Excess Kurtosis Definition Types Example
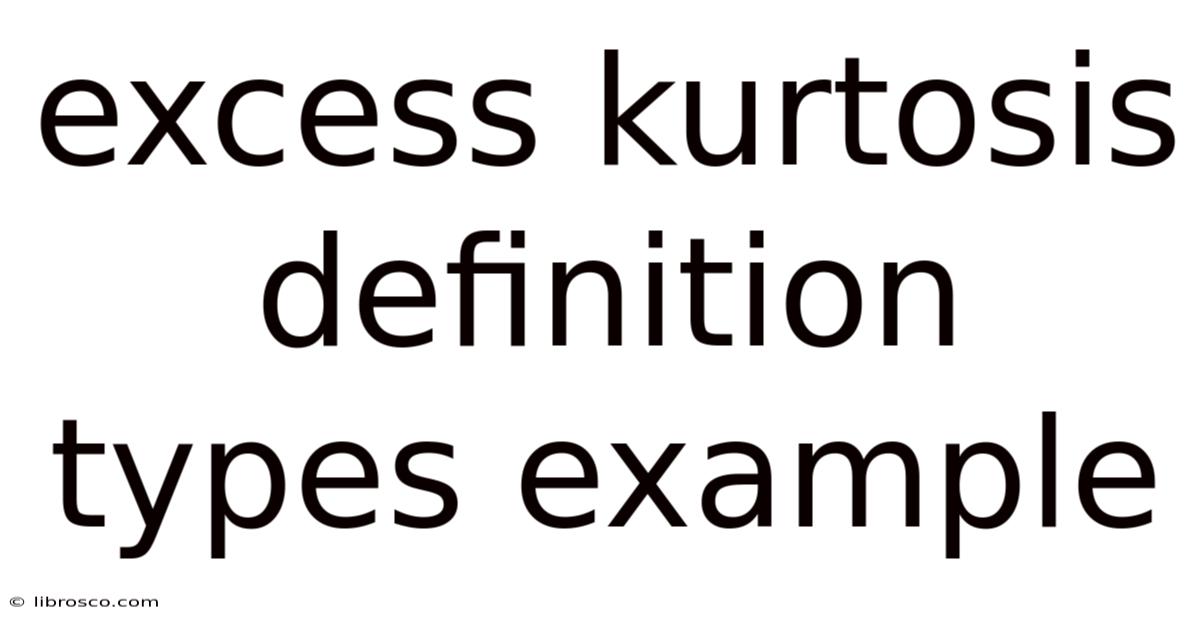
Discover more detailed and exciting information on our website. Click the link below to start your adventure: Visit Best Website meltwatermedia.ca. Don't miss out!
Table of Contents
Unpacking Excess Kurtosis: Definition, Types, and Examples
What if understanding excess kurtosis unlocks a deeper understanding of data distribution and risk assessment? This crucial statistical concept reveals hidden patterns and informs more accurate predictions across diverse fields.
Editor’s Note: This article on excess kurtosis provides a comprehensive overview of its definition, types, practical applications, and interpretation. Readers will gain a solid understanding of this important statistical measure and its implications for data analysis and modeling. This updated information is relevant for students, researchers, and professionals working with data across various disciplines.
Why Excess Kurtosis Matters:
Excess kurtosis is a critical statistical measure that describes the "tailedness" and "peakedness" of a probability distribution compared to a normal distribution. Unlike simply looking at the mean and standard deviation, kurtosis offers insights into the presence of outliers and the potential for extreme values. Its applications are far-reaching, impacting fields like finance (risk management), engineering (quality control), and even social sciences (analyzing survey data). Understanding excess kurtosis allows for more accurate modeling, improved risk assessment, and a more nuanced interpretation of data. It helps identify datasets that deviate significantly from the familiar bell curve, leading to better informed decisions.
Overview: What This Article Covers:
This article will provide a thorough exploration of excess kurtosis. We will define kurtosis and excess kurtosis, distinguishing between leptokurtic, mesokurtic, and platykurtic distributions. We will delve into the mathematical calculation of excess kurtosis and illustrate its application with real-world examples from various fields. Finally, we will discuss the limitations of relying solely on excess kurtosis for data analysis.
The Research and Effort Behind the Insights:
This article synthesizes information from established statistical textbooks, peer-reviewed research papers, and reputable online resources. The explanations and examples provided are based on widely accepted statistical principles and methodologies. The goal is to present a clear, accurate, and accessible understanding of excess kurtosis for a broad audience.
Key Takeaways:
- Definition and Core Concepts: A precise definition of kurtosis and excess kurtosis, along with the mathematical formulas.
- Types of Kurtosis: A clear explanation of leptokurtic, mesokurtic, and platykurtic distributions and their visual representations.
- Practical Applications: Real-world examples demonstrating the use of excess kurtosis in different fields.
- Limitations and Considerations: A discussion of the limitations of using excess kurtosis in isolation and the importance of considering other statistical measures.
Smooth Transition to the Core Discussion:
Having established the importance and scope of this article, let's now delve into the core concepts of excess kurtosis, beginning with its precise definition.
Exploring the Key Aspects of Excess Kurtosis:
1. Definition and Core Concepts:
Kurtosis measures the "tailedness" and "peakedness" of a probability distribution. A high kurtosis indicates a sharper peak and heavier tails, while a low kurtosis suggests a flatter peak and lighter tails. However, the raw kurtosis value is often difficult to interpret directly. This is where excess kurtosis comes into play.
Excess kurtosis is simply the kurtosis of a distribution minus 3. The subtraction of 3 is a normalization that sets the kurtosis of a normal distribution to 0. Therefore, excess kurtosis provides a more intuitive measure, allowing for easier comparison across different distributions.
Mathematically, the excess kurtosis (γ<sub>2</sub>) is calculated as:
γ<sub>2</sub> = μ<sub>4</sub> / σ<sup>4</sup> - 3
where:
- μ<sub>4</sub> is the fourth central moment of the distribution.
- σ is the standard deviation of the distribution.
2. Types of Kurtosis:
Based on the value of excess kurtosis, distributions are categorized as follows:
-
Mesokurtic (γ<sub>2</sub> = 0): A mesokurtic distribution has a kurtosis similar to a normal distribution. Its peak is neither too sharp nor too flat, and its tails are neither too heavy nor too light.
-
Leptokurtic (γ<sub>2</sub> > 0): A leptokurtic distribution is characterized by a sharper peak and heavier tails than a normal distribution. This means there's a higher concentration of data points around the mean and a greater probability of extreme values. Leptokurtic distributions are often associated with higher risk in financial applications, as they indicate a higher likelihood of extreme events.
-
Platykurtic (γ<sub>2</sub> < 0): A platykurtic distribution has a flatter peak and lighter tails than a normal distribution. It suggests a more uniform distribution of data points, with fewer extreme values.
3. Applications Across Industries:
Excess kurtosis finds applications across numerous fields:
-
Finance: In risk management, excess kurtosis is a crucial indicator of potential extreme events (e.g., market crashes). A high excess kurtosis in asset returns suggests a higher risk of large losses or gains.
-
Engineering: In quality control, excess kurtosis can help identify manufacturing defects or variations in product characteristics. A leptokurtic distribution might indicate that a significant portion of products fall outside the acceptable range.
-
Healthcare: Analyzing medical data, excess kurtosis can help identify outliers or unusual patterns that may require further investigation. For example, it might help detect unusual blood pressure readings or other vital signs.
-
Image Processing: Excess kurtosis can be used in image analysis to identify regions of high contrast or texture.
-
Social Sciences: In analyzing survey data, excess kurtosis can reveal the presence of extreme opinions or responses that may skew the overall results.
4. Impact on Innovation:
Understanding excess kurtosis has spurred innovation in areas like robust statistics, which focuses on developing statistical methods that are less sensitive to outliers. This is particularly crucial when dealing with datasets that may contain errors or extreme values. Moreover, the use of excess kurtosis in risk management has driven the development of more sophisticated risk models that better account for the potential of extreme events.
Closing Insights: Summarizing the Core Discussion:
Excess kurtosis is a powerful tool for understanding the shape of a distribution beyond simply looking at its mean and standard deviation. By identifying leptokurtic, mesokurtic, or platykurtic distributions, analysts gain valuable insights into the potential for extreme values and the overall risk associated with the data. Its applications extend across numerous fields, contributing to improved decision-making and risk management strategies.
Exploring the Connection Between Sample Size and Excess Kurtosis:
The accuracy of excess kurtosis estimates is heavily influenced by the sample size. With small sample sizes, the estimated excess kurtosis can be highly variable and unreliable. Larger samples provide more stable and accurate estimates. This is because the fourth central moment, a key component in calculating kurtosis, is more sensitive to outliers and sampling variability compared to lower-order moments like the mean and variance.
Key Factors to Consider:
-
Roles and Real-World Examples: In financial modeling, small sample sizes (e.g., short time series of stock returns) can lead to unreliable kurtosis estimates, potentially underestimating or overestimating the risk of extreme events.
-
Risks and Mitigations: The risk of inaccurate excess kurtosis estimations due to small sample size can be mitigated by using larger datasets whenever possible. Alternatively, robust estimation methods less sensitive to outliers can be employed.
-
Impact and Implications: Inaccurate excess kurtosis estimations can lead to flawed conclusions in various applications. For example, in quality control, it might lead to incorrect acceptance or rejection of products based on misleading assessments of variability.
Conclusion: Reinforcing the Connection:
The relationship between sample size and excess kurtosis highlights the importance of considering sample size when interpreting kurtosis values. Researchers and analysts must be aware of this limitation and take appropriate steps to mitigate the risks associated with small sample sizes.
Further Analysis: Examining Sample Size Effects in Greater Detail:
The impact of sample size on excess kurtosis is often studied through simulation studies. Researchers generate random samples from known distributions with varying sample sizes and calculate the excess kurtosis for each sample. This allows them to observe the variability in kurtosis estimates across different sample sizes and quantify the bias associated with smaller samples. These studies consistently demonstrate that larger samples are necessary for reliable kurtosis estimation.
FAQ Section: Answering Common Questions About Excess Kurtosis:
-
What is excess kurtosis? Excess kurtosis is a statistical measure that describes the "tailedness" and "peakedness" of a probability distribution compared to a normal distribution. It's calculated as the kurtosis minus 3.
-
How is excess kurtosis calculated? Excess kurtosis (γ<sub>2</sub>) is calculated as: γ<sub>2</sub> = μ<sub>4</sub> / σ<sup>4</sup> - 3, where μ<sub>4</sub> is the fourth central moment and σ is the standard deviation.
-
What does a positive excess kurtosis indicate? A positive excess kurtosis (leptokurtic distribution) indicates a sharper peak and heavier tails than a normal distribution, suggesting a higher probability of extreme values.
-
What does a negative excess kurtosis indicate? A negative excess kurtosis (platykurtic distribution) indicates a flatter peak and lighter tails than a normal distribution, suggesting fewer extreme values.
-
Why is excess kurtosis important? Excess kurtosis is important because it provides insights into the shape of a distribution and helps identify potential outliers or extreme values. This information is crucial for risk assessment, quality control, and many other applications.
Practical Tips: Maximizing the Benefits of Excess Kurtosis:
-
Understand the Basics: Begin by grasping the fundamental concepts of kurtosis and excess kurtosis.
-
Assess Sample Size: Ensure that the sample size is sufficiently large to obtain reliable estimates of excess kurtosis.
-
Consider Other Measures: Don't rely solely on excess kurtosis. Combine it with other descriptive statistics (mean, median, standard deviation) and visualizations (histograms, box plots) for a more complete understanding of the data.
-
Choose Appropriate Methods: Use robust estimation techniques when dealing with datasets that might contain outliers.
-
Interpret Cautiously: Always interpret excess kurtosis in the context of the specific application and data being analyzed.
Final Conclusion: Wrapping Up with Lasting Insights:
Excess kurtosis is a valuable tool for understanding data distribution and risk assessment. However, its interpretation requires careful consideration of sample size and the limitations of using it in isolation. By combining excess kurtosis with other statistical measures and visualizing the data, analysts can make more informed decisions and better understand the characteristics of their data. Its continued application across diverse fields underscores its enduring relevance in modern data analysis.
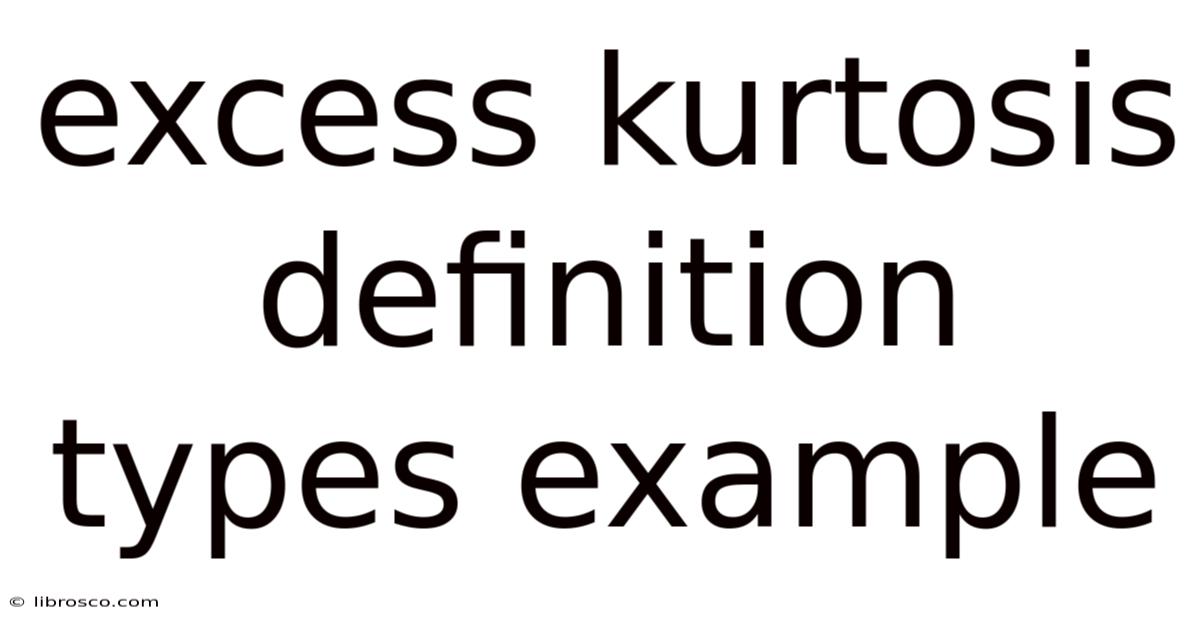
Thank you for visiting our website wich cover about Excess Kurtosis Definition Types Example. We hope the information provided has been useful to you. Feel free to contact us if you have any questions or need further assistance. See you next time and dont miss to bookmark.
Also read the following articles
Article Title | Date |
---|---|
How Much Is Car Insurance In Washington State | Apr 11, 2025 |
How Much Is Latuda With Insurance | Apr 11, 2025 |
Eurozone Definition History Member Countries | Apr 11, 2025 |
How Much Does Malpractice Insurance Cost For Nurse Practitioners | Apr 11, 2025 |
What Is Private Flood Insurance | Apr 11, 2025 |