Casualty Adalah
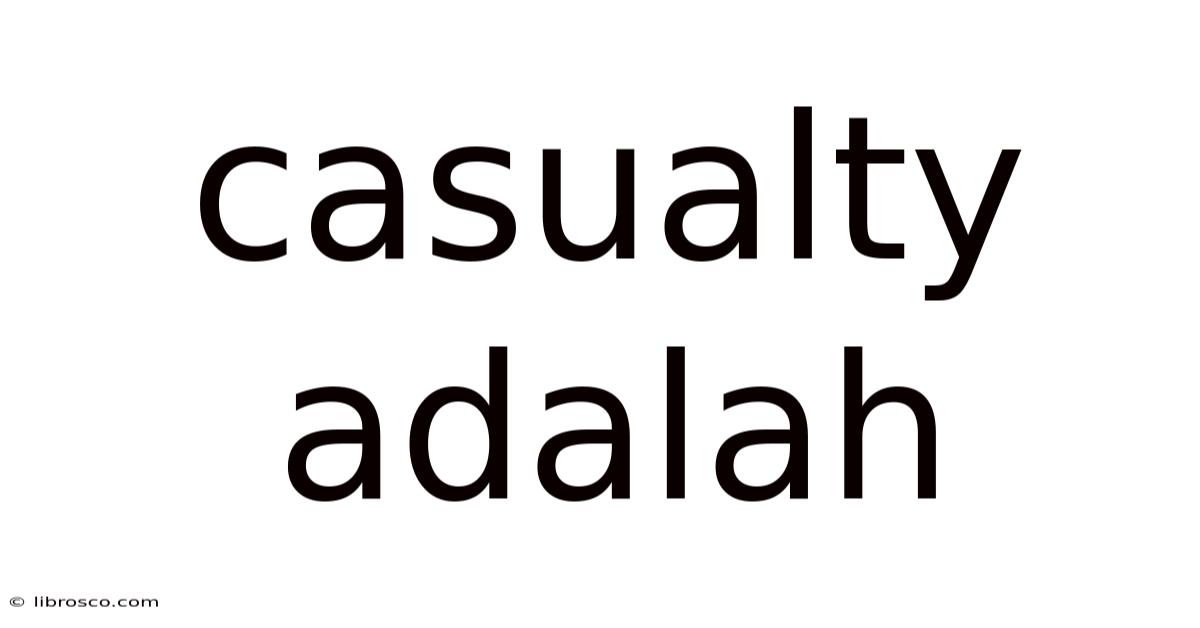
Discover more detailed and exciting information on our website. Click the link below to start your adventure: Visit Best Website meltwatermedia.ca. Don't miss out!
Table of Contents
Unveiling the Multifaceted Nature of Casualty: A Comprehensive Exploration
What if our understanding of casualty profoundly impacts our ability to predict, mitigate, and even prevent catastrophic events? This intricate concept, far from being a simple cause-and-effect relationship, holds the key to understanding complex systems and shaping a more resilient future.
Editor’s Note: This article on "casualty" provides a comprehensive overview of the term's various interpretations across different fields. We explore its nuances, practical applications, and challenges in understanding causality in complex systems. This in-depth analysis aims to equip readers with a thorough understanding of this crucial concept.
Why "Casualty" Matters: Beyond Simple Cause and Effect
The term "casualty" often evokes images of victims in accidents or warfare. While this is a significant aspect, the concept of casualty extends far beyond this immediate understanding. In its broadest sense, casualty refers to an event or occurrence that is the consequence of another event or action. This encompasses a wide spectrum of scenarios, from the relatively simple (a dropped glass shattering due to gravity) to incredibly complex systems (the interplay of factors leading to a global pandemic). Understanding casualty is crucial for various disciplines, including:
- Healthcare: Analyzing casualty rates in hospitals to improve patient safety and treatment protocols.
- Engineering: Designing safer infrastructure and products by understanding the causal factors behind accidents.
- Insurance: Assessing risk and determining premiums based on the probability of certain events.
- Social Sciences: Identifying the root causes of social issues and developing effective intervention strategies.
- Climate Science: Modeling and predicting the impact of climate change by understanding causal links between greenhouse gas emissions and environmental consequences.
Overview: What This Article Covers
This article delves into the multifaceted nature of casualty, exploring its definitions, applications, and challenges. We will examine various perspectives on causality, including philosophical viewpoints and practical methodologies employed in different fields. Furthermore, we will investigate the crucial role of data analysis, statistical modeling, and counterfactual reasoning in uncovering causal relationships. Finally, we will address the complexities involved in understanding causality in intricate systems and offer practical insights for navigating these challenges.
The Research and Effort Behind the Insights
This article is the result of extensive research, drawing upon a range of sources, including academic literature in philosophy, statistics, epidemiology, and engineering. We have reviewed numerous case studies, empirical research, and expert opinions to ensure a comprehensive and accurate portrayal of the concept of casualty. The structured approach used ensures that the information presented is clear, concise, and readily accessible to a broad audience.
Key Takeaways:
- Definition and Core Concepts: A detailed explanation of causality, its philosophical underpinnings, and its practical applications.
- Types of Causal Relationships: Exploration of different types of causal links, including direct, indirect, and mediating relationships.
- Methods for Establishing Causality: Examination of various research methods used to determine causal relationships, such as randomized controlled trials, observational studies, and causal inference techniques.
- Challenges in Causal Inference: Discussion of biases and limitations in determining causality, including confounding variables and selection bias.
- Applications in Various Fields: Examples of how understanding causality is utilized in healthcare, engineering, social sciences, and climate science.
Smooth Transition to the Core Discussion:
Having established the significance and scope of casualty, we will now embark on a detailed exploration of its key facets. We will begin by examining the philosophical foundations of causality before moving on to practical methodologies for identifying and interpreting causal relationships.
Exploring the Key Aspects of Casualty:
1. Definition and Core Concepts:
The concept of causality is central to our understanding of the world. It refers to the relationship between cause and effect, where one event (the cause) leads to another event (the effect). However, defining causality rigorously has proven challenging for philosophers throughout history. Different perspectives exist, including:
- Deterministic Causality: This view suggests that every event has a specific cause and that, given the same initial conditions, the same effect will always occur.
- Probabilistic Causality: This perspective acknowledges the role of chance and probability in causal relationships. It suggests that a cause increases the probability of an effect occurring, but it does not guarantee it.
- Counterfactual Causality: This approach defines causality in terms of what would have happened if the cause had not occurred. If the effect would not have occurred without the cause, then a causal relationship is established.
2. Types of Causal Relationships:
Causal relationships can be complex and multifaceted. They can be:
- Direct: A straightforward relationship where one event directly leads to another. Example: Turning on a light switch (cause) causes the light to turn on (effect).
- Indirect: A more intricate relationship where a chain of events connects the cause and effect. Example: Exposure to asbestos (cause) leads to lung cancer (effect) through a series of intermediate biological processes.
- Mediating: A relationship where a third variable (the mediator) explains the link between the cause and effect. Example: Stress (cause) leads to unhealthy eating habits (mediator), which in turn leads to weight gain (effect).
- Moderating: A relationship where a third variable (the moderator) influences the strength or direction of the causal link. Example: The effect of exercise (cause) on weight loss (effect) might be moderated by diet (moderator).
3. Methods for Establishing Causality:
Establishing causal relationships requires rigorous methodologies. Key approaches include:
- Randomized Controlled Trials (RCTs): These are considered the gold standard in establishing causality. They involve randomly assigning participants to different groups (treatment and control) to minimize bias.
- Observational Studies: These studies observe existing relationships without manipulating variables. While they cannot definitively prove causality, they can provide strong evidence suggestive of causal links.
- Causal Inference Techniques: These statistical methods use sophisticated algorithms to analyze data and infer causal relationships, even in the absence of randomized experiments. This includes techniques like propensity score matching and instrumental variables.
4. Challenges in Causal Inference:
Determining causality is often challenging due to several factors:
- Confounding Variables: These are variables that affect both the cause and effect, potentially masking or distorting the true causal relationship.
- Selection Bias: This occurs when the selection of participants for a study is not representative of the population of interest.
- Reverse Causality: This occurs when the direction of causality is misinterpreted; the effect is mistakenly identified as the cause.
5. Applications in Various Fields:
Understanding causality is crucial across numerous fields:
- Healthcare: Identifying risk factors for diseases, evaluating the effectiveness of treatments, and improving patient safety.
- Engineering: Understanding the causes of accidents and failures to design safer products and infrastructure.
- Social Sciences: Identifying the root causes of social problems, such as poverty and crime, to develop effective interventions.
- Climate Science: Attributing changes in climate patterns to specific factors, such as greenhouse gas emissions, to inform policy decisions.
Closing Insights: Summarizing the Core Discussion
Causality is not a simple concept; it is a complex and multifaceted area of study with profound implications across a wide spectrum of disciplines. While establishing definitive causal relationships can be challenging, rigorous methodologies and careful consideration of potential biases are crucial for gaining a deeper understanding of how events unfold and influence one another.
Exploring the Connection Between Data Analysis and Casualty
Data analysis plays a pivotal role in uncovering and understanding causal relationships. The sheer volume and complexity of data generated in today's world necessitate sophisticated analytical techniques to unravel the intricate web of cause and effect. The connection is profound because:
-
Data Provides Evidence: Data serves as the foundation for identifying potential causal links. By analyzing patterns and correlations within datasets, researchers can generate hypotheses about causal relationships.
-
Statistical Modeling Aids Inference: Statistical models allow researchers to quantify the strength and direction of causal relationships, considering confounding variables and other sources of bias. Techniques such as regression analysis and structural equation modeling are commonly employed.
-
Causal Inference Methods Rely on Data: Advanced causal inference techniques, such as propensity score matching and instrumental variables, rely heavily on data to estimate causal effects accurately. These methods help to disentangle the effects of multiple variables and to isolate the impact of specific causes.
Key Factors to Consider:
- Data Quality: The accuracy and reliability of causal inferences depend heavily on the quality of the data. Inaccurate or incomplete data can lead to misleading conclusions.
- Data Bias: Data bias can significantly distort causal inferences. It's essential to identify and address potential biases in data collection and analysis.
- Model Selection: Choosing the appropriate statistical model is crucial for accurately representing causal relationships. An inappropriate model can lead to incorrect conclusions.
Risks and Mitigations:
- Oversimplification: Attempting to simplify complex causal relationships can lead to an incomplete or inaccurate understanding.
- Correlation vs. Causation: Correlation does not imply causation. Observing a correlation between two variables does not necessarily indicate a causal relationship.
- Bias in Interpretation: Researchers' biases can influence the interpretation of data and causal inferences. Transparency and rigorous methodology are crucial to mitigate this risk.
Impact and Implications:
The accurate identification and understanding of causal relationships through data analysis have profound implications for various fields. It allows for more effective:
- Policy Making: Informed policy decisions based on a strong understanding of causal relationships.
- Intervention Strategies: Development of targeted interventions to address the root causes of problems.
- Prediction and Forecasting: More accurate predictions of future events based on a clear understanding of causal mechanisms.
Conclusion: Reinforcing the Connection
The connection between data analysis and casualty is undeniable. Data provides the essential evidence, statistical modeling offers the tools for analysis, and causal inference methods enable the identification of causal relationships, even in complex systems. However, careful attention must be paid to data quality, bias, and model selection to ensure the accuracy and reliability of causal inferences.
Further Analysis: Examining Counterfactual Reasoning in Greater Detail
Counterfactual reasoning is a crucial aspect of causal inference. It involves considering what would have happened if a particular event had not occurred. This approach is essential for determining whether a specific cause truly led to a particular effect. For instance, in evaluating the effectiveness of a new drug, counterfactual reasoning asks: "Would the patient have recovered without the drug?" This is difficult to answer directly, but statistical methods can help estimate the counterfactual outcome.
FAQ Section: Answering Common Questions About Casualty
-
What is the difference between correlation and causation? Correlation indicates a relationship between two variables, while causation implies that one variable directly influences the other. Correlation does not imply causation.
-
How can confounding variables be controlled for? Confounding variables can be controlled for through techniques such as randomization in experimental studies, statistical adjustment in observational studies, and careful study design.
-
What are some common biases in causal inference? Common biases include selection bias, confounding bias, and recall bias.
-
How can causal inference be used to make better decisions? By understanding causal relationships, we can make more informed decisions by targeting interventions at the root causes of problems rather than just treating symptoms.
Practical Tips: Maximizing the Benefits of Understanding Casualty
-
Start with a Clear Question: Begin by formulating a specific question about the causal relationship you are interested in.
-
Gather High-Quality Data: Ensure that the data you collect are accurate, reliable, and representative of the population of interest.
-
Choose Appropriate Methods: Select appropriate statistical methods for analyzing your data and drawing causal inferences.
-
Consider Potential Biases: Carefully consider potential biases in your data and analysis, and take steps to mitigate them.
-
Interpret Results Carefully: Avoid overinterpreting your results, and remember that correlation does not imply causation.
Final Conclusion: Wrapping Up with Lasting Insights
The concept of casualty, while seemingly straightforward, reveals a rich tapestry of philosophical and methodological considerations. Understanding casualty is not just an academic exercise; it's a crucial skill for navigating the complexities of the world around us. By employing rigorous methods, acknowledging limitations, and critically evaluating evidence, we can unlock the power of causal inference to improve decision-making, advance scientific knowledge, and build a more resilient future. The journey to understanding casualty is ongoing, and continued exploration of this crucial concept will undoubtedly lead to further insights and advancements across various disciplines.
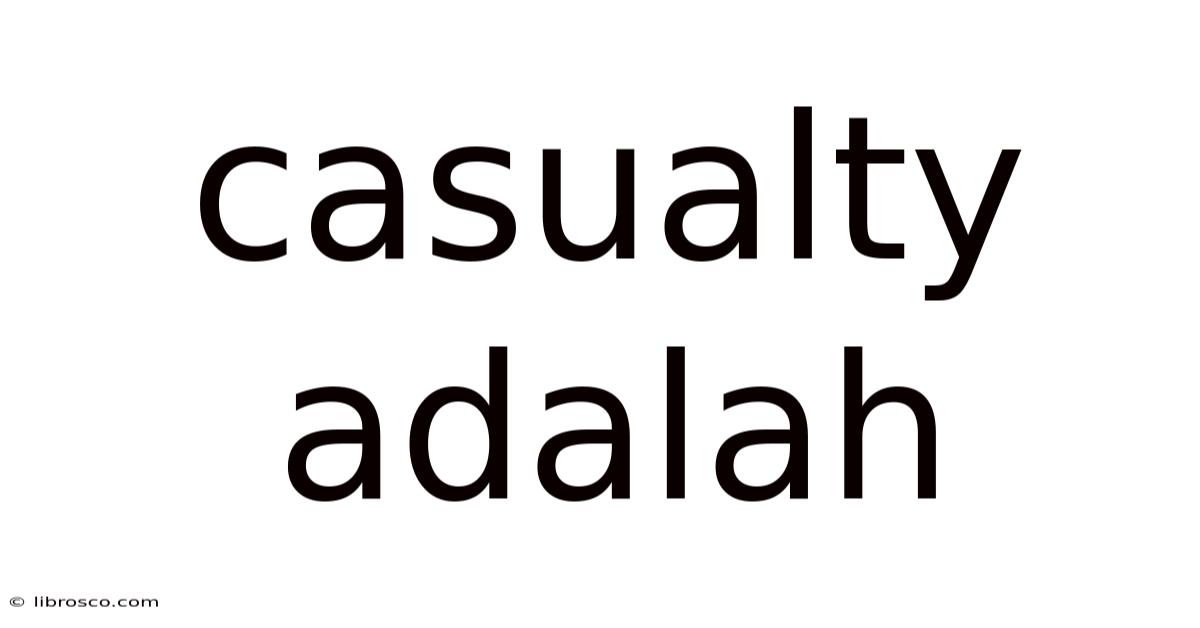
Thank you for visiting our website wich cover about Casualty Adalah. We hope the information provided has been useful to you. Feel free to contact us if you have any questions or need further assistance. See you next time and dont miss to bookmark.
Also read the following articles
Article Title | Date |
---|---|
How Much Is Augmentin Without Insurance At Walgreens | Apr 10, 2025 |
What Is A Credit Score Of 900 | Apr 10, 2025 |
How To Know Billing Cycle Of Credit Card Axis | Apr 10, 2025 |
Is A Fico Score Of 9 Bad | Apr 10, 2025 |
Billing Cycle Closing Date | Apr 10, 2025 |