Definition Z Score
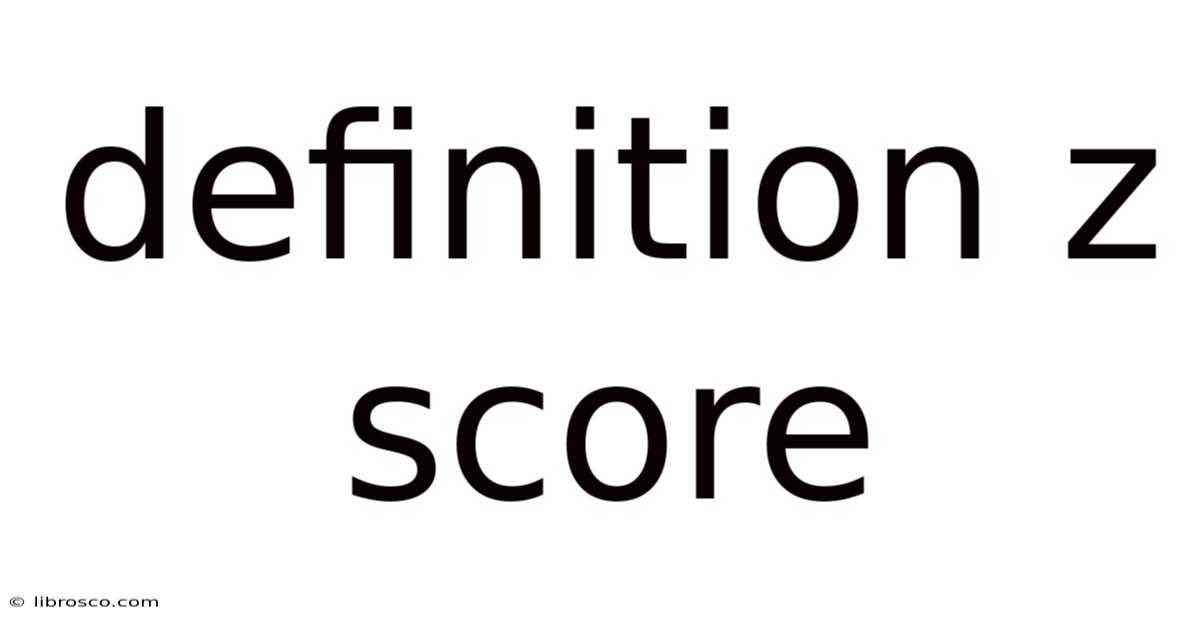
Discover more detailed and exciting information on our website. Click the link below to start your adventure: Visit Best Website meltwatermedia.ca. Don't miss out!
Table of Contents
Understanding the Z-Score: A Comprehensive Guide
What if unlocking the secrets of data analysis hinged on mastering the z-score? This powerful statistical tool provides invaluable insights into data distribution and is essential for a wide range of applications.
Editor’s Note: This article on the z-score provides a comprehensive overview, exploring its definition, calculations, applications, and interpretations. It aims to equip readers with a solid understanding of this crucial statistical concept.
Why Z-Scores Matter: Relevance, Practical Applications, and Industry Significance
The z-score, also known as the standard score, is a fundamental concept in statistics with widespread applications across diverse fields. It allows for the standardization of data, enabling comparisons across different datasets with varying means and standard deviations. This standardized measure is crucial for hypothesis testing, identifying outliers, and understanding the relative position of a data point within a distribution. Its importance spans various industries, including finance (risk assessment), healthcare (clinical trials), education (standardized testing), and engineering (quality control).
Overview: What This Article Covers
This article provides a detailed exploration of the z-score, covering its definition, calculation methods, interpretations, practical applications in various contexts, and common misconceptions. Readers will gain a thorough understanding of how to calculate and interpret z-scores, along with practical examples to illustrate their real-world usage.
The Research and Effort Behind the Insights
This article is the result of extensive research, drawing upon established statistical textbooks, peer-reviewed journal articles, and reputable online resources. The information presented is based on well-established statistical principles and aims to provide a clear, accurate, and accessible explanation of the z-score concept.
Key Takeaways:
- Definition and Core Concepts: A precise definition of the z-score and its underlying principles.
- Calculation Methods: Step-by-step instructions for calculating z-scores for individual data points and for entire datasets.
- Interpreting Z-Scores: Understanding the meaning and implications of positive, negative, and extreme z-scores.
- Applications Across Industries: Exploring the diverse applications of z-scores in various fields.
- Limitations and Considerations: Acknowledging the limitations and potential pitfalls of using z-scores.
Smooth Transition to the Core Discussion:
With a foundational understanding of the z-score's significance, let's delve into its core aspects, starting with a precise definition and then progressing to its calculation, interpretation, and practical applications.
Exploring the Key Aspects of the Z-Score
1. Definition and Core Concepts:
The z-score represents the number of standard deviations a data point lies away from the mean of its distribution. A positive z-score indicates the data point is above the mean, while a negative z-score signifies it's below the mean. A z-score of 0 indicates the data point is exactly at the mean. The z-score effectively transforms a raw data point into a standardized value, allowing for comparisons across datasets with different scales and units.
2. Calculation Methods:
The formula for calculating a z-score is:
z = (x - μ) / σ
Where:
z
is the z-scorex
is the individual data pointμ
is the population meanσ
is the population standard deviation
If the population parameters (μ and σ) are unknown, sample statistics (x̄ and s) can be used as estimates:
z = (x - x̄) / s
Where:
x̄
is the sample means
is the sample standard deviation
Calculating z-scores for an entire dataset involves applying this formula to each data point individually. Statistical software packages like R, Python (with libraries like SciPy), and Excel can automate this process for large datasets.
3. Interpreting Z-Scores:
The interpretation of a z-score depends on its magnitude and sign. A z-score of +1 indicates the data point is one standard deviation above the mean, while a z-score of -1 indicates it's one standard deviation below the mean. Z-scores between -1 and +1 are considered relatively close to the mean. Z-scores greater than +2 or less than -2 are often considered outliers, representing data points that are significantly far from the mean. The exact threshold for identifying outliers can depend on the context and the desired level of stringency.
4. Applications Across Industries:
The versatility of z-scores makes them applicable across a wide range of disciplines:
- Finance: Assessing investment risk, identifying unusual market fluctuations, and detecting fraudulent transactions.
- Healthcare: Analyzing clinical trial data, evaluating patient outcomes, and identifying patients with extreme values on physiological measurements.
- Education: Standardizing test scores, comparing student performance across different schools or years, and identifying students who require additional support.
- Manufacturing: Implementing quality control measures, identifying defective products, and optimizing manufacturing processes.
- Sports Analytics: Evaluating athlete performance, identifying trends, and predicting future outcomes.
Closing Insights: Summarizing the Core Discussion
The z-score serves as a powerful tool for data analysis, enabling the standardization of data and facilitating meaningful comparisons across various datasets. Understanding its calculation, interpretation, and limitations is crucial for making informed decisions based on data-driven insights. Its wide-ranging applications highlight its significance across various industries.
Exploring the Connection Between Probability Distributions and Z-Scores
The z-score's power is intrinsically linked to probability distributions, particularly the standard normal distribution. The standard normal distribution has a mean of 0 and a standard deviation of 1. By transforming raw data into z-scores, we can determine the probability of observing a data point at or below a certain value. This probability is often found using z-tables or statistical software.
Key Factors to Consider:
- Roles and Real-World Examples: The ability to convert a raw score into a probability through z-scores is vital in fields like quality control, where one might want to know the probability of producing a defective item.
- Risks and Mitigations: Misinterpreting z-scores as having direct causal implications or applying them to non-normally distributed data can lead to inaccurate conclusions. Transforming the data to approximate normality or employing non-parametric methods can mitigate this risk.
- Impact and Implications: Understanding the probability associated with a z-score allows for informed decision-making in various applications, from setting acceptance criteria in quality control to determining the statistical significance of a hypothesis test.
Conclusion: Reinforcing the Connection
The connection between z-scores and probability distributions, particularly the standard normal distribution, underscores the importance of the z-score in statistical inference and decision-making. By properly understanding and applying this connection, researchers and analysts can leverage z-scores to gain deep insights from their data.
Further Analysis: Examining Probability Tables and Software in Greater Detail
Z-tables, also known as standard normal tables, provide cumulative probabilities for different z-scores. These tables list the probability that a randomly selected value from a standard normal distribution will be less than or equal to a specific z-score. Statistical software packages offer more sophisticated methods for calculating these probabilities and can handle more complex probability calculations involving z-scores.
FAQ Section: Answering Common Questions About Z-Scores
-
What is a z-score? A z-score is a standardized value that indicates how many standard deviations a data point is from the mean of its distribution.
-
How is a z-score calculated? The formula is
z = (x - μ) / σ
(or using sample statistics if population parameters are unknown). -
What does a positive z-score mean? A positive z-score means the data point is above the mean.
-
What does a negative z-score mean? A negative z-score means the data point is below the mean.
-
What is the significance of a z-score of 0? A z-score of 0 means the data point is equal to the mean.
-
How are z-scores used in hypothesis testing? Z-scores are used to calculate p-values, which determine the statistical significance of a test result.
-
Can z-scores be used for non-normal distributions? While z-scores are most accurately interpreted with normal distributions, transformations may allow for approximate usage in some cases. However, non-parametric methods are often more appropriate for non-normal data.
Practical Tips: Maximizing the Benefits of Z-Scores
-
Understand the Basics: Ensure a firm grasp of the z-score formula, its interpretation, and its relationship with the standard normal distribution.
-
Identify Data Distribution: Before calculating z-scores, assess whether the data is approximately normally distributed. If not, consider data transformations or alternative methods.
-
Choose Appropriate Software: Utilize statistical software for efficient calculation and interpretation of z-scores, especially for large datasets.
-
Contextualize Results: Interpret z-scores within the context of the problem and the specific data being analyzed.
-
Consider Limitations: Be mindful of the assumptions and limitations of using z-scores.
Final Conclusion: Wrapping Up with Lasting Insights
The z-score is a fundamental concept in statistics with far-reaching applications. Its ability to standardize data, enabling comparisons and probability calculations, makes it an indispensable tool across numerous disciplines. By mastering the calculation, interpretation, and limitations of z-scores, individuals can unlock a deeper understanding of their data and make more informed decisions. The z-score is not just a statistical measure; it's a key to unlocking data-driven insights.
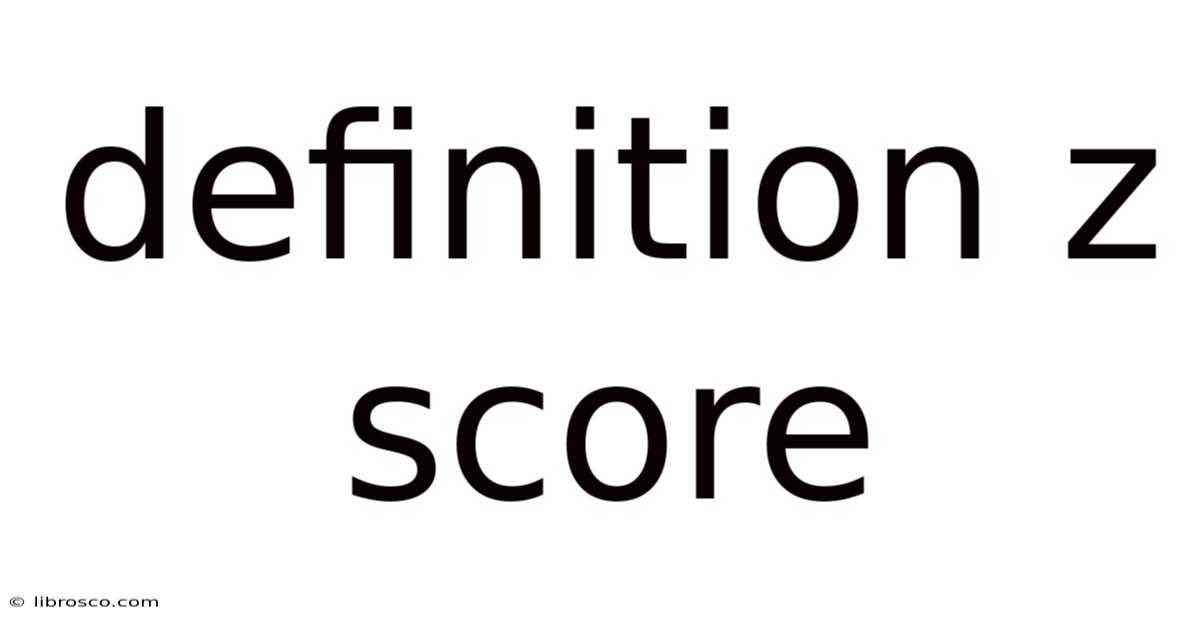
Thank you for visiting our website wich cover about Definition Z Score. We hope the information provided has been useful to you. Feel free to contact us if you have any questions or need further assistance. See you next time and dont miss to bookmark.
Also read the following articles
Article Title | Date |
---|---|
Cfa West | Apr 02, 2025 |
What Is The Credit Limit For Discover It | Apr 02, 2025 |
Difference Between Initial Yield And Equivalent Yield | Apr 02, 2025 |
Z Effective Definition | Apr 02, 2025 |
Grace Period Meaning In Loan | Apr 02, 2025 |