Empirical Rule Definition Formula Example How Its Used
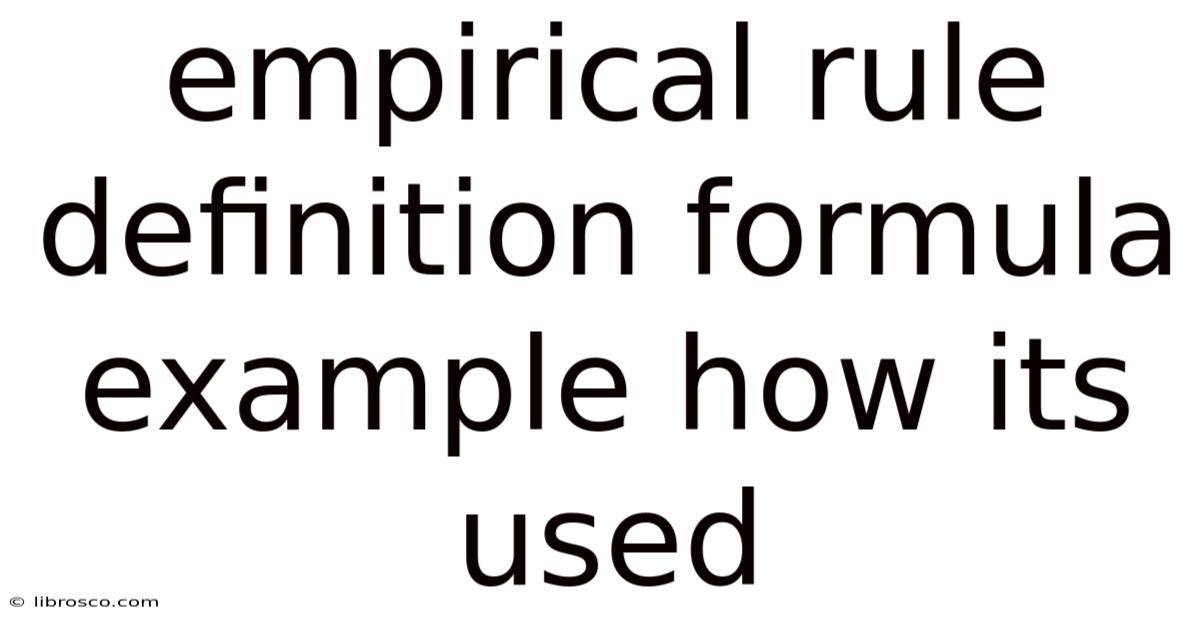
Discover more detailed and exciting information on our website. Click the link below to start your adventure: Visit Best Website meltwatermedia.ca. Don't miss out!
Table of Contents
Decoding the Empirical Rule: Understanding, Applying, and Interpreting the 68-95-99.7 Rule
What if predicting the likelihood of events within a dataset was as simple as applying a single, elegant rule? The Empirical Rule, also known as the 68-95-99.7 rule, offers precisely that – a powerful tool for understanding and interpreting normally distributed data.
Editor’s Note: This article provides a comprehensive overview of the Empirical Rule, its applications, and its limitations. We’ve included numerous examples to illustrate its practical use and help you confidently interpret normally distributed data.
Why the Empirical Rule Matters:
The Empirical Rule is a cornerstone of statistics, providing a straightforward method for estimating probabilities within a normal distribution. Its relevance spans numerous fields, including finance (analyzing stock prices), healthcare (interpreting patient data), manufacturing (controlling product quality), and more. Understanding this rule empowers professionals to make data-driven decisions and extract meaningful insights from their datasets.
Overview: What This Article Covers:
This article will explore the Empirical Rule from its foundational definition to its practical applications. We will cover:
- Definition and Core Concepts: Understanding the underlying principles of the normal distribution and the Empirical Rule itself.
- Formula and Calculation: A step-by-step guide on how to calculate probabilities using the Empirical Rule.
- Applications Across Industries: Examples illustrating how this rule is utilized in various sectors.
- Limitations and Considerations: Recognizing the scenarios where the Empirical Rule may not be applicable.
- The Relationship Between Standard Deviation and the Empirical Rule: Deepening the understanding of the role of standard deviation in probability estimation.
- Real-world examples: Illustrating the application of the rule with detailed scenarios and calculations.
- Frequently Asked Questions: Addressing common queries surrounding the Empirical Rule.
- Practical Tips: Advice on effectively applying the rule in data analysis.
The Research and Effort Behind the Insights:
This article draws upon established statistical principles and widely accepted methodologies. Numerous examples are provided to illustrate the concepts, ensuring clarity and understanding. The information presented is supported by established statistical texts and widely accepted practices within the field.
Key Takeaways:
- The Empirical Rule provides a quick estimate of probabilities for data following a normal distribution.
- It relies on the mean and standard deviation of the dataset.
- The rule is applicable only to data that is approximately normally distributed.
- Understanding its limitations is crucial for accurate interpretation.
Smooth Transition to the Core Discussion:
Now that we've established the importance and scope of the Empirical Rule, let's delve into its core aspects, starting with its definition and foundational principles.
Exploring the Key Aspects of the Empirical Rule:
1. Definition and Core Concepts:
The Empirical Rule is a statistical guideline that states that for a normal distribution:
- Approximately 68% of the data falls within one standard deviation of the mean.
- Approximately 95% of the data falls within two standard deviations of the mean.
- Approximately 99.7% of the data falls within three standard deviations of the mean.
This rule is a consequence of the properties of the normal distribution, a symmetrical bell-shaped curve characterized by its mean (average) and standard deviation (a measure of data spread).
2. Formula and Calculation:
The Empirical Rule doesn't involve a complex formula; instead, it relies on understanding the relationship between the mean (μ), standard deviation (σ), and the percentage of data within certain intervals. To apply the rule, you need to know the mean and standard deviation of your dataset. Once you have these, you can determine the ranges corresponding to the 68%, 95%, and 99.7% intervals:
- 68% interval: (μ - σ, μ + σ)
- 95% interval: (μ - 2σ, μ + 2σ)
- 99.7% interval: (μ - 3σ, μ + 3σ)
3. Applications Across Industries:
The Empirical Rule finds extensive use in various sectors:
-
Quality Control: In manufacturing, it helps determine the acceptable range of variation in product dimensions or performance. If a significant portion of products falls outside the 95% or 99.7% interval, it signals a potential quality issue.
-
Finance: Analyzing stock prices, returns, or risk assessments often involves assuming a normal distribution. The Empirical Rule can help estimate the probability of returns falling within specific ranges.
-
Healthcare: Medical data, such as blood pressure or cholesterol levels, sometimes follows a normal distribution. The Empirical Rule assists in understanding the distribution of these values within a population.
-
Education: Test scores often approximate a normal distribution. The Empirical Rule can be used to interpret the performance of students relative to the average score.
4. Limitations and Considerations:
It's crucial to remember that the Empirical Rule is an approximation and works best for data that closely resembles a perfect normal distribution. Skewed data or data with outliers will significantly affect the accuracy of the rule's estimations. Moreover, the rule only provides estimations; for precise probability calculations, more sophisticated statistical methods are required.
Exploring the Connection Between Standard Deviation and the Empirical Rule:
The standard deviation (σ) is the cornerstone of the Empirical Rule. It measures the spread or dispersion of data around the mean. A larger standard deviation indicates a wider spread, meaning the data is more dispersed, while a smaller standard deviation indicates a tighter distribution around the mean. The intervals defined by the Empirical Rule directly utilize multiples of the standard deviation to delineate the probability regions. This emphasizes that the standard deviation is not just a descriptive statistic, but a critical component in probabilistic estimations for normally distributed data.
Key Factors to Consider:
-
Data normality: Verify that your data is approximately normally distributed before applying the Empirical Rule. Techniques like histograms, Q-Q plots, and normality tests can help assess this.
-
Sample size: The accuracy of the Empirical Rule improves with larger sample sizes.
-
Outliers: Outliers can significantly distort the results, making the Empirical Rule less reliable.
Real-World Examples:
Example 1: Student Test Scores:
Suppose the average score on a standardized test is 75, with a standard deviation of 10. Using the Empirical Rule:
- Approximately 68% of students scored between 65 (75-10) and 85 (75+10).
- Approximately 95% of students scored between 55 (75-20) and 95 (75+20).
- Approximately 99.7% of students scored between 45 (75-30) and 105 (75+30).
Example 2: Manufacturing Process:
A factory produces bolts with a mean diameter of 10mm and a standard deviation of 0.1mm. Using the Empirical Rule:
- Approximately 68% of the bolts have diameters between 9.9mm and 10.1mm.
- Approximately 95% of the bolts have diameters between 9.8mm and 10.2mm.
- Approximately 99.7% of the bolts have diameters between 9.7mm and 10.3mm.
Conclusion: Reinforcing the Connection:
The relationship between standard deviation and the Empirical Rule is fundamental. The standard deviation acts as the scaling factor determining the spread of the data within the probability intervals dictated by the rule. Understanding this connection is crucial for accurate interpretation and application.
Further Analysis: Examining Standard Deviation in Greater Detail:
The standard deviation's role extends beyond the Empirical Rule. It's a critical measure in various statistical analyses, informing decisions on data variability, risk assessment, and the reliability of statistical inferences. Its significance underlines the importance of understanding its calculation and interpretation within the context of different statistical procedures.
FAQ Section:
Q: What happens if my data is not normally distributed?
A: The Empirical Rule is only accurate for approximately normally distributed data. For non-normal data, other methods like Chebyshev's inequality might be more appropriate, although they provide less precise estimations.
Q: Can I use the Empirical Rule for small sample sizes?
A: While you can technically apply the rule, its accuracy diminishes with smaller sample sizes. The larger the sample, the more likely the data will approximate a normal distribution, making the Empirical Rule more reliable.
Q: How can I determine if my data is normally distributed?
A: Several methods exist, including visual inspection using histograms and Q-Q plots, and formal statistical tests like the Shapiro-Wilk test or Kolmogorov-Smirnov test.
Practical Tips:
-
Visualize your data: Create histograms or box plots to get a visual sense of the data's distribution before applying the Empirical Rule.
-
Check for outliers: Identify and handle outliers appropriately, as they can significantly skew results.
-
Use appropriate statistical software: Software packages like R or Python can help with normality tests and calculations.
Final Conclusion:
The Empirical Rule, while simple, is a powerful tool for understanding and interpreting normally distributed data. Its ease of application and wide-ranging applicability across diverse fields make it a valuable asset for data analysts, researchers, and decision-makers. However, always remember to assess the normality of your data and be aware of the rule's limitations for accurate and reliable interpretations. By understanding its strengths and weaknesses, you can harness its power to extract meaningful insights from your data.
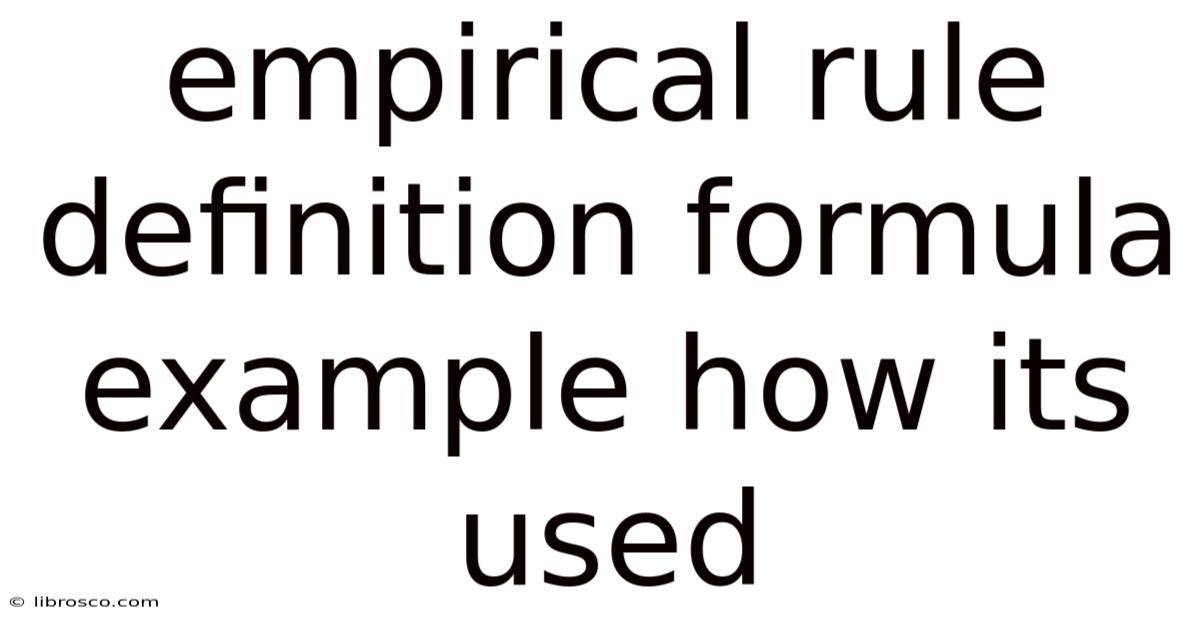
Thank you for visiting our website wich cover about Empirical Rule Definition Formula Example How Its Used. We hope the information provided has been useful to you. Feel free to contact us if you have any questions or need further assistance. See you next time and dont miss to bookmark.
Also read the following articles
Article Title | Date |
---|---|
How To See Credit Score On Citibank App | Apr 10, 2025 |
Cara Mengubah Cicilan Kartu Kredit Citibank | Apr 10, 2025 |
How To Change Billing Cycle Of Credit Card Sbi | Apr 10, 2025 |
How Can I Change My Amazon Prime Payment Date | Apr 10, 2025 |
What Credit Score Does Navy Federal Use For Auto Loans Reddit | Apr 10, 2025 |