Endogenous Variable Definition Meaning And Examples
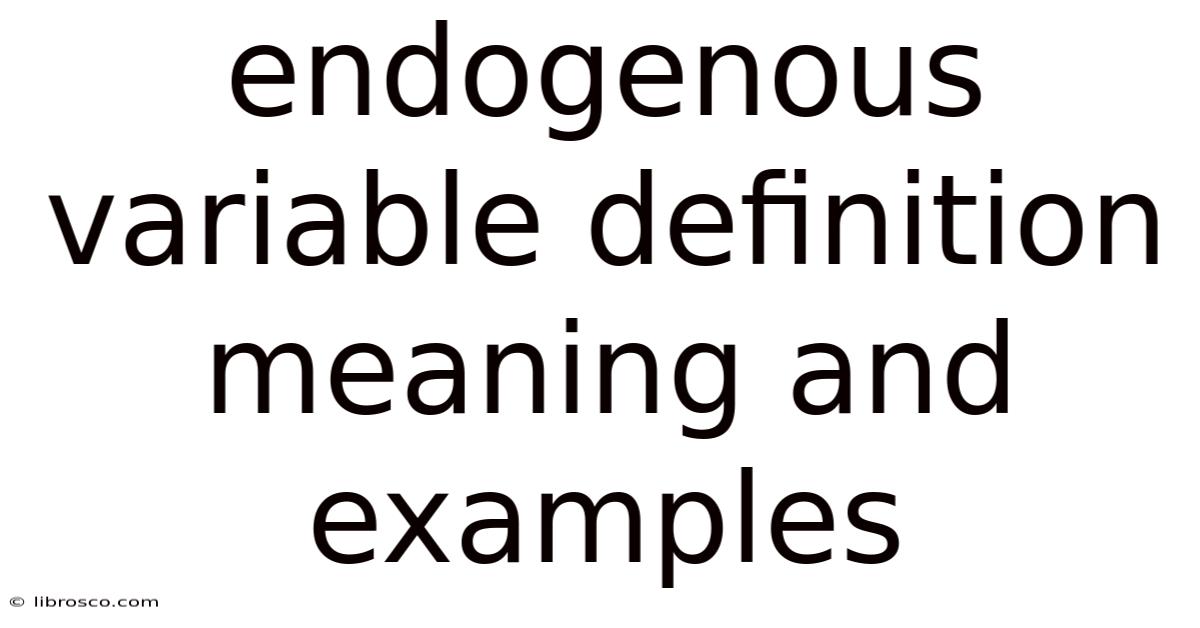
Discover more detailed and exciting information on our website. Click the link below to start your adventure: Visit Best Website meltwatermedia.ca. Don't miss out!
Table of Contents
Unlocking the Secrets of Endogenous Variables: Definition, Meaning, and Examples
What if the very foundations of your economic model rested on a misunderstanding of endogenous variables? A thorough grasp of endogenous variables is crucial for accurate modeling, insightful analysis, and informed decision-making in various fields.
Editor’s Note: This article on endogenous variables provides a comprehensive overview of this crucial concept in econometrics and related fields. Updated with the latest research and examples, it aims to clarify the definition, meaning, and practical applications of endogenous variables for students, researchers, and professionals alike.
Why Endogenous Variables Matter: Relevance, Practical Applications, and Industry Significance
Endogenous variables are the lifeblood of many economic and statistical models. Understanding them is critical for building accurate predictive models and drawing valid inferences from data. Their influence extends across diverse fields, from macroeconomics (analyzing national GDP growth) to microeconomics (examining consumer behavior), and even into areas like marketing (measuring the effectiveness of advertising campaigns) and public health (assessing the impact of policy interventions). Failure to account for endogeneity can lead to biased estimates and flawed conclusions, potentially resulting in misinformed policy decisions or ineffective strategies. The ability to identify and address endogeneity is therefore a crucial skill for anyone working with quantitative data in these areas.
Overview: What This Article Covers
This article provides a thorough exploration of endogenous variables. We will begin by defining endogenous variables and contrasting them with exogenous variables. Then, we will delve into the sources of endogeneity, examining common scenarios where this problem arises. The article will illustrate these concepts with clear examples from different fields, including detailed explanations of how endogeneity biases results and how to mitigate these biases using techniques like instrumental variables. Finally, we’ll address common questions and provide practical tips for identifying and handling endogenous variables in your own research.
The Research and Effort Behind the Insights
This article draws upon a wide range of sources, including leading econometrics textbooks, peer-reviewed journal articles, and reputable online resources. The analysis presented is grounded in established econometric theory and supported by real-world examples to enhance understanding and practical application. A structured approach has been employed to ensure clarity and a logical progression of ideas.
Key Takeaways:
- Definition and Core Concepts: A clear and concise definition of endogenous variables, explaining their key characteristics and differentiating them from exogenous variables.
- Sources of Endogeneity: Identification of common causes of endogeneity, including omitted variables, simultaneity, and measurement error.
- Consequences of Endogeneity: Understanding the biases introduced by endogeneity and their implications for statistical inference.
- Methods to Address Endogeneity: Exploration of techniques like instrumental variables and other econometric methods designed to mitigate endogeneity bias.
- Real-World Applications: Illustrative examples from various fields showcasing the relevance and practical applications of endogenous variable concepts.
Smooth Transition to the Core Discussion
Having established the importance of understanding endogenous variables, let's now delve into their precise definition and the factors that contribute to their endogeneity.
Exploring the Key Aspects of Endogenous Variables
1. Definition and Core Concepts:
An endogenous variable is a variable within a statistical model whose value is determined within the model itself. In simpler terms, it's a variable whose value is influenced by other variables within the system under study. This contrasts sharply with an exogenous variable, whose value is determined outside the model and is considered independent of other variables within the model. The key characteristic of an endogenous variable is its correlation with the error term in the model's equation. This correlation is the source of bias in estimates.
2. Sources of Endogeneity:
Several factors can contribute to the endogeneity of a variable:
-
Omitted Variable Bias: This occurs when a relevant variable that influences both the dependent and independent variables is excluded from the model. The effect of the omitted variable is then absorbed into the error term, causing correlation between the error term and the independent variable.
-
Simultaneity Bias: This arises in models where causality flows in both directions between variables. For example, in a model of supply and demand, price influences quantity demanded, and quantity demanded influences price. Both price and quantity are considered endogenous in this scenario.
-
Measurement Error: If the independent variable is measured with error, the error component will be correlated with the measured independent variable, leading to endogeneity. This is particularly problematic when the measurement error is systematic rather than random.
-
Sample Selection Bias: This occurs when the sample used for analysis isn't representative of the population of interest. For example, if you only analyze data from high-income earners when studying the relationship between income and education, you'll obtain biased results.
3. Consequences of Endogeneity:
Endogeneity leads to inconsistent and biased estimates of the model's parameters. This means that the estimated coefficients will not converge to their true values even with an infinitely large sample size. The direction and magnitude of the bias depend on the specific source of endogeneity and the nature of the relationships between the variables. Consequently, hypothesis testing based on these biased estimates will be unreliable, leading to potentially incorrect conclusions.
4. Impact on Innovation:
Recognizing and addressing endogeneity is crucial for advancing our understanding of complex systems. The development of techniques to handle endogeneity, such as instrumental variables, has significantly improved the accuracy and reliability of econometric models, enabling researchers to make more informed predictions and policy recommendations.
Closing Insights: Summarizing the Core Discussion
Understanding the nature and sources of endogenous variables is paramount for anyone working with quantitative data, especially in economics and related fields. Ignoring endogeneity can lead to flawed analyses and misleading conclusions. The importance of this concept lies in its ability to inform better modeling and ultimately lead to more effective policy decisions and informed strategies.
Exploring the Connection Between Causality and Endogenous Variables
The concept of causality is intrinsically linked to endogenous variables. Endogeneity often arises when there's a violation of the assumption of causality—specifically, when a variable is both a cause and an effect within the system. This creates a feedback loop that makes it challenging to isolate the true effect of one variable on another.
Key Factors to Consider:
-
Roles and Real-World Examples: Consider a model examining the effect of education on income. If unobserved factors like innate ability influence both education level and income, then education becomes endogenous. The effect of education on income is confounded by this unobserved variable.
-
Risks and Mitigations: The primary risk associated with ignoring endogeneity is obtaining biased and inconsistent estimates. This can be mitigated through techniques like instrumental variable regression, which seeks to find variables that are correlated with the endogenous variable but not directly with the error term.
-
Impact and Implications: The impact of endogeneity extends beyond biased estimates. It can lead to inaccurate predictions, flawed policy recommendations, and a compromised understanding of the underlying relationships between variables.
Conclusion: Reinforcing the Connection
The connection between causality and endogenous variables highlights the importance of carefully considering the underlying relationships between variables when building econometric models. Failing to account for endogeneity can lead to serious errors in interpretation and prediction.
Further Analysis: Examining Causality in Greater Detail
Causality is a complex concept. It's essential to distinguish between correlation and causation. While a correlation between two variables may suggest a causal relationship, it does not prove it. Endogeneity often arises when correlation is misinterpreted as causation, leading to flawed inferences. Techniques like Granger causality tests can provide additional insights into the direction of causal relationships, but they cannot definitively establish causality.
FAQ Section: Answering Common Questions About Endogenous Variables
-
What is an endogenous variable? An endogenous variable is a variable whose value is determined within the model, often correlated with the error term.
-
How can I identify endogenous variables? Endogenous variables often show a correlation with the error term. Statistical tests, such as the Hausman test, can help identify endogeneity.
-
What are the consequences of ignoring endogeneity? Ignoring endogeneity leads to biased and inconsistent estimates, making your results unreliable and potentially misleading.
-
How can I address endogeneity? Common techniques include instrumental variable regression, two-stage least squares (2SLS), and control function approaches.
-
Are all correlated variables endogenous? No, correlation does not necessarily imply causality or endogeneity. Endogeneity refers specifically to a correlation between a variable and the error term.
Practical Tips: Maximizing the Benefits of Understanding Endogenous Variables
-
Careful Model Specification: Begin by carefully considering all potential variables and their relationships. Include all relevant variables to minimize omitted variable bias.
-
Diagnostic Testing: Utilize statistical tests (e.g., Hausman test) to formally assess for endogeneity.
-
Appropriate Method Selection: Choose the appropriate econometric technique to address endogeneity, such as instrumental variables regression.
-
Robustness Checks: Conduct sensitivity analyses and robustness checks to ensure your results are not overly sensitive to assumptions or model specifications.
-
Transparency and Reporting: Clearly document your methodology, including the tests performed and the methods used to address endogeneity.
Final Conclusion: Wrapping Up with Lasting Insights
Understanding endogenous variables is fundamental to constructing accurate and reliable econometric models. The ability to identify and address endogeneity is a crucial skill for researchers, analysts, and policymakers. By implementing the techniques and strategies discussed in this article, individuals can significantly enhance the quality and validity of their analyses, leading to better decision-making in a variety of fields. The ongoing development of new econometric techniques continues to refine our ability to handle endogeneity, further strengthening the reliability and impact of quantitative research.
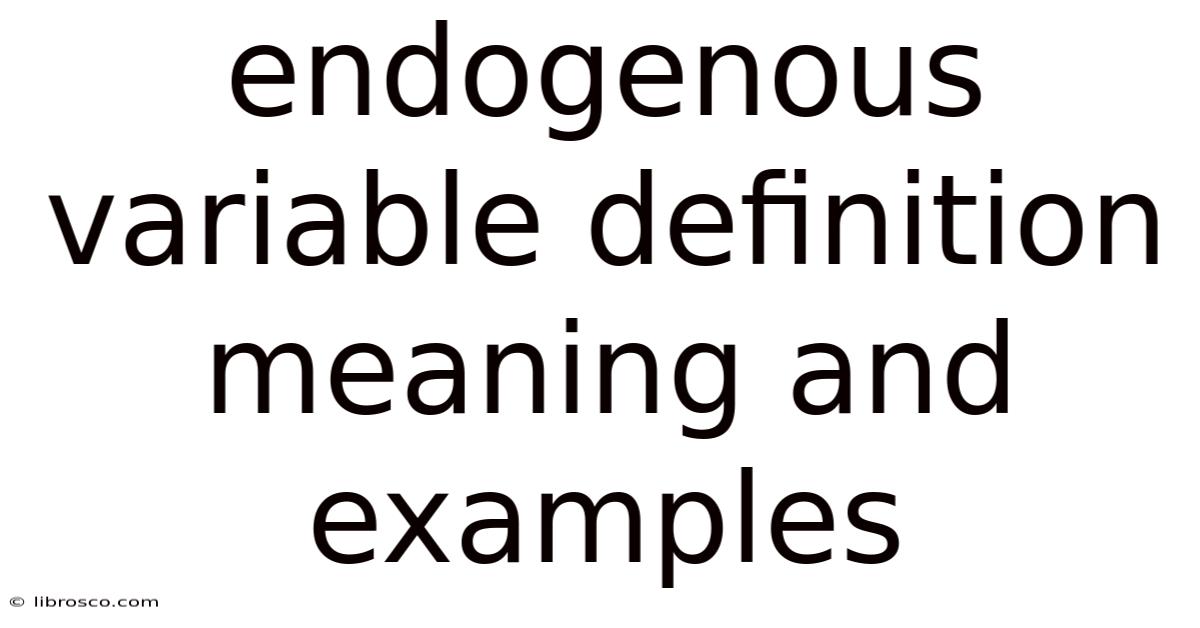
Thank you for visiting our website wich cover about Endogenous Variable Definition Meaning And Examples. We hope the information provided has been useful to you. Feel free to contact us if you have any questions or need further assistance. See you next time and dont miss to bookmark.
Also read the following articles
Article Title | Date |
---|---|
How To Change Billing Cycle Of Idfc Credit Card | Apr 10, 2025 |
When Does Macys Charge Interest | Apr 10, 2025 |
How To Check Cycle Count Iphone | Apr 10, 2025 |
Ending Inventory Definition Calculation And Valuation Methods | Apr 10, 2025 |
What Credit Bureau Does Chase Use In Florida | Apr 10, 2025 |