Commodity Selection Index Csi Definition
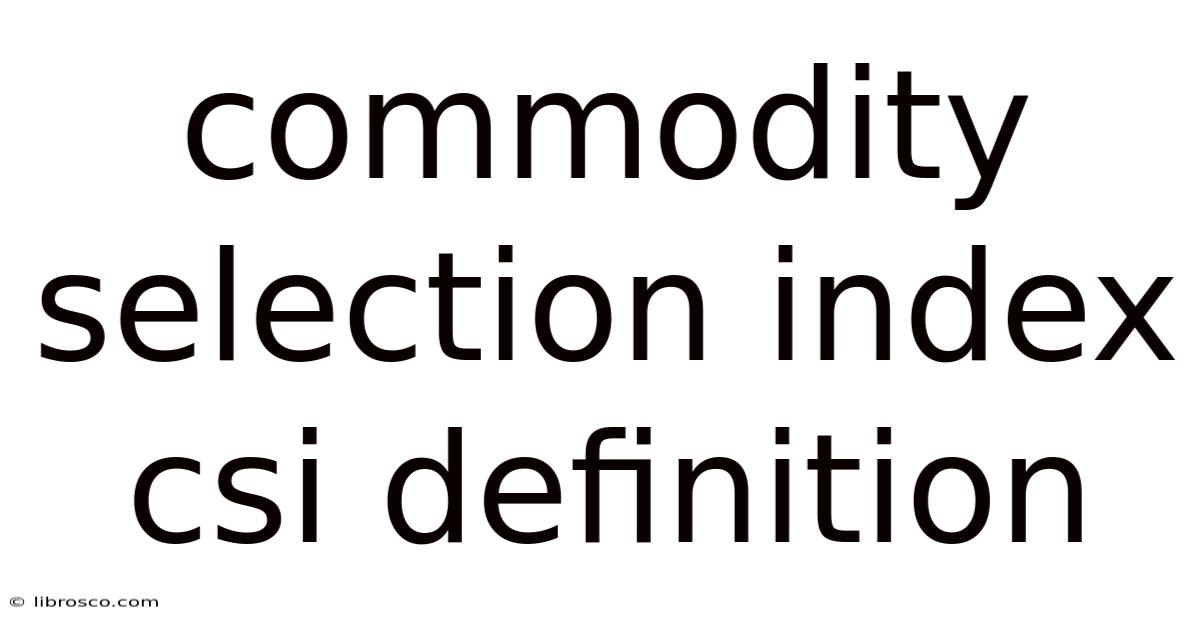
Discover more detailed and exciting information on our website. Click the link below to start your adventure: Visit Best Website meltwatermedia.ca. Don't miss out!
Table of Contents
Decoding the Commodity Selection Index (CSI): A Deep Dive into Definition, Applications, and Implications
What if a single index could significantly improve investment decisions in the volatile world of commodities? The Commodity Selection Index (CSI), a powerful analytical tool, offers just that, providing crucial insights for navigating the complexities of commodity markets.
Editor’s Note: This article provides a comprehensive overview of the Commodity Selection Index (CSI), its definition, methodology, applications, and implications for investors and businesses alike. Updated with the latest information, this resource aims to empower readers with a deeper understanding of this vital analytical tool.
Why the Commodity Selection Index (CSI) Matters:
The commodity market is notoriously volatile, influenced by a multitude of factors including geopolitical events, weather patterns, technological advancements, and global economic trends. Effective investment and risk management in this arena demand sophisticated analytical tools. The CSI serves precisely this purpose, providing a structured framework for identifying promising commodities and mitigating potential risks. Its relevance extends beyond individual investors, impacting businesses involved in commodity sourcing, production, and trading. Understanding the CSI is crucial for making informed decisions, optimizing resource allocation, and navigating the challenges of this dynamic market.
Overview: What This Article Covers:
This article will delve into the intricacies of the Commodity Selection Index (CSI), starting with its precise definition and exploring its various methodologies. We'll analyze its practical applications across diverse sectors, examining its strengths and limitations. Furthermore, we will explore the key factors that influence CSI calculations, highlighting the significance of data accuracy and model selection. Finally, we will discuss the broader implications of the CSI for investment strategies, risk management, and future market trends.
The Research and Effort Behind the Insights:
This in-depth analysis draws upon extensive research, incorporating insights from academic literature, industry reports, and practical applications of CSI methodologies. Data from reputable sources, including financial news outlets, commodity market data providers, and government publications, have been meticulously reviewed to ensure the accuracy and reliability of the information presented. The structured approach adopted ensures a clear, coherent, and evidence-based understanding of the CSI and its implications.
Key Takeaways:
- Definition and Core Concepts: A precise definition of the CSI and its underlying principles.
- Methodologies and Calculations: Different approaches to constructing a CSI and the key variables involved.
- Applications Across Industries: How the CSI is utilized in various sectors, including finance, agriculture, and energy.
- Strengths and Limitations: An objective assessment of the CSI's advantages and potential drawbacks.
- Future Implications: The potential impact of the CSI on commodity market analysis and investment strategies.
Smooth Transition to the Core Discussion:
Now that the importance and scope of this analysis have been established, let's embark on a detailed exploration of the Commodity Selection Index (CSI), starting with its core definition and the various approaches to its construction.
Exploring the Key Aspects of the Commodity Selection Index (CSI):
1. Definition and Core Concepts:
The Commodity Selection Index (CSI) is a quantitative tool used to rank and select commodities based on a predefined set of criteria. It doesn't represent the price of a commodity basket like a commodity price index, but rather serves as a relative ranking system. The specific criteria used to construct a CSI can vary widely depending on the investor's objectives, risk tolerance, and market outlook. However, common factors incorporated include:
- Price Momentum: The recent price performance of the commodity. A rising price might indicate strong demand or positive market sentiment.
- Supply and Demand Dynamics: Analysis of production levels, consumption patterns, and inventory levels to predict future price movements.
- Geopolitical Factors: The impact of political instability, trade wars, or sanctions on commodity prices.
- Economic Indicators: Correlation between commodity prices and macroeconomic indicators like inflation, interest rates, and GDP growth.
- Technical Indicators: Use of technical analysis tools like moving averages, relative strength index (RSI), and MACD to identify potential price trends.
2. Methodologies and Calculations:
Several methodologies exist for constructing a CSI. Some common approaches include:
- Factor-Based Models: These models assign weights to different factors based on their perceived importance and correlation with future price movements. For example, a model might give a higher weight to supply and demand factors than to geopolitical events.
- Rank-Based Models: These models rank commodities based on each factor individually and then aggregate the ranks to create an overall score.
- Machine Learning Models: Advanced models employing machine learning algorithms can analyze vast datasets and identify complex relationships between variables to predict future commodity performance. These models can adapt and learn over time, improving accuracy.
3. Applications Across Industries:
The applications of the CSI extend far beyond the realm of individual investors:
- Portfolio Management: Investors use CSIs to construct diversified commodity portfolios, selecting commodities with high potential returns and low correlations to reduce overall portfolio risk.
- Commodity Trading: Traders utilize CSIs to identify potential entry and exit points for specific commodities, capitalizing on short-term price fluctuations.
- Risk Management: Companies involved in commodity sourcing or production employ CSIs to identify potential supply chain disruptions or price volatility risks and develop appropriate hedging strategies.
- Investment Strategy Development: Financial analysts use CSIs to create long-term investment strategies, aligning their portfolio with projected market trends.
4. Strengths and Limitations:
The CSI offers several advantages:
- Structured Approach: Provides a systematic and objective way to evaluate and select commodities.
- Diversification Potential: Enables the construction of diversified portfolios to mitigate risk.
- Improved Decision-Making: Offers data-driven insights to support informed investment decisions.
However, limitations exist:
- Data Dependency: The accuracy of the CSI heavily relies on the quality and reliability of the underlying data. Inaccurate or incomplete data can lead to flawed conclusions.
- Model Risk: The chosen methodology can significantly influence the results. A model that performs well in one market environment might fail in another.
- Future Uncertainty: Predicting future commodity prices remains inherently challenging, and no model can guarantee perfect accuracy.
5. Impact on Innovation:
The development and refinement of CSI methodologies continue to drive innovation in commodity market analysis. The increasing use of machine learning and artificial intelligence is leading to more sophisticated models capable of processing vast datasets and identifying intricate patterns. This innovation empowers investors and businesses with more precise forecasts and improved risk management capabilities.
Exploring the Connection Between Data Quality and the Commodity Selection Index (CSI):
The accuracy and reliability of the CSI are inextricably linked to the quality of the input data. Inaccurate or incomplete data can lead to misleading results, undermining the utility of the index.
Key Factors to Consider:
- Data Sources: The credibility and reputation of the data providers are crucial. Using data from multiple, reputable sources can help mitigate errors and biases.
- Data Frequency: The frequency of data updates influences the responsiveness of the CSI to market changes. More frequent updates provide a more dynamic and up-to-date picture.
- Data Consistency: Inconsistent data formats or measurement units can create errors in the calculations. Standardization of data is essential for reliable results.
Roles and Real-World Examples:
Consider a CSI that relies on government-reported agricultural production figures. If these figures are consistently underreported due to bureaucratic inefficiencies, the CSI will likely undervalue the relevant agricultural commodities, leading to inaccurate investment decisions.
Risks and Mitigations:
The risk of inaccurate data can be mitigated through:
- Data Validation: Thorough verification of data sources and cross-checking with multiple sources.
- Data Cleaning: Identifying and correcting inconsistencies or errors in the data.
- Sensitivity Analysis: Testing the CSI's sensitivity to changes in input data to identify potential vulnerabilities.
Impact and Implications:
The impact of data quality on the CSI is profound. Inaccurate data can lead to poor investment decisions, resulting in financial losses for investors and businesses. Conversely, high-quality data enhances the accuracy and reliability of the CSI, fostering better decision-making and optimized resource allocation.
Conclusion: Reinforcing the Connection:
The relationship between data quality and the CSI is paramount. Without accurate and reliable data, the CSI's value diminishes significantly. By implementing rigorous data validation and cleaning procedures, investors and businesses can ensure the integrity of the CSI and maximize its potential for improving investment outcomes and risk management.
Further Analysis: Examining Data Sources in Greater Detail:
Investigating the diverse data sources utilized in CSI construction is crucial. These sources can include:
- Government Agencies: Official statistics on production, consumption, and trade.
- Industry Associations: Reports and forecasts from industry-specific organizations.
- Financial Data Providers: Real-time market data and historical price information.
- Satellite Imagery: Data from satellites can provide insights into agricultural yields and other relevant factors.
Each source presents unique strengths and weaknesses. For example, government data might lag behind real-time market developments, while industry association reports could reflect biases or vested interests. A comprehensive CSI methodology would ideally integrate data from diverse sources, carefully weighing their strengths and limitations.
FAQ Section: Answering Common Questions About the Commodity Selection Index (CSI):
Q: What is the CSI's primary purpose?
A: The CSI's primary purpose is to provide a structured and quantitative framework for ranking and selecting commodities based on a predefined set of criteria. It aims to aid in decision-making related to investment, trading, and risk management in commodity markets.
Q: How does the CSI differ from a commodity price index?
A: Unlike a commodity price index, which tracks the aggregate price of a basket of commodities, the CSI is a relative ranking system. It doesn't reflect the absolute price level but rather the relative attractiveness of different commodities based on a range of factors.
Q: Can the CSI predict future commodity prices with certainty?
A: No, the CSI cannot predict future prices with certainty. It provides a probabilistic assessment based on historical data and projected trends, but market dynamics are inherently unpredictable.
Q: What are the key limitations of using a CSI?
A: The accuracy of a CSI is dependent on data quality, the suitability of the chosen model, and the unpredictable nature of commodity markets. Overreliance on a CSI without considering other factors can lead to flawed decisions.
Practical Tips: Maximizing the Benefits of the CSI:
-
Understand your objectives: Clearly define your investment goals and risk tolerance before selecting a CSI methodology.
-
Choose reliable data sources: Use data from multiple, reputable sources to ensure accuracy and mitigate biases.
-
Test and validate your model: Backtest the CSI using historical data to evaluate its performance and refine the methodology.
-
Combine the CSI with other analytical tools: Don't rely solely on the CSI; incorporate fundamental and technical analysis to gain a comprehensive understanding of the market.
-
Regularly review and update your model: Market conditions change, so the CSI methodology needs to be regularly reviewed and updated to maintain accuracy.
Final Conclusion: Wrapping Up with Lasting Insights:
The Commodity Selection Index (CSI) represents a powerful tool for navigating the complexities of commodity markets. By systematically evaluating a range of factors and employing a structured approach, the CSI enhances investment decision-making, optimizes resource allocation, and mitigates risks. However, it is crucial to acknowledge its limitations and employ it in conjunction with other analytical methods to develop comprehensive investment strategies. Continuous advancements in data analytics and machine learning will further refine the CSI, expanding its applications and enhancing its ability to provide valuable insights into the ever-evolving world of commodities.
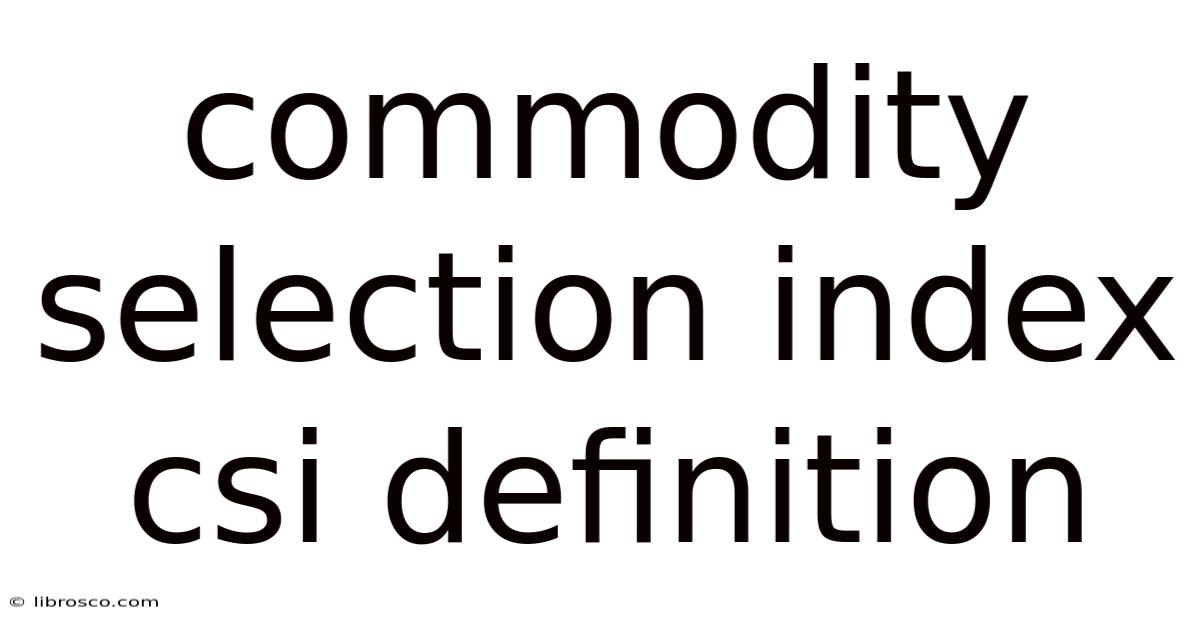
Thank you for visiting our website wich cover about Commodity Selection Index Csi Definition. We hope the information provided has been useful to you. Feel free to contact us if you have any questions or need further assistance. See you next time and dont miss to bookmark.
Also read the following articles
Article Title | Date |
---|---|
How Much Is Starbucks Health Insurance | Mar 13, 2025 |
Commodity Market Definition Types Example And How It Works | Mar 13, 2025 |
Commissioner Of Banking Definition | Mar 13, 2025 |
What Is A Beneficiary For Health Insurance | Mar 13, 2025 |
Common Size Balance Sheet Definition Formula Example | Mar 13, 2025 |