information: Accurate expected value calculation depends on having reliable probability estimates. Inaccurate or incomplete information can lead to misleading results.
Exploring the Connection Between Risk Aversion and Expected Value:
Risk aversion refers to the preference of individuals to avoid uncertainty and risk, even if it means accepting a lower expected value. A risk-averse investor might prefer Option B (the low-risk investment) in the previous example, even though Option A has a higher expected value. This highlights that expected value is not the only factor influencing real-world decision-making. Utility theory, which incorporates risk preferences into the decision-making framework, extends the application of expected value.
Key Factors to Consider:
- Roles and Real-World Examples: How risk aversion manifests in real-world investment and insurance decisions is crucial to understand. For example, insurance companies price premiums higher than the pure expected value of payouts to account for their own risk aversion.
- Risks and Mitigations: Risk-averse decision-makers can mitigate risk by diversifying investments or employing strategies like hedging.
- Impact and Implications: Understanding risk aversion improves predictions of economic behavior and allows for the development of tailored risk management strategies.
Conclusion: Reinforcing the Connection:
The interplay between risk aversion and expected value underscores the complexity of decision-making under uncertainty. While expected value provides a valuable framework, incorporating individual preferences for risk is crucial for practical applications.
Further Analysis: Examining Risk Aversion in Greater Detail:
Risk aversion can be formally modeled using utility functions, which quantify the subjective value or satisfaction associated with different levels of wealth or outcomes. These utility functions are often concave, reflecting the diminishing marginal utility of wealth; the additional happiness derived from an extra dollar decreases as one's wealth increases. This explains why a risk-averse person might choose a certain, smaller gain over a gamble with a higher expected value but also higher risk.
FAQ Section: Answering Common Questions About Expected Value:
- What is expected value? Expected value is the average outcome you'd expect over many repetitions of a random event.
- How is expected value calculated? The formula differs slightly depending on whether the random variable is discrete or continuous. For discrete variables, it's the sum of each outcome multiplied by its probability. For continuous variables, it involves integration.
- Why is expected value important? It provides a quantitative framework for decision-making under uncertainty across numerous fields, from finance to healthcare.
- What are the limitations of expected value? It doesn't always account for risk aversion or non-linear payoffs, and its accuracy depends on the reliability of probability estimates.
Practical Tips: Maximizing the Benefits of Expected Value:
- Clearly Define Outcomes and Probabilities: Before calculating expected value, ensure you have clearly identified all possible outcomes and their associated probabilities.
- Choose the Right Formula: Use the appropriate formula for discrete or continuous random variables.
- Consider Risk Aversion: Don't solely rely on expected value if risk aversion is a significant factor in the decision.
- Refine Probability Estimates: Strive to obtain the most accurate probability estimates possible, as inaccurate probabilities lead to inaccurate expected values.
Final Conclusion: Wrapping Up with Lasting Insights:
Expected value serves as a powerful tool for making informed decisions in the face of uncertainty. By understanding its definition, formula, applications, and limitations, individuals and organizations can leverage its capabilities to optimize strategies across diverse domains. While it is not a panacea, its inclusion within a broader decision-making framework that accounts for individual risk preferences greatly enhances its practical value. Mastering expected value provides a significant advantage in navigating the complexities of an uncertain world.
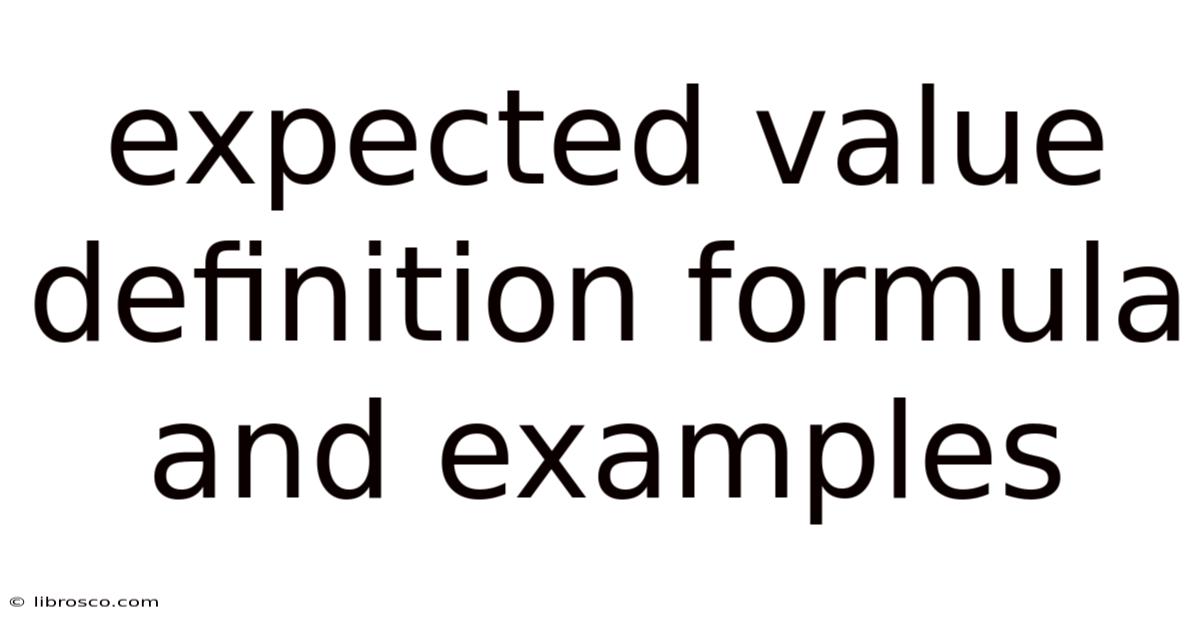
Thank you for visiting our website wich cover about Expected Value Definition Formula And Examples. We hope the information provided has been useful to you. Feel free to contact us if you have any questions or need further assistance. See you next time and dont miss to bookmark.
Also read the following articles
Article Title | Date |
---|---|
Exchange Of Futures For Physical Efp Definition And Example | Apr 11, 2025 |
Factor Market Definition Types And Examples | Apr 11, 2025 |
Export Incentives Definition Types And Benefits | Apr 11, 2025 |
How Much Is A No Insurance Ticket In California | Apr 11, 2025 |
What Was The European Community Ec Definition And History | Apr 11, 2025 |