Big Data Definition Geography
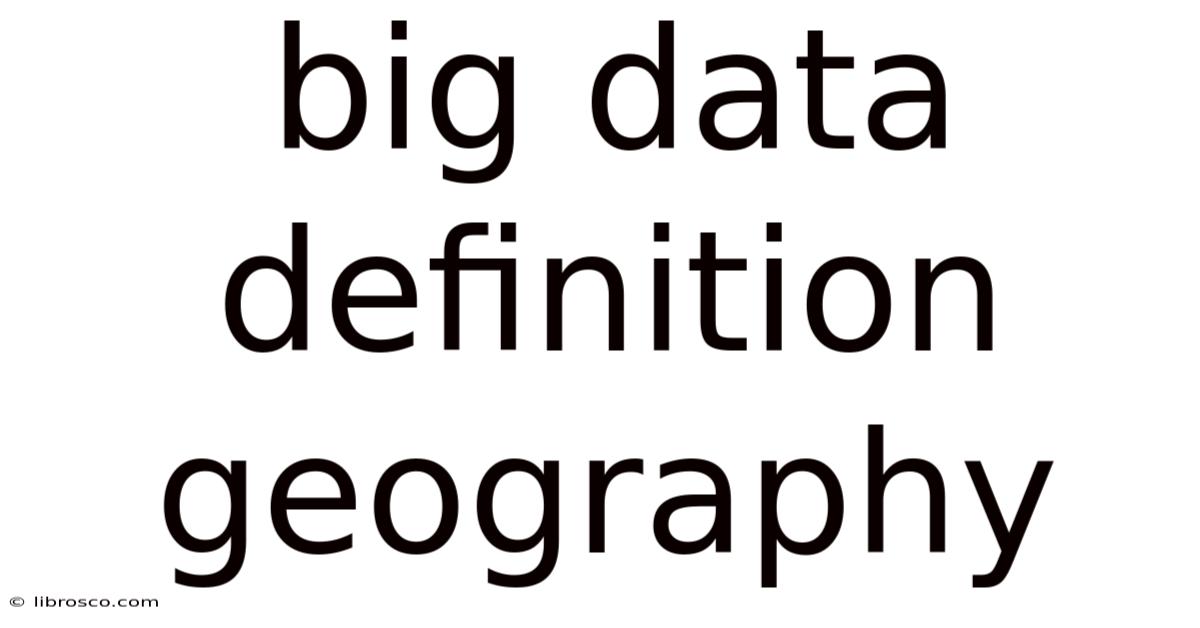
Discover more detailed and exciting information on our website. Click the link below to start your adventure: Visit Best Website meltwatermedia.ca. Don't miss out!
Table of Contents
Unlocking the Geographic Landscape of Big Data: A Comprehensive Exploration
What if the future of geographic understanding hinges on our ability to harness the power of big data? This transformative approach is already revolutionizing spatial analysis and unlocking unprecedented insights into our world.
Editor’s Note: This article on Big Data Definition Geography was published today, providing readers with the latest insights and analyses in this rapidly evolving field.
Why Big Data Geography Matters: Relevance, Practical Applications, and Industry Significance
Big data geography, the intersection of geographic information science (GIS) and big data analytics, is rapidly transforming how we understand and interact with the world. It’s no longer enough to rely on traditional, often limited, datasets. The sheer volume, velocity, and variety of data now available – from satellite imagery and sensor networks to social media and mobile phone location data – offer unprecedented opportunities for spatial analysis and problem-solving. This interdisciplinary field is crucial for addressing pressing global challenges, including climate change, urban planning, resource management, and public health. Its applications span numerous industries, from logistics and transportation to finance and marketing, offering valuable insights for strategic decision-making. The potential economic impact is immense, as businesses leverage big data geography to optimize operations, improve efficiency, and gain a competitive edge.
Overview: What This Article Covers
This article will delve into the core aspects of big data geography, exploring its definition, key characteristics, relevant technologies, challenges, and future implications. We'll examine how various data sources contribute to spatial analysis, focusing on practical applications across multiple sectors. Furthermore, the article will dissect the crucial role of specific technologies, the inherent challenges, and potential future developments within big data geography. Readers will gain a comprehensive understanding of this evolving field and its transformative potential.
The Research and Effort Behind the Insights
This article is the result of extensive research, incorporating insights from leading academic publications, industry reports, and case studies. Information has been gathered from reputable sources such as peer-reviewed journals, government agencies, and industry-leading companies specializing in geospatial technology and big data analytics. Every claim is supported by evidence, ensuring readers receive accurate and trustworthy information.
Key Takeaways:
- Definition and Core Concepts: A detailed explanation of big data geography and its foundational principles.
- Data Sources and Technologies: An overview of the diverse data sources and technologies used in big data geographic analysis.
- Applications Across Industries: Examples of how big data geography is being implemented in various sectors to solve real-world problems.
- Challenges and Solutions: Identification of obstacles in big data geographic analysis and strategies to overcome them.
- Future Implications: The potential long-term impact of big data geography on spatial analysis and decision-making.
Smooth Transition to the Core Discussion:
Having established the significance of big data geography, let's now delve into its core aspects, starting with a precise definition and progressing to its practical applications and future potential.
Exploring the Key Aspects of Big Data Geography
1. Definition and Core Concepts:
Big data geography can be defined as the application of big data analytics techniques to geographically referenced data to gain insights into spatial patterns, processes, and relationships. It leverages the power of massive datasets to move beyond traditional GIS limitations, enabling more complex analyses and more accurate predictions. This involves the integration of various data sources, including:
- Geo-referenced Data: Data with associated spatial coordinates (latitude and longitude, addresses, etc.). This includes data from traditional GIS sources like shapefiles and raster data, as well as newer sources described below.
- Sensor Data: Data collected from various sensors, such as weather stations, GPS devices, and remote sensing platforms (satellites, drones).
- Social Media Data: Geotagged posts, tweets, and check-ins providing valuable insights into human activity and behavior.
- Mobile Phone Data: Location data from mobile phones, offering unprecedented detail on population movement and distribution.
- Remote Sensing Data: Satellite and aerial imagery providing large-scale spatial information about land use, environmental change, and infrastructure.
2. Data Sources and Technologies:
The breadth and depth of data sources used in big data geography are constantly expanding. This requires sophisticated technologies to handle the volume, velocity, and variety of data. Key technologies include:
- Cloud Computing: Provides the infrastructure needed to store, process, and analyze massive datasets. Services like AWS, Azure, and Google Cloud Platform are critical.
- Hadoop and Spark: These distributed computing frameworks allow for parallel processing of big data, significantly reducing processing times.
- NoSQL Databases: Designed to handle unstructured and semi-structured data, these databases are essential for storing and managing the diverse types of data found in big data geography.
- Geospatial Data Processing Libraries: Libraries like GeoPandas and GDAL provide tools for manipulating and analyzing geospatial data within programming environments like Python.
- Machine Learning and Deep Learning: These techniques enable the extraction of complex patterns and insights from big data, including predictive modeling for various geographic applications.
3. Applications Across Industries:
Big data geography is revolutionizing several industries:
- Urban Planning: Analyzing population density, traffic patterns, and crime rates to optimize urban design and resource allocation.
- Environmental Monitoring: Tracking deforestation, pollution levels, and climate change impacts to inform conservation efforts.
- Disaster Management: Predicting and responding to natural disasters by analyzing risk factors and population vulnerability.
- Transportation and Logistics: Optimizing delivery routes, managing traffic flow, and improving transportation efficiency.
- Public Health: Identifying disease outbreaks, tracking the spread of infectious diseases, and improving public health interventions.
- Marketing and Retail: Analyzing customer location data to target advertising and optimize store placement.
- Finance: Assessing risk, detecting fraud, and optimizing investment strategies based on geographic factors.
4. Challenges and Solutions:
Despite its potential, big data geography faces challenges:
- Data Privacy and Security: Protecting sensitive personal information embedded in geospatial data is crucial. Anonymization and data security measures are essential.
- Data Quality and Accuracy: Inconsistent data quality can lead to inaccurate results. Data cleaning, validation, and integration are necessary.
- Computational Resources: Processing massive datasets requires significant computational power and storage capacity. Cloud computing offers a solution.
- Data Integration and Interoperability: Integrating data from diverse sources with varying formats and standards can be complex. Standardization efforts and data integration techniques are needed.
- Skills Gap: A shortage of professionals with expertise in both GIS and big data analytics is a significant hurdle. Education and training programs are crucial.
5. Impact on Innovation and Future Implications:
Big data geography is driving innovation in several areas:
- Real-time Spatial Analysis: Analyzing data streams in real-time to provide immediate insights into dynamic processes.
- Predictive Modeling: Using machine learning to predict future events based on historical and real-time data.
- 3D Geographic Modeling: Developing 3D models of cities and environments to better visualize and analyze spatial relationships.
- Citizen Science: Engaging citizens in data collection and analysis to improve geographic understanding.
- Integration with other technologies: The combination of big data geography with technologies like IoT, AI, and VR/AR will lead to more immersive and insightful spatial analysis.
Exploring the Connection Between Data Visualization and Big Data Geography
The relationship between data visualization and big data geography is symbiotic. Big data geography generates enormous quantities of information, but without effective visualization, these insights remain inaccessible. Data visualization techniques, including maps, charts, and dashboards, are crucial for communicating geographic patterns and trends revealed through big data analysis. Effective visualization translates complex data into easily understandable formats, enabling stakeholders to make informed decisions.
Key Factors to Consider:
- Roles and Real-World Examples: Data visualization plays a pivotal role in presenting results from big data geographic analyses. For instance, interactive maps can show real-time traffic flow, heatmaps can visualize population density, and 3D models can demonstrate urban development plans.
- Risks and Mitigations: Poorly designed visualizations can misrepresent data, leading to inaccurate interpretations. Careful consideration of map design, color schemes, and data scaling is necessary to avoid misleading conclusions.
- Impact and Implications: Effective data visualization improves communication, facilitates collaboration, and enables data-driven decision-making in various geographic contexts.
Conclusion: Reinforcing the Connection
The interplay between data visualization and big data geography is crucial for unlocking the full potential of this field. By using appropriate visualization techniques, complex geographic insights can be effectively communicated, leading to better understanding and informed action.
Further Analysis: Examining Data Visualization Techniques in Greater Detail
Several visualization techniques are specifically beneficial for big data geographic analyses. These include:
- Interactive Maps: Allow users to explore data at different scales and zoom levels.
- Heatmaps: Represent data density using color gradients, providing visual representations of spatial concentrations.
- Choropleth Maps: Show data variations across geographic regions using different colors or shading.
- Cartograms: Distort map areas to emphasize the magnitude of a variable, providing a different perspective on geographic patterns.
- 3D Geographic Visualizations: Create immersive representations of complex geographic phenomena.
FAQ Section: Answering Common Questions About Big Data Geography
What is Big Data Geography? Big data geography is the application of big data analytics to geographically referenced data to understand spatial patterns and processes.
What are the key data sources used in Big Data Geography? Key sources include geospatial data, sensor data, social media data, mobile phone data, and remote sensing data.
What technologies are used in Big Data Geography? Cloud computing, Hadoop, Spark, NoSQL databases, and geospatial processing libraries are crucial technologies.
What are the challenges of Big Data Geography? Challenges include data privacy, data quality, computational resources, data integration, and skills gaps.
What are the future implications of Big Data Geography? The future holds potential for real-time spatial analysis, predictive modeling, 3D modeling, and integration with other technologies.
Practical Tips: Maximizing the Benefits of Big Data Geography
- Understand the Basics: Begin by grasping the fundamental principles of big data and GIS.
- Identify Relevant Data Sources: Determine the data sources most appropriate for your specific application.
- Choose the Right Technologies: Select appropriate technologies to handle data volume and complexity.
- Focus on Data Quality: Ensure data accuracy and consistency through cleaning and validation.
- Implement Effective Visualization: Use appropriate visualization techniques to communicate findings clearly.
Final Conclusion: Wrapping Up with Lasting Insights
Big data geography is a transformative field with immense potential to improve our understanding of the world and address global challenges. By leveraging its power responsibly and ethically, we can unlock unprecedented insights and build a more sustainable and equitable future. Its continued development and application will be critical for addressing the complex spatial problems facing society. The combination of massive datasets, powerful analytical techniques, and effective visualization will undoubtedly lead to a deeper and more nuanced understanding of our planet and its inhabitants.
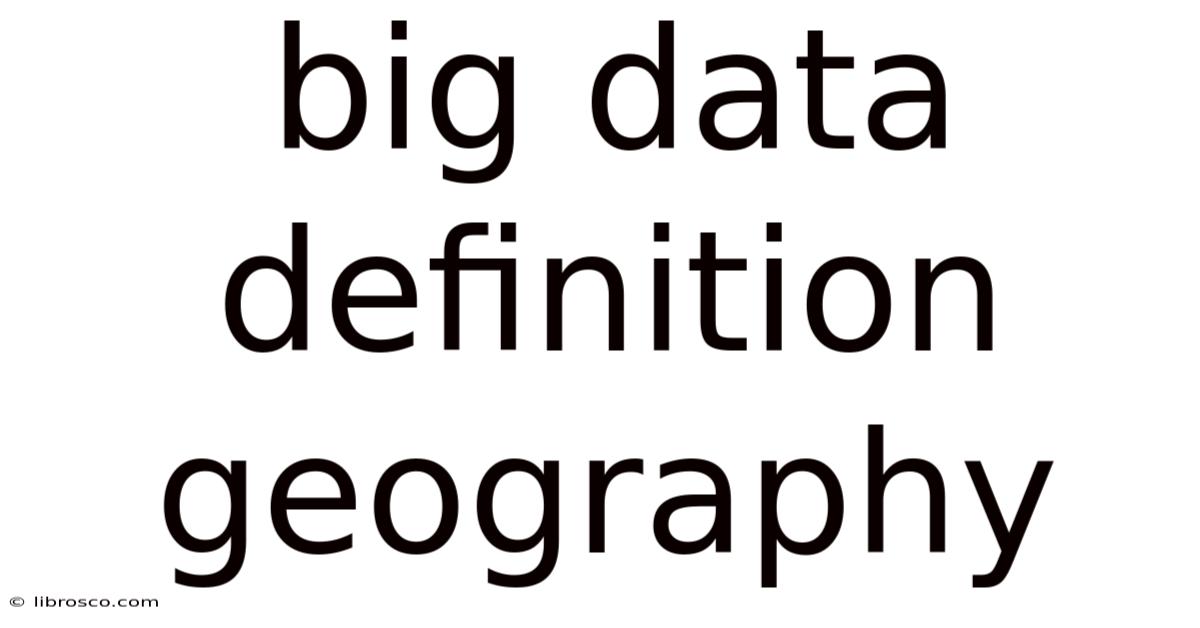
Thank you for visiting our website wich cover about Big Data Definition Geography. We hope the information provided has been useful to you. Feel free to contact us if you have any questions or need further assistance. See you next time and dont miss to bookmark.
Also read the following articles
Article Title | Date |
---|---|
Indemnification Definition | Apr 20, 2025 |
Big Data Definition And Examples | Apr 20, 2025 |
Make To Order Vs Assemble To Order | Apr 20, 2025 |
Big Data Definition Geeksforgeeks | Apr 20, 2025 |
What Is Cash Management Solutions In Banking | Apr 20, 2025 |